PromptSum: Parameter-Efficient Controllable Abstractive Summarization
CoRR(2023)
摘要
Prompt tuning (PT), a parameter-efficient technique that only tunes the additional prompt embeddings while keeping the backbone pre-trained language model (PLM) frozen, has shown promising results in language understanding tasks, especially in low-resource scenarios. However, effective prompt design methods suitable for generation tasks such as summarization are still lacking. At the same time, summarization guided through instructions (discrete prompts) can achieve a desirable double objective of high quality and controllability in summary generation. Towards a goal of strong summarization performance under the triple conditions of parameter-efficiency, data-efficiency, and controllability, we introduce PromptSum, a method combining PT with a multi-task objective and discrete entity prompts for abstractive summarization. Our model achieves competitive ROUGE results on popular abstractive summarization benchmarks coupled with a strong level of controllability through entities, all while only tuning several orders of magnitude less parameters.
更多查看译文
关键词
summarization,parameter-efficient
AI 理解论文
溯源树
样例
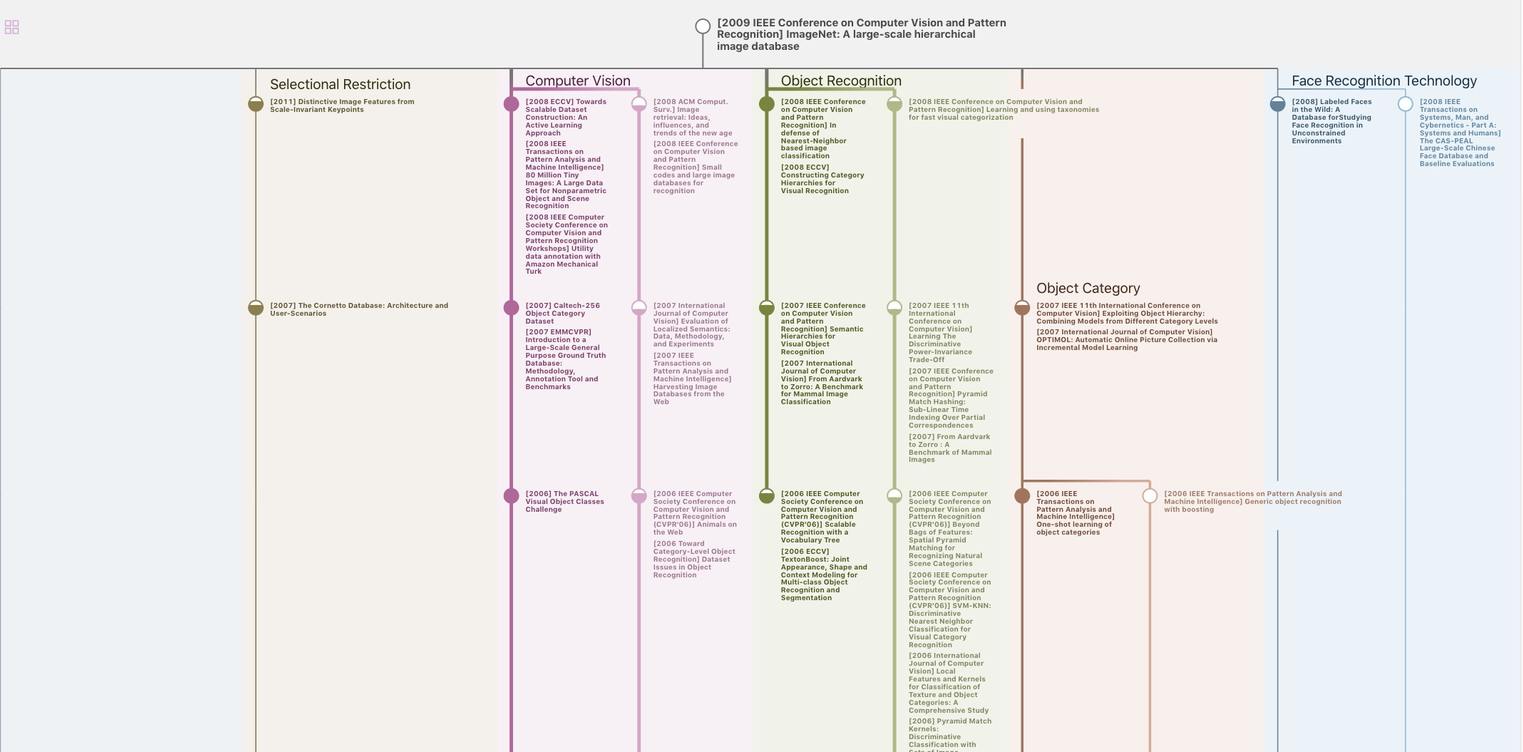
生成溯源树,研究论文发展脉络
Chat Paper
正在生成论文摘要