Prism: Revealing Hidden Functional Clusters from Massive Instances in Cloud Systems
2023 38TH IEEE/ACM INTERNATIONAL CONFERENCE ON AUTOMATED SOFTWARE ENGINEERING, ASE(2023)
摘要
Ensuring the reliability of cloud systems is critical for both cloud vendors and customers. Cloud systems often rely on virtualization techniques to create instances of hardware resources, such as virtual machines. However, virtualization hinders the observability of cloud systems, making it challenging to diagnose platform-level issues. To improve system observability, we propose to infer functional clusters of instances, i.e., groups of instances having similar functionalities. We first conduct a pilot study on a large-scale cloud system, i.e., Huawei Cloud, demonstrating that instances having similar functionalities share similar communication and resource usage patterns. Motivated by these findings, we formulate the identification of functional clusters as a clustering problem and propose a non-intrusive solution called Prism. Prism adopts a coarse-to-fine clustering strategy. It first partitions instances into coarse-grained chunks based on communication patterns. Within each chunk, Prism further groups instances with similar resource usage patterns to produce fine-grained functional clusters. Such a design reduces noises in the data and allows Prism to process massive instances efficiently. We evaluate Prism on two datasets collected from the real-world production environment of Huawei Cloud. Our experiments show that Prism achieves a v-measure of similar to 0.95, surpassing existing state-of-the-art solutions. Additionally, we illustrate the integration of Prism within monitoring systems for enhanced cloud reliability through two real-world use cases.
更多查看译文
关键词
functional clusters,cloud observability,instances,cloud systems,software reliability
AI 理解论文
溯源树
样例
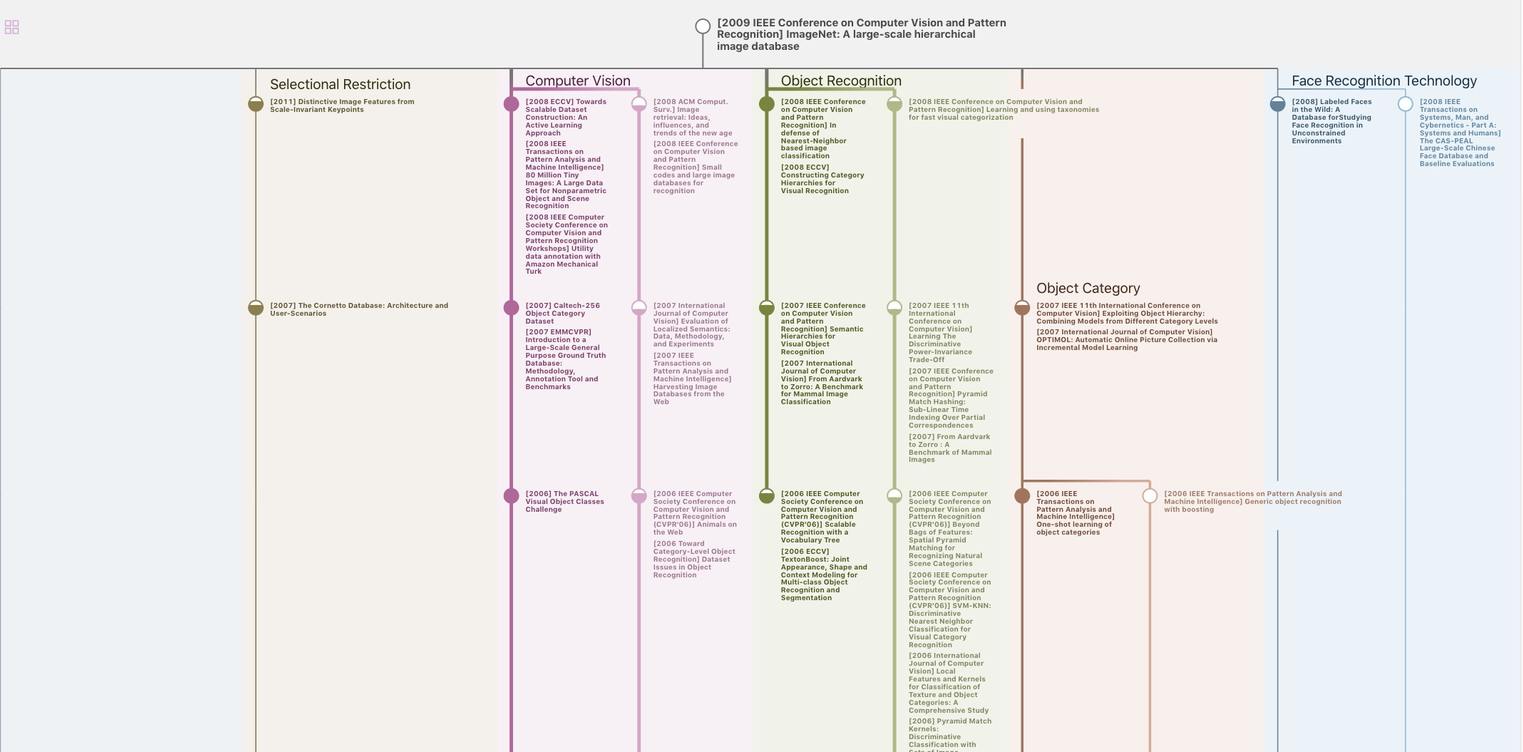
生成溯源树,研究论文发展脉络
Chat Paper
正在生成论文摘要