AgentBench: Evaluating LLMs as Agents
ICLR 2024(2023)
摘要
Large Language Models (LLMs) are becoming increasingly smart and autonomous, targeting real-world pragmatic missions beyond traditional NLP tasks. As a result, there has been an urgent need to evaluate LLMs as agents on challenging tasks in interactive environments. We present AgentBench, a multi-dimensional evolving benchmark that currently consists of 8 distinct environments to assess LLM-as-Agent's reasoning and decision-making abilities in a multi-turn open-ended generation setting. Our extensive test over 25 LLMs (including APIs and open-sourced models) shows that, while top commercial LLMs present a strong ability of acting as agents in complex environments, there is a significant disparity in performance between them and open-sourced competitors. It also serves as a component of an ongoing project with wider coverage and deeper consideration towards systematic LLM evaluation. Datasets, environments, and an integrated evaluation package for AgentBench are released at https://github.com/THUDM/AgentBench
更多查看译文
关键词
Large language models,Autonomous agents,Reasoning,Evaluation,Benchmark
AI 理解论文
溯源树
样例
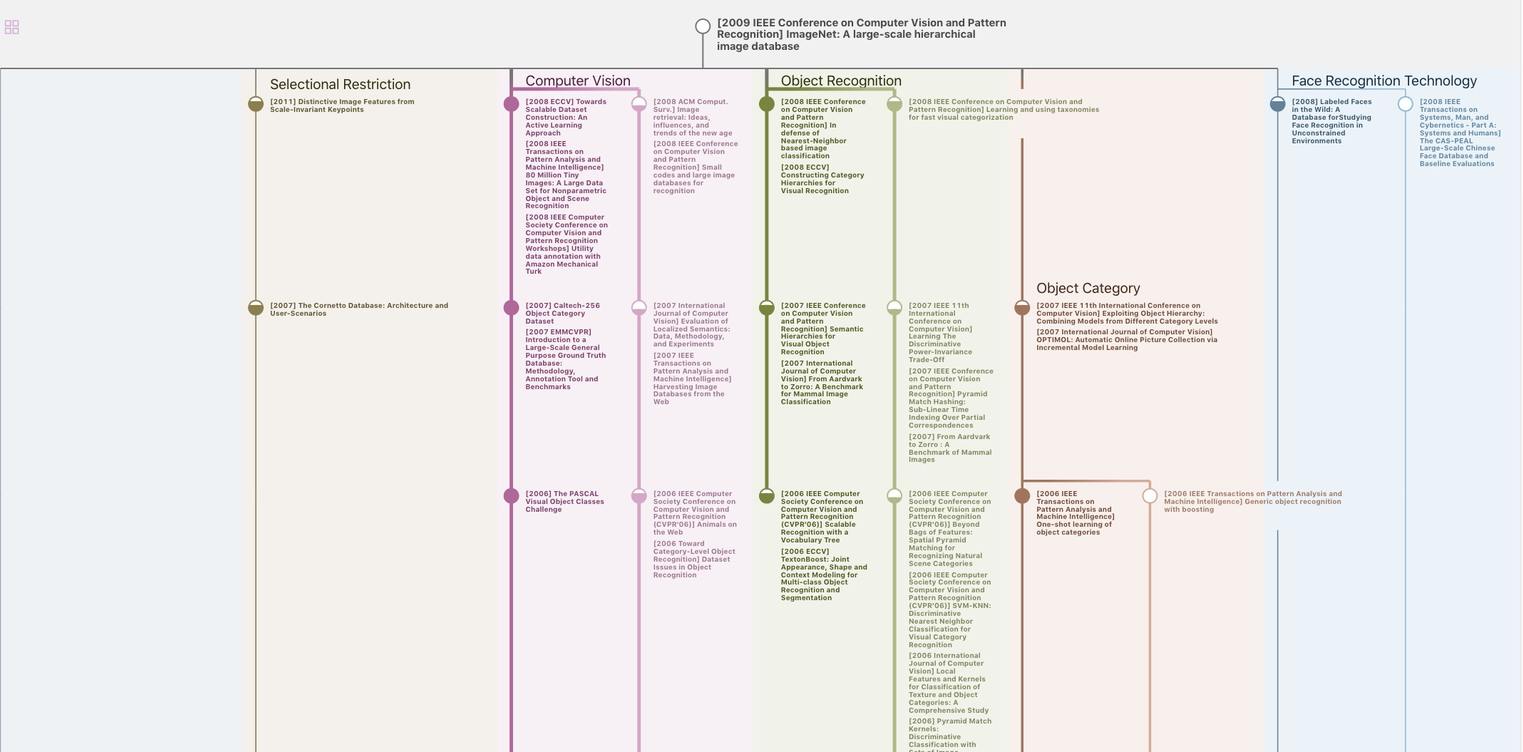
生成溯源树,研究论文发展脉络
Chat Paper
正在生成论文摘要