Adaptive Hyperparameter Optimization for Short Term Traffic Flow Prediction with Spatial Temporal Correlated Raster Data
EVOLVING SYSTEMS(2024)
摘要
IoT-based Intelligent transport system(ITS) is an innovative solution to urban traffic problems by providing different modes of traffic, traffic management and enabling the user to be informed and safe. ITS throughput is highly dependent on the effectiveness of short-term traffic flow prediction (STTP). To overcome this challenge, we propose the Adaptive grid search-based particle swarm optimization (GPSO) Long Short Term Memory (LSTM) model with spatial-temporal correlated raster data to predict short-term traffic flow. Initially, we explore non-euclidean space, uncertainty, and the nonlinearity of traffic flow. After that, we optimize hyperparameters of the LSTM according to real-time traffic flow data by Adaptive GPSO and incorporate spatial-temporal correlation value with LSTM to STTP. We evaluate the performance of the proposed model by doing a thorough experiment on two real-world data sets. To inspect the scalability of the proposed approach, we demonstrate it in different traffic conditions and for different congestion parameters. Simultaneously, we compare the performance of Adaptive GPSO-LSTM with existing state-of-art algorithms.
更多查看译文
关键词
Short-term traffic flow prediction,Spatial-temporal correlation,LSTM,Adaptive grid search based particle swarm optimization,Intelligent transport system
AI 理解论文
溯源树
样例
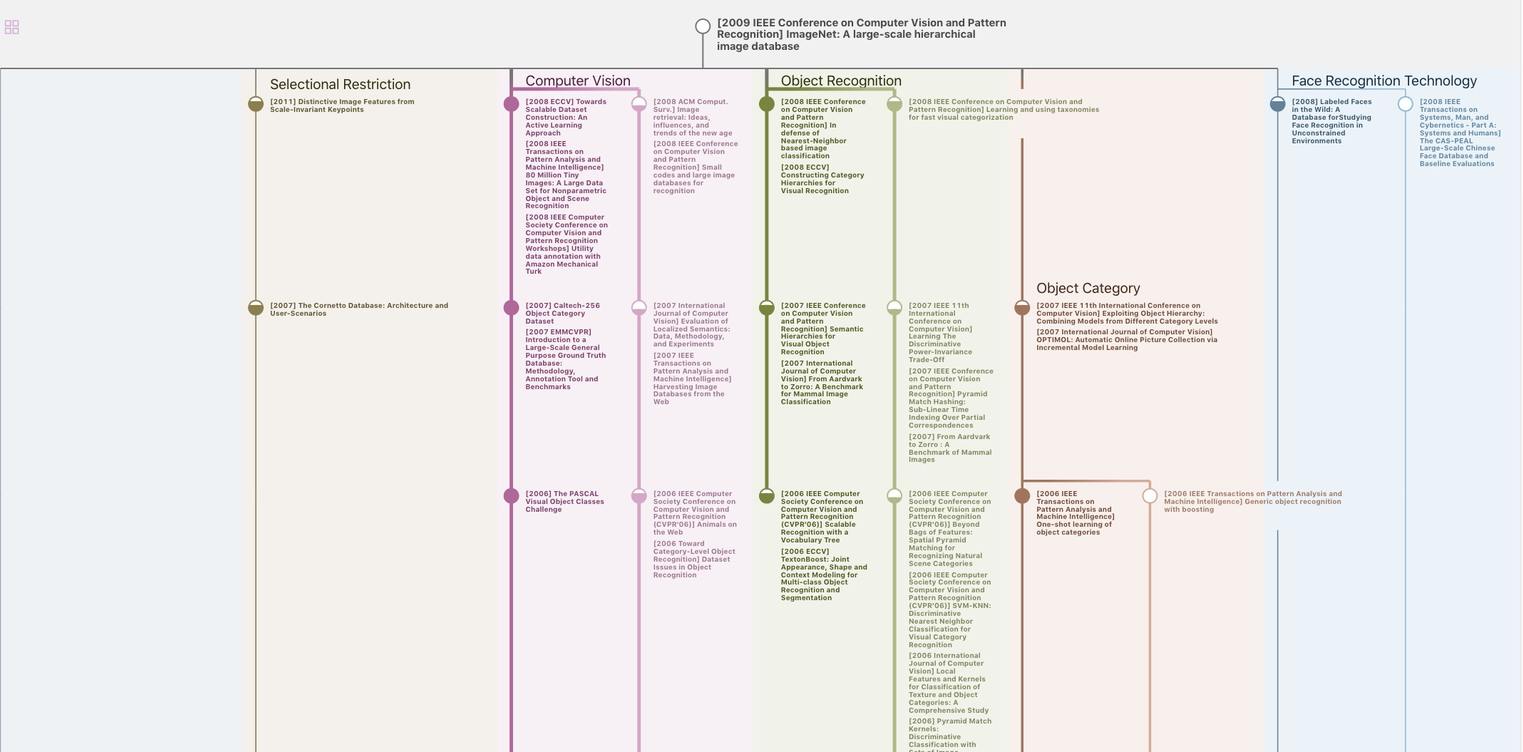
生成溯源树,研究论文发展脉络
Chat Paper
正在生成论文摘要