Multiple Object Tracking with Adaptive Multi-Features Fusion and Improved Learnable Graph Matching
VISUAL COMPUTER(2024)
摘要
Multiple object tracking is challenging due to the complex spatiotemporal relationship and the occlusion of different targets. Most existing methods use separate neural networks to generate robust features for data association inside the targets' bounding boxes. Unlike existing methods that just consider each target and the trajectory formed independently while ignoring the context information between tracklet and intra-frame detection, this paper proposes a tracking algorithm that combines multi-channel features with learnable graph matching. In brief, we use global and local salient features to model the appearance of intra-frame targets based on parallel graphs and mining high-order intra-context relationships using a completely undirected graph relationship between trajectories and detections. On this basis, we establish a multi-channel message communication mechanism through the maximum pooling mechanism to transmit the maximum dissimilation registration results to the graph matching layer, thereby forming a more robust allocation result in the data association process. Lastly, the evaluations on MOTChallenge benchmarks verify the effectiveness of the proposed method.
更多查看译文
关键词
Graph matching,Graph convolution,Object detection,Feature extraction,Target association,Multiple object tracking
AI 理解论文
溯源树
样例
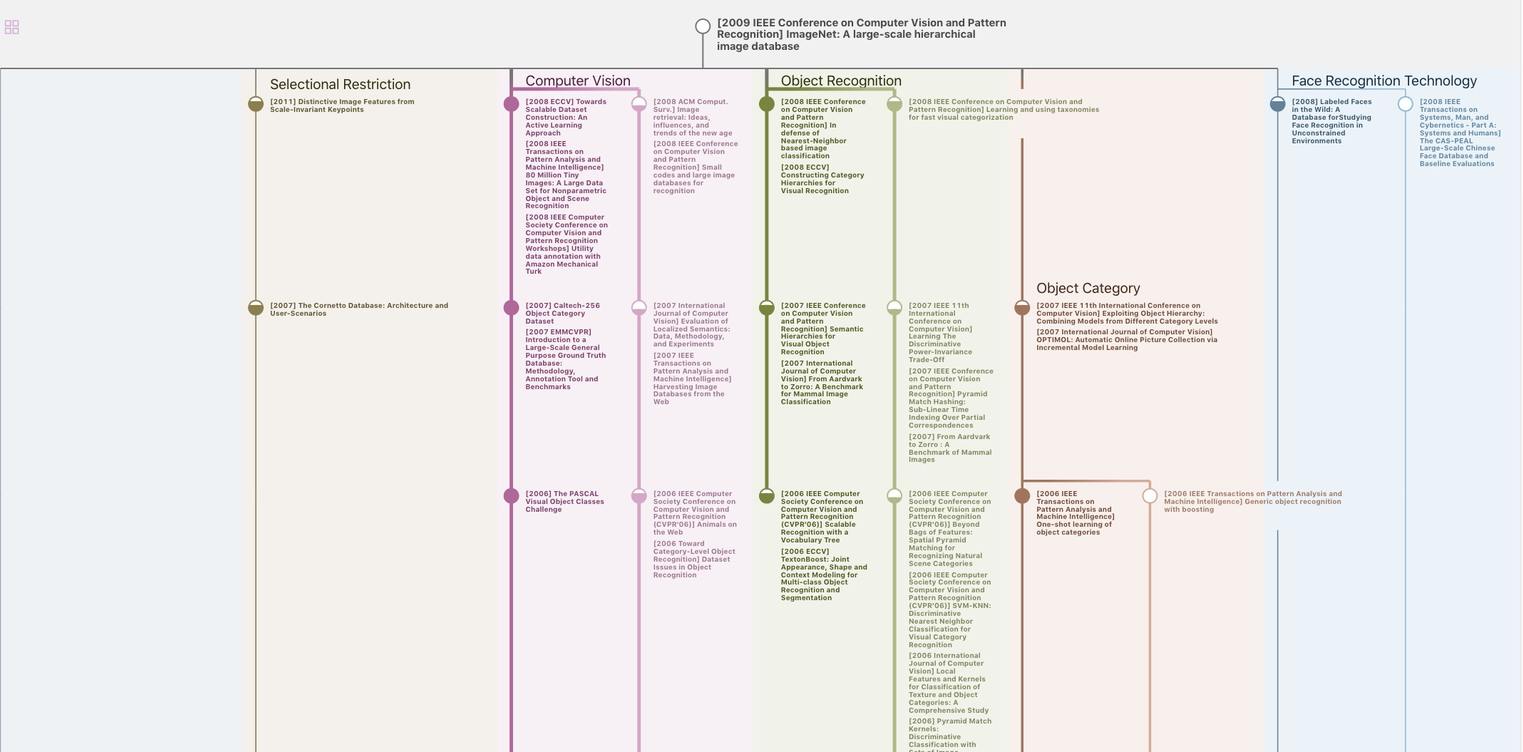
生成溯源树,研究论文发展脉络
Chat Paper
正在生成论文摘要