Development and Validation of Machine-Learning Models to Support Clinical Diagnosis for Non-Epileptic Psychogenic Seizures
Applied sciences(2023)
摘要
Electroencephalographic (EEG) signal processing and machine learning can support neurologists' work in discriminating Psychogenic Non-Epileptic Seizure (PNES) from epilepsy. PNES represents a neurological disease often misdiagnosed. Although the symptoms of PNES patients can be similar to those exhibited by epileptic patients, EEG signals during a psychogenic seizure do not show ictal patterns such as in epilepsy. Therefore, PNES diagnosis requires long-term EEG video. Applying signal processing and machine-learning methodologies could help clinicians find helpful information in the clinical diagnosis of PNES by analyzing EEG signals registered in resting conditions and in a short time. These methodologies should prevent long EEG recording sessions and avoid inducing seizures in the subjects. The aim of our study is to develop and validate several machine-learning models on a larger dataset, consisting of 225 EEGs (75 healthy, 75 PNES, and 75 subjects with epilepsy). A deep analysis of our results shows that changes in the evaluation strategy led to changes in accuracy from 45% to 83.98% for a standard Light Gradient Boosting Machine (LGBM) classifier. Our findings suggest that it is necessary to operate a very rigorous control in terms of experimental data collection (patient selection, signal acquisition) and terms of validation strategies to obtain and reproducible results.
更多查看译文
关键词
epilepsy,PNES,quantitative EEG,data mining,classification
AI 理解论文
溯源树
样例
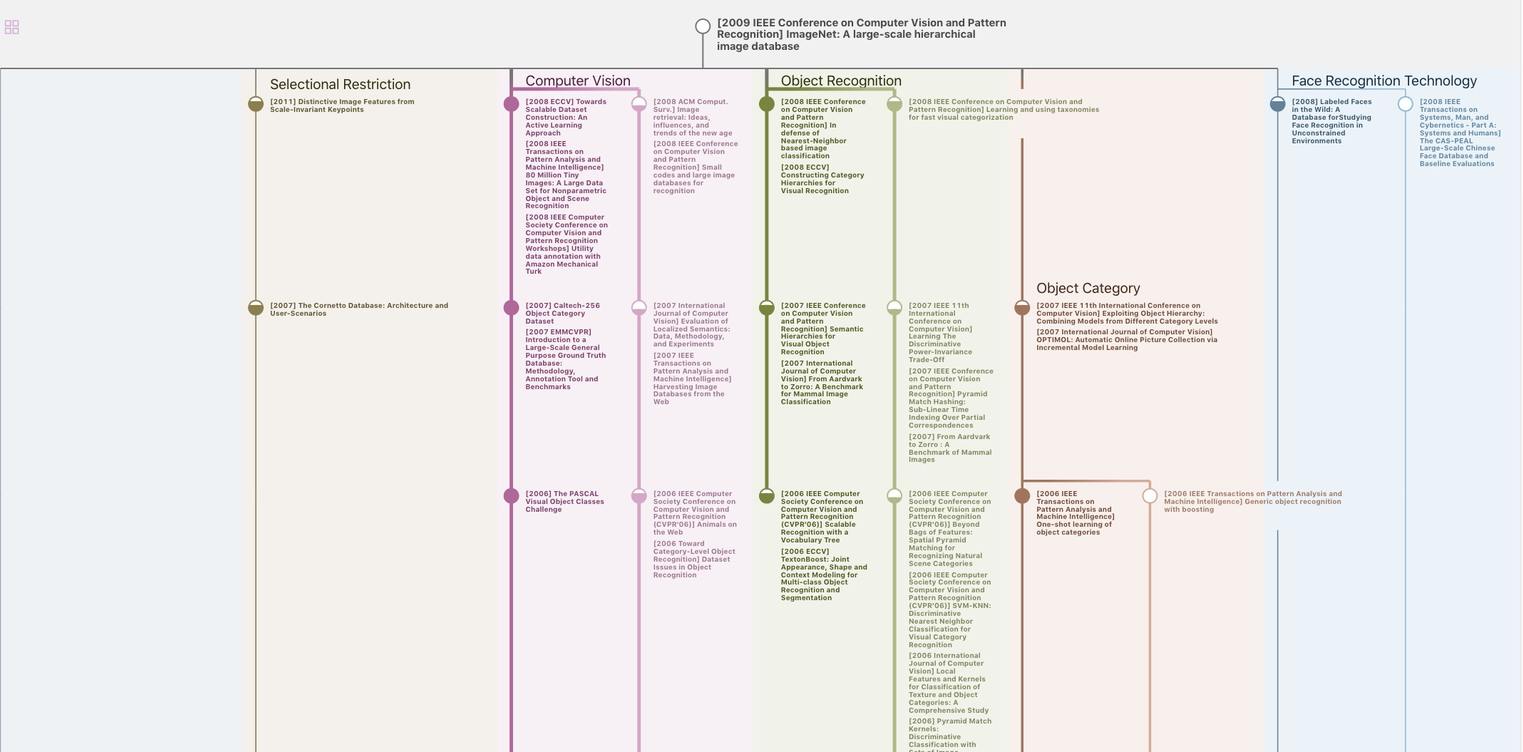
生成溯源树,研究论文发展脉络
Chat Paper
正在生成论文摘要