Adversarial Data Augmentation for HMM-Based Anomaly Detection
IEEE TRANSACTIONS ON PATTERN ANALYSIS AND MACHINE INTELLIGENCE(2023)
摘要
In this work, we concentrate on the detection of anomalous behaviors in systems operating in the physical world and for which it is usually not possible to have a complete set of all possible anomalies in advance. We present a data augmentation and retraining approach based on adversarial learning for improving anomaly detection. In particular, we first define a method for generating adversarial examples for anomaly detectors based on Hidden Markov Models (HMMs). Then, we present a data augmentation and retraining technique that uses these adversarial examples to improve anomaly detection performance. Finally, we evaluate our adversarial data augmentation and retraining approach on four datasets showing that it achieves a statistically significant performance improvement and enhances the robustness to adversarial attacks. Key differences from the state-of-the-art on adversarial data augmentation are the focus on multivariate time series (as opposed to images), the context of one-class classification (in contrast to standard multi-class classification), and the use of HMMs (in contrast to neural networks).
更多查看译文
关键词
Adversarial learning,anomaly detection,cyber-physical systems,data augmentation,HMMs,robotic systems
AI 理解论文
溯源树
样例
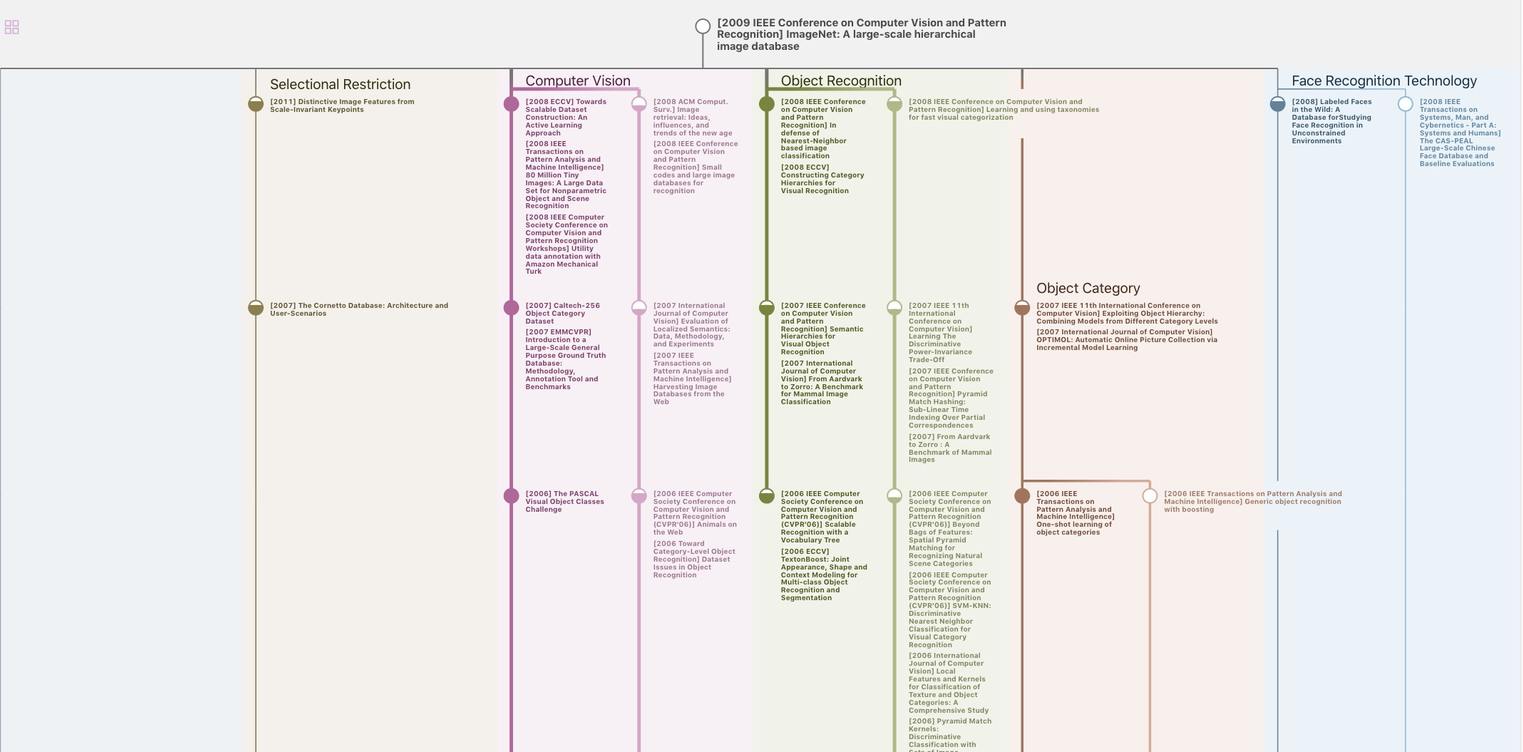
生成溯源树,研究论文发展脉络
Chat Paper
正在生成论文摘要