Adaptive multi-information distillation network for image dehazing
Multimedia Tools and Applications(2024)
Abstract
Image dehazing is a challenging low-level vision task that estimates potentially haze-free images from hazy images. In recent years, convolutional neural network-based methods have achieved impressive results in image dehazing. However, most existing image dehazing methods tend to train networks on large paired artificial datasets and directly generate a visually plausible result. These methods obtain high performance in homogeneous haze images, but often fail in real haze images, especially for haze images with large inhomogeneous areas. We find that the main possible reason is that these methods did not distinguish between haze areas of different densities when extracting haze features. To this end, in this paper, we present a highly generalized dehazing network that utilizes dense combinations of information distillation to obtain more effective and diversified features. We devise a multi-information distillation module to compensate for the limitations caused by inhomogeneous haze, which can adaptively assign weights to different haze regions according to the importance of the features. After 3 times of channel segmentation operations at a fixed ratio, the important features are gradually extracted while relatively unimportant features will be retained. To further improve the low-level features that are easily lost during the training process, we design an enhanced spatial-channel attention module to guide the network to focus on the recovery of detailed information. Benefiting from the property of this network, we can more easily recover different types of haze images. Extensive experimental results demonstrate that our method surpasses most state-of-the-art dehazing methods in terms of visual quality, quantitative index, and various datasets.
MoreTranslated text
Key words
Image dehazing,Attention mechanism,Information distillation,Deep learning
AI Read Science
Must-Reading Tree
Example
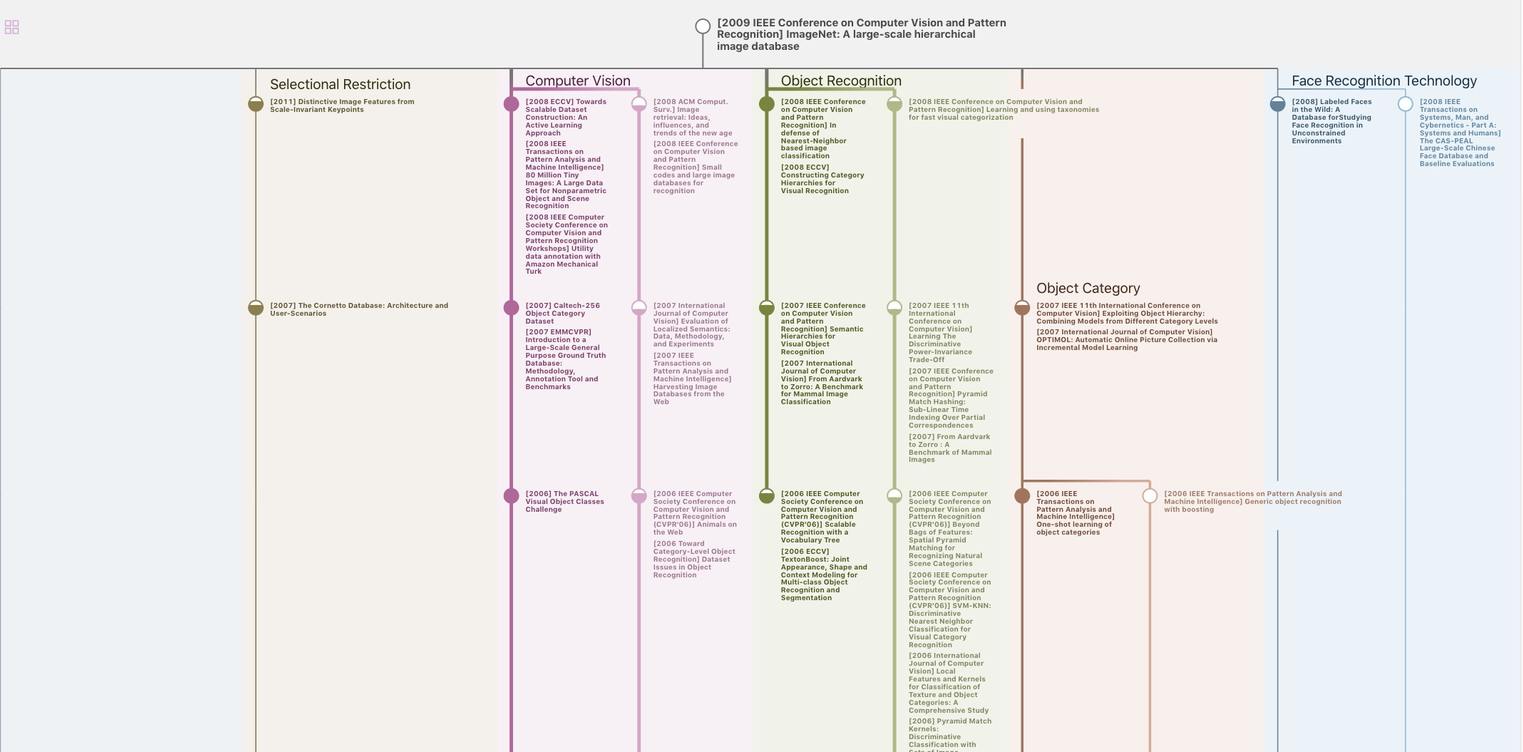
Generate MRT to find the research sequence of this paper
Chat Paper
Summary is being generated by the instructions you defined