Development of a Prognostic Model for Personalized Prediction of Colon Adenocarcinoma (COAD) Patient Outcomes Using Methylation-Driven Genes
JOURNAL OF APPLIED GENETICS(2023)
关键词
Colon adenocarcinoma,Methylation-driven,Differentially expressed gene,Prognostic model,Personalization
AI 理解论文
溯源树
样例
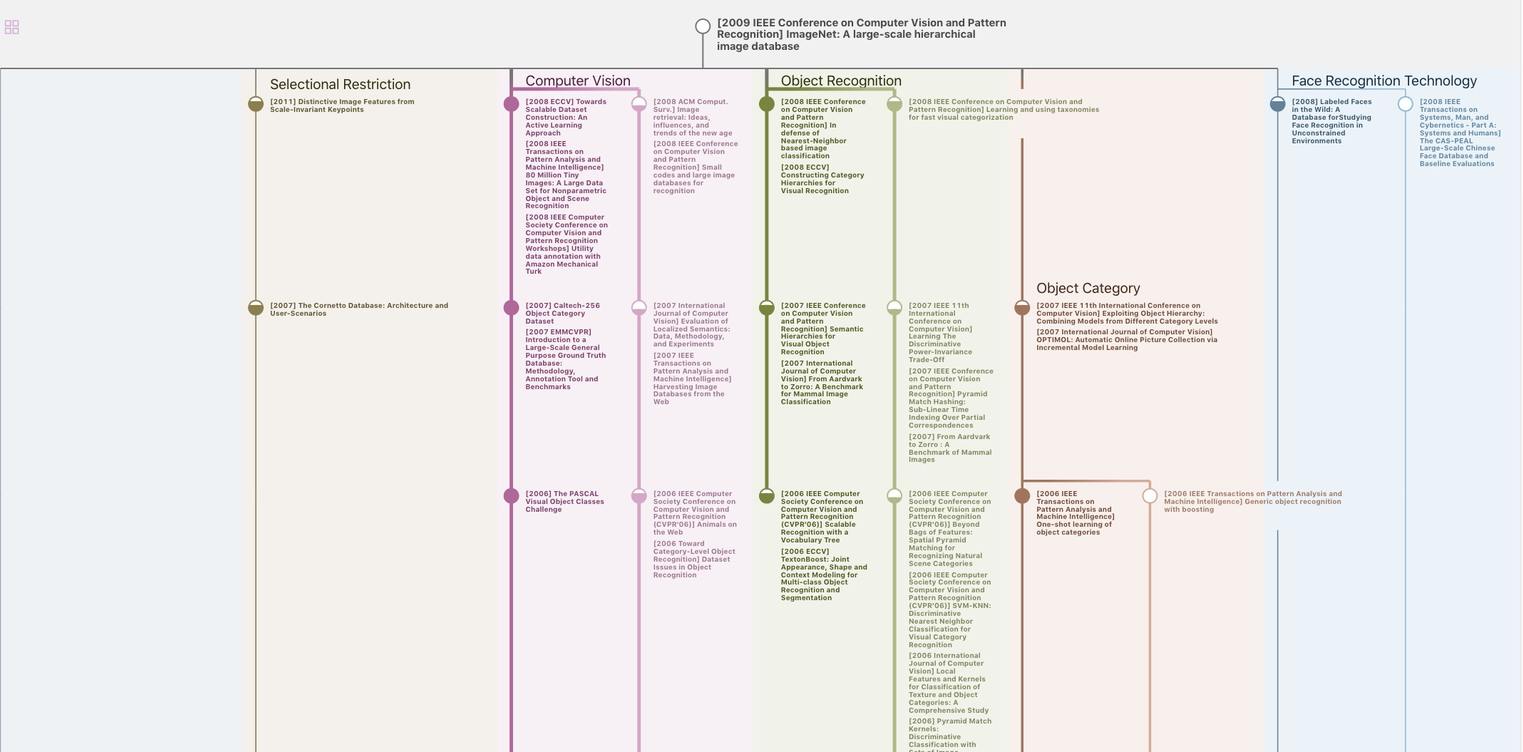
生成溯源树,研究论文发展脉络
Chat Paper
正在生成论文摘要