Fault Diagnosis of Rolling Bearing under Limited Samples Using Joint Learning Network Based on Local-Global Feature Perception
Journal of mechanical science and technology(2023)
摘要
Deep learning is widely used in the field of rolling bearing fault diagnosis because of its excellent advantages in data analysis. However, in practical industrial scenarios, the capability of intelligent fault diagnosis (IFD) method is still affected by two problems: (1) The signal samples provided for network learning are limited; (2) Fully extracting feature information from the original data is difficult. To address the above issues, a novel fault diagnosis method using joint learning network (JLNet) based on local-global feature perception is proposed. The method enhances the learning mechanism of fault signal through the local information dynamic perception subnetwork, which dynamically distinguishes between local impulse segment and normal signal segment. Then, a global channel attention mechanism (CAM) is used to guide the assignment of weights, which helps bidirectional gated recurrent unit (BiGRU) to learn advanced discriminative features. The feature information of the original signal is thoroughly mined through local-global comprehensive perception, thus realizing efficient diagnosis. In addition, the variation of the characteristics of each layer is analyzed by visualization, which improves the interpretability of the network. Finally, experiments are conducted using two different datasets, and the results show that JLNet has a better diagnostic effects and robustness.
更多查看译文
关键词
Fault diagnosis,Dynamic perception,Joint learning network,Global channel attention,Limited samples
AI 理解论文
溯源树
样例
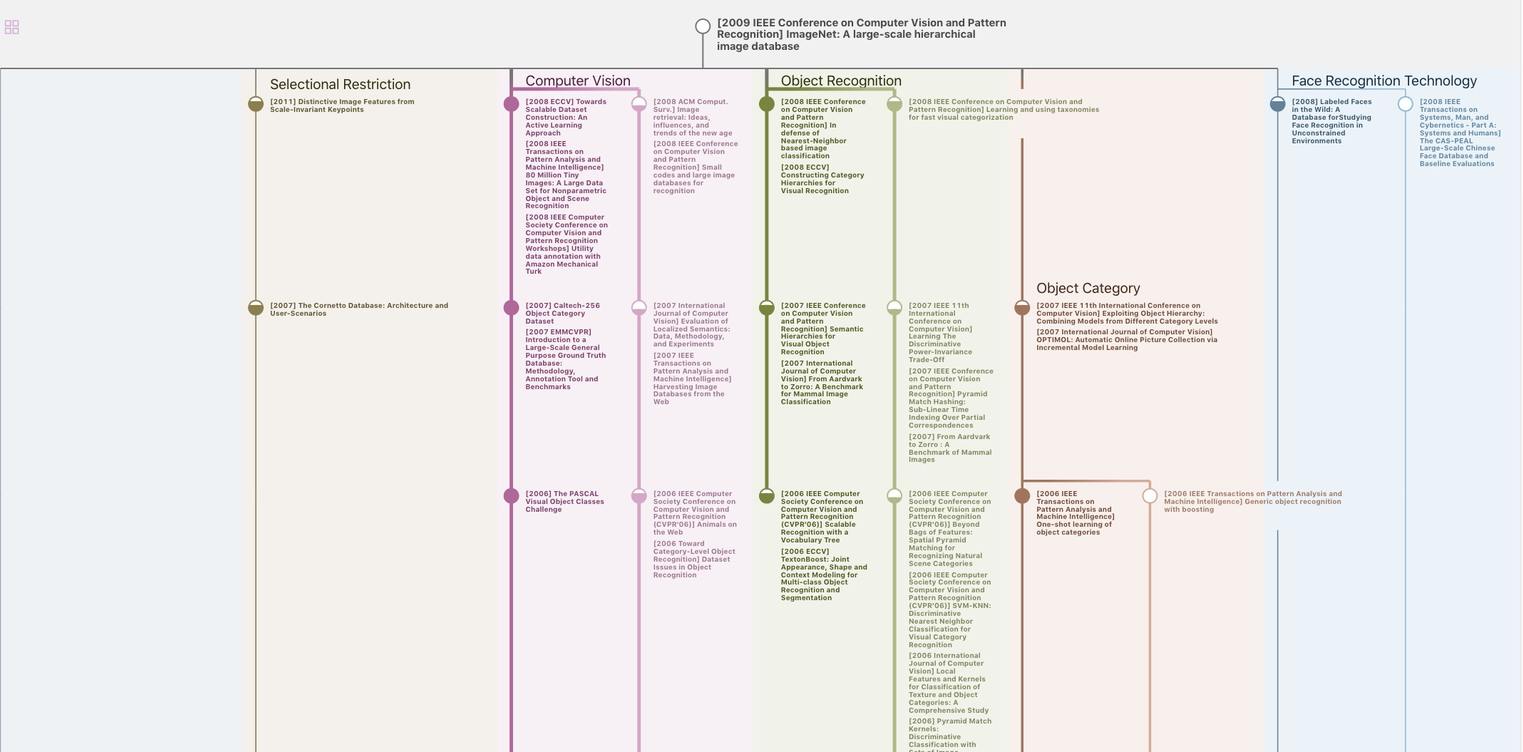
生成溯源树,研究论文发展脉络
Chat Paper
正在生成论文摘要