Imbalanced data generation and fusion for in-situ monitoring of laser powder bed fusion
MECHANICAL SYSTEMS AND SIGNAL PROCESSING(2023)
摘要
Laser powder bed fusion (LPBF) can produce near net shape products with complex geometries. Unfortunately, the LPBF technology still largely suffer from poor part consistency and process repeatability. This has driven significant research efforts combining process sensing and datadriven models to perform in-situ monitoring for ensuring part quality. However, the collected sensing datasets in actual applications may be imbalanced and making it challenging for in-situ monitoring. In this paper, we propose an imbalanced data generation and fusion approach to achieve in-situ quality monitoring with imbalanced datasets in the LPBF process. Layer-wise images of the solidified layer, acoustic and photodiode signals emitted during the printing process are first captured by the developed multi-sensor in-situ monitoring system. Thereafter, three imbalanced datasets of the collected sensing data are intentionally created. Then, a generative adversarial network-based data generation model is proposed to generate samples of the minority classes. A deep learning (DL)-based data fusion method is ultimately established to aggregate the three augmented balanced datasets. Results show that the generated high-quality samples can significantly improve the model performance, and the developed DL-based data fusion model outperforms the single-sensor-based quality classification models, providing a possible way for imbalanced quality monitoring in the LPBF process.
更多查看译文
关键词
Additive manufacturing,Laser powder bed fusion,Imbalanced quality monitoring,Data fusion,Deep learning
AI 理解论文
溯源树
样例
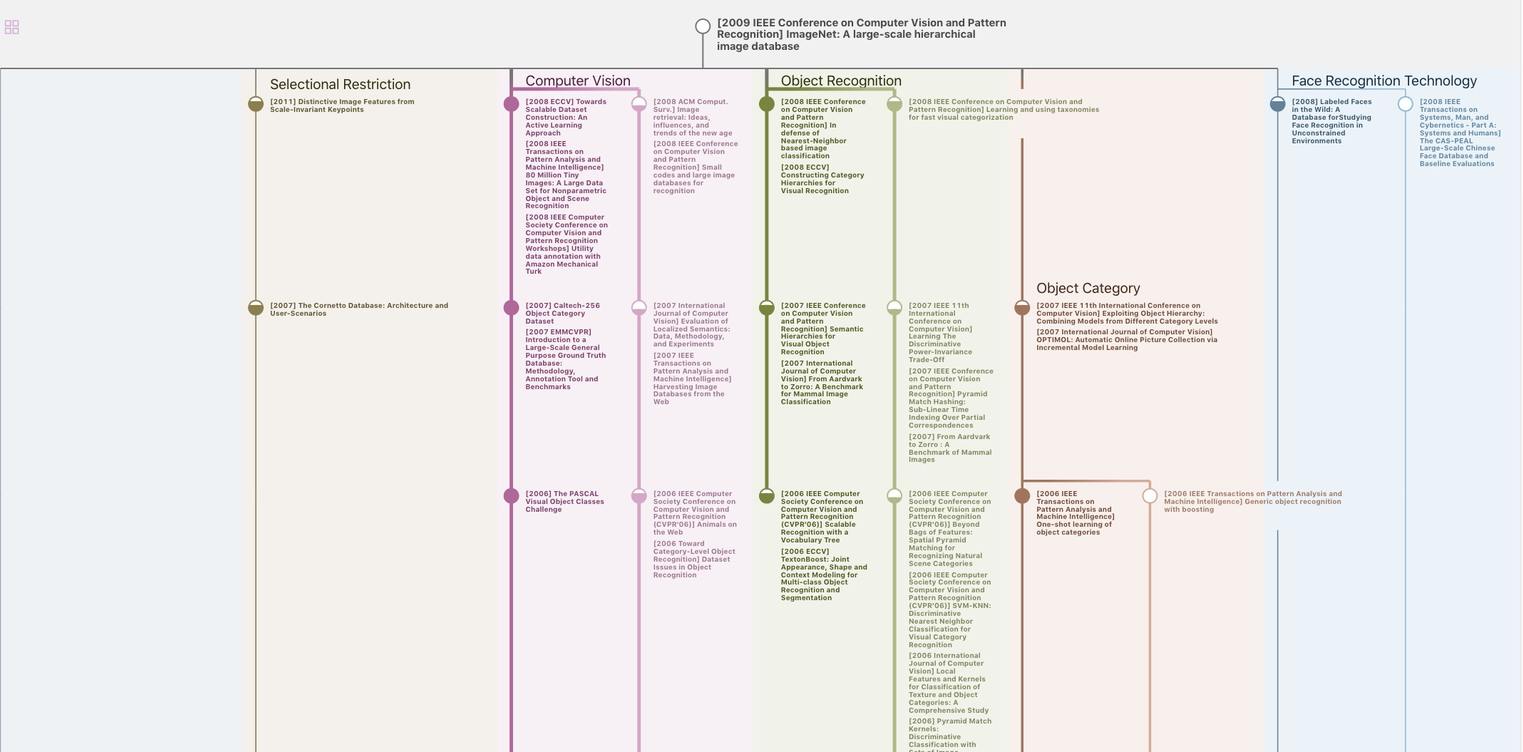
生成溯源树,研究论文发展脉络
Chat Paper
正在生成论文摘要