A Deep Neural Framework for Named Entity Recognition with Boosted Word Embeddings.
MULTIMEDIA TOOLS AND APPLICATIONS(2024)
摘要
The upcoming deep neural architectures overpower other previously proposed techniques for named entity recognition by involving fewer efforts but improving accuracy. In the present study, an important variant of recurrent neural network namely, a Bidirectional Long Short-Term Memory which is specially used for sequence classification problems has been used for the NER task. Word contextual features play an important role in the correct prediction of named entities. The present work contributes to developing novel word embeddings i.e. boosted word embeddings which can learn contextual features efficiently. Boosted word embeddings are the combination of character-based convolutional embeddings, part of speech embeddings, and word length embeddings. The results obtained using boosted word embeddings include f-score values of 73.99%, 66.94% and 77.95% respectively for Hindi, Punjabi and bilingual Hindi and Punjabi named entity recognition system. Boosted word embeddings are found effective in raising the accuracy of the named entity recognition model as compared to other models already present in the literature.
更多查看译文
关键词
Named entity recognition (NER),Deep neural network (DNN),Convolutional neural network (CNN),Bidirectional long short-term memory (bi-LSTM),Boosted word embeddings
AI 理解论文
溯源树
样例
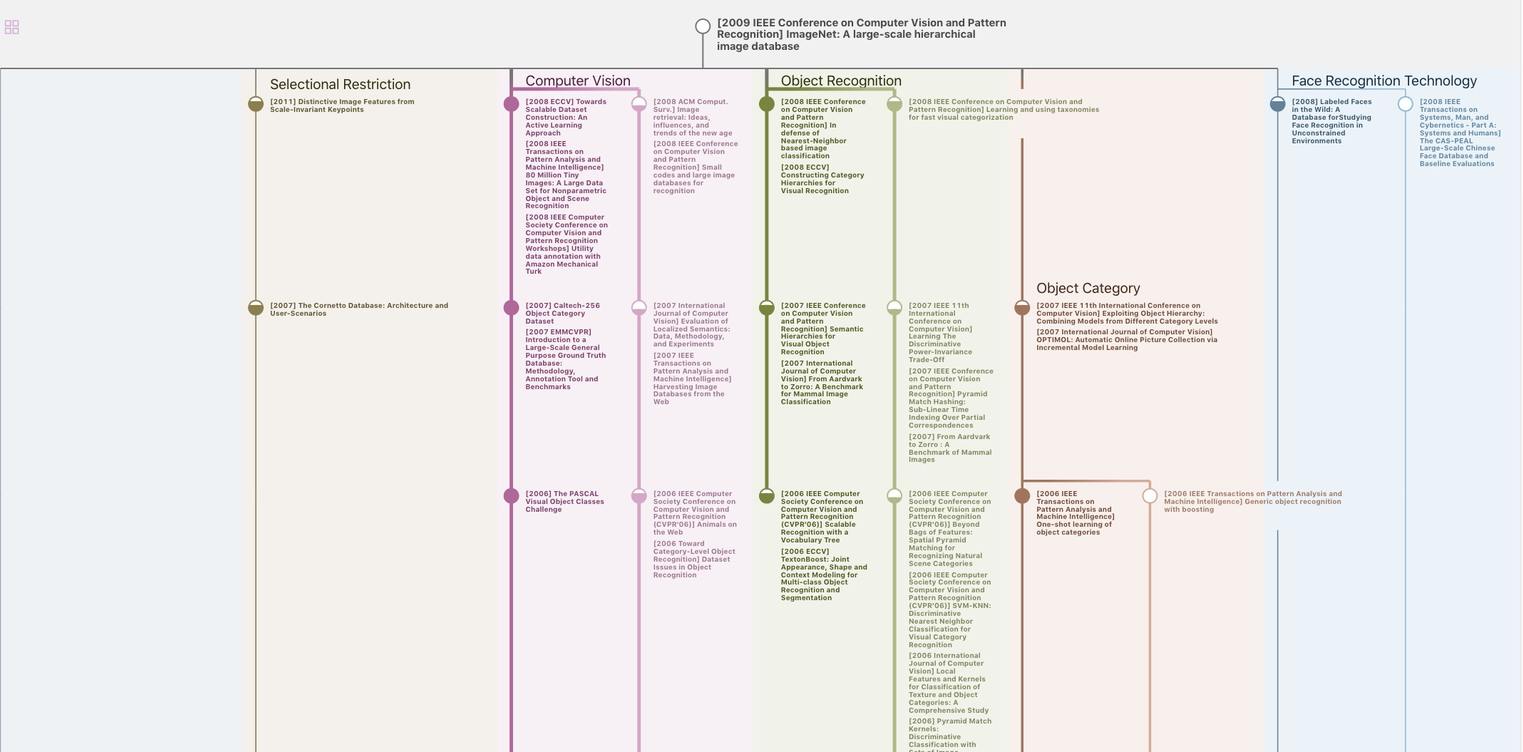
生成溯源树,研究论文发展脉络
Chat Paper
正在生成论文摘要