Synergistic effects of adaptive reward and reinforcement learning rules on cooperation
NEW JOURNAL OF PHYSICS(2023)
摘要
Cooperative behavior in multi-agent systems has been a focal point of research, particularly in the context of pairwise interaction games. While previous studies have successfully used reinforcement learning rules to explain and predict the behavior of agents in two-agent interactions, multi-agent interactions are more complex, and the impact of reward mechanisms on agent behavior is often overlooked. To address this gap, we propose a framework that combines the public goods game (PGG) with reinforcement learning and adaptive reward mechanisms to better capture decision-making behavior in multi-agent interactions. In that, PGG is adopted to reflect the decision-making behavior of multi-agent interactions, self-regarding Q-learning emphasizes an experience-based strategy update, and adaptive reward focuses on the adaptability. We are mainly concentrating on the synergistic effects of them. The simulations demonstrate that while self-regarding Q-learning fails to prevent the collapse of cooperation in the traditional PGG, the fraction of cooperation increases significantly when the adaptive reward strategy is included. Meanwhile, the theoretical analyses aligned with our simulation results, which revealed that there is a specific reward cost required to maximize the fraction of cooperation. Overall, this study provides a novel perspective on establishing cooperative reward mechanisms in social dilemmas and highlights the importance of considering adaptive reward mechanisms in multi-agent interactions.
更多查看译文
关键词
public goods game, adaptive reward, cooperation, self-regarding Q-learning
AI 理解论文
溯源树
样例
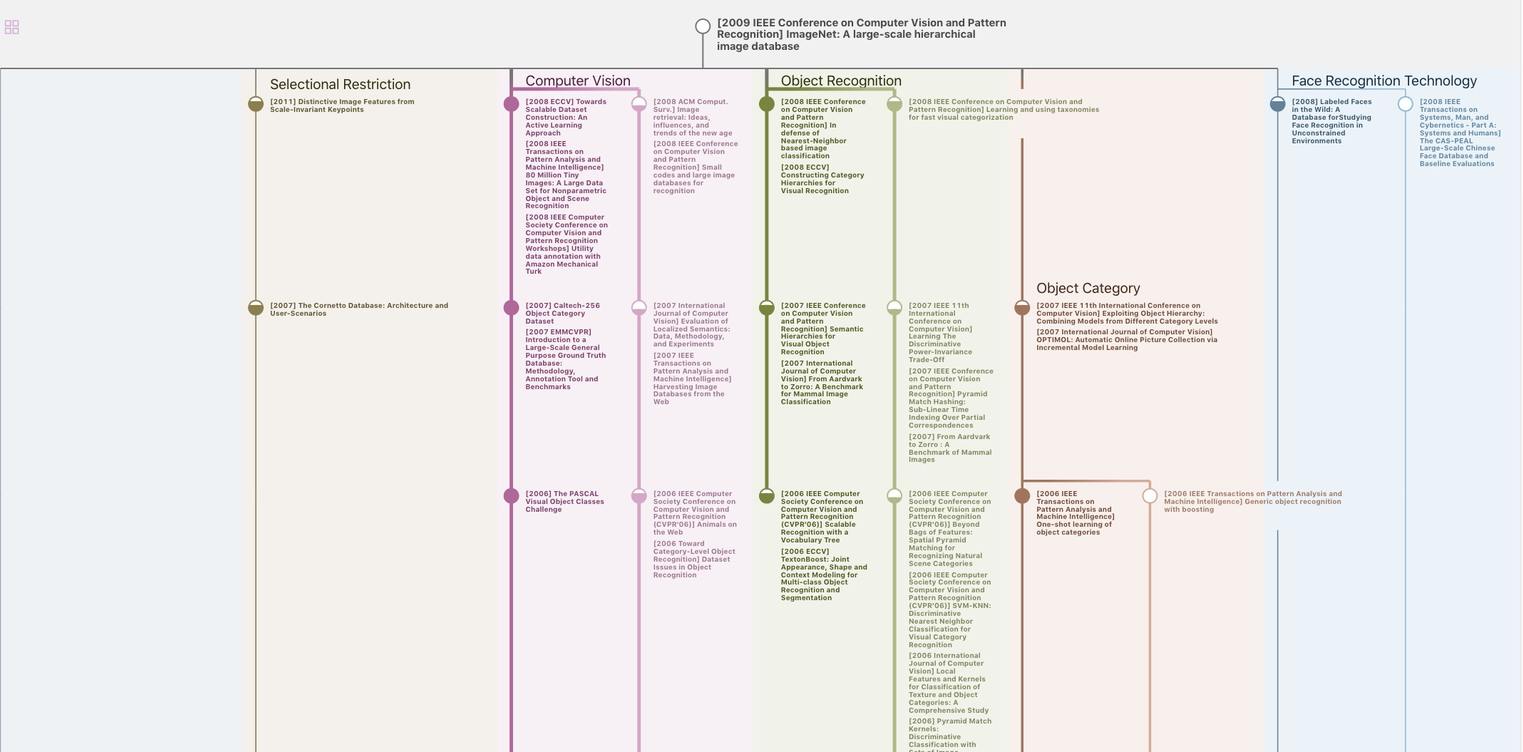
生成溯源树,研究论文发展脉络
Chat Paper
正在生成论文摘要