Quantitative Analysis of Chest MRI Images for Benign Malignant Diagnosis of Pulmonary Solid Nodules
Frontiers in oncology(2023)
摘要
Background In this study, we developed and validated machine learning (ML) models by combining radiomic features extracted from magnetic resonance imaging (MRI) with clinicopathological factors to assess pulmonary nodule classification for benign malignant diagnosis. Methods A total of 333 consecutive patients with pulmonary nodules (233 in the training cohort and 100 in the validation cohort) were enrolled. A total of 2,824 radiomic features were extracted from the MRI images (CE T1w and T2w). Logistic regression (LR), Naïve Bayes (NB), support vector machine (SVM), random forest (RF), and extreme gradient boosting (XGBoost) classifiers were used to build the predictive models, and a radiomics score (Rad-score) was obtained for each patient after applying the best prediction model. Clinical factors and Rad-scores were used jointly to build a nomogram model based on multivariate logistic regression analysis, and the diagnostic performance of the five prediction models was evaluated using the area under the receiver operating characteristic curve (AUC). Results A total of 161 women (48.35%) and 172 men (51.65%) with pulmonary nodules were enrolled. Six important features were selected from the 2,145 radiomic features extracted from CE T1w and T2w images. The XGBoost classifier model achieved the highest discrimination performance with AUCs of 0.901, 0.906, and 0.851 in the training, validation, and test cohorts, respectively. The nomogram model improved the performance with AUC values of 0.918, 0.912, and 0.877 in the training, validation, and test cohorts, respectively. Conclusion MRI radiomic ML models demonstrated good nodule classification performance with XGBoost, which was superior to that of the other four models. The nomogram model achieved higher performance with the addition of clinical information.
更多查看译文
关键词
magnetic resonance imaging,radiomics,machine learning,pulmonary nodules,differential diagnosis
AI 理解论文
溯源树
样例
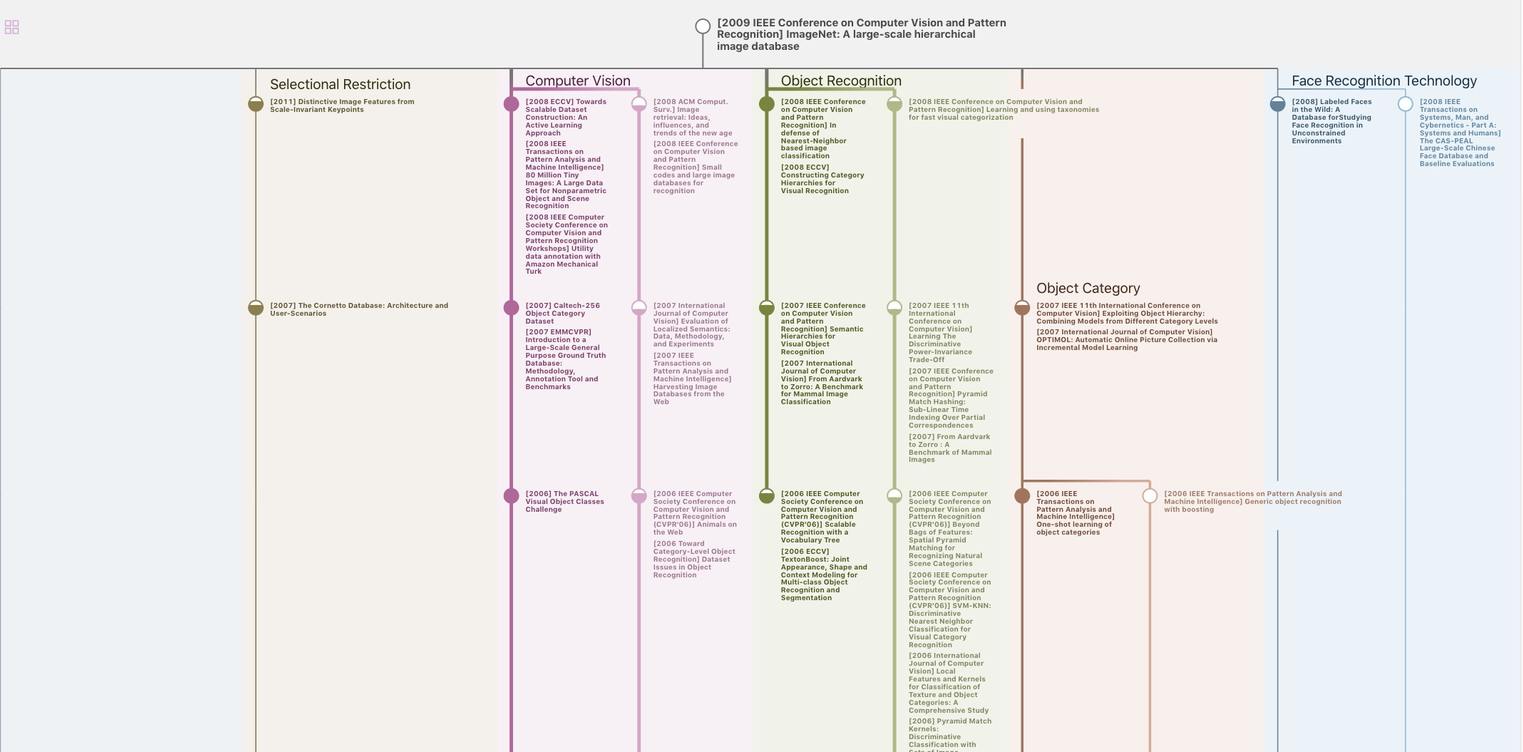
生成溯源树,研究论文发展脉络
Chat Paper
正在生成论文摘要