COCA: COllaborative CAusal Regularization for Audio-Visual Question Answering.
AAAI(2023)
摘要
Audio-Visual Question Answering (AVQA) is a sophisticated QA task, which aims at answering textual questions over given video-audio pairs with comprehensive multimodal reasoning. Through detailed causal-graph analyses and careful inspections of their learning processes, we reveal that AVQA models are not only prone to over-exploit prevalent language bias, but also suffer from additional joint-modal biases caused by the shortcut relations between textual-auditory/visual cooccurrences and dominated answers. In this paper, we propose a COllabrative CAusal (COCA) Regularization to remedy this more challenging issue of data biases. Specifically, a novel Bias-centered Causal Regularization (BCR) is proposed to alleviate specific shortcut biases by intervening biasirrelevant causal effects, and further introspect the predictions of AVQA models in counterfactual and factual scenarios. Based on the fact that the dominated bias impairing model robustness for different samples tends to be different, we introduce a Multi-shortcut Collaborative Debiasing (MCD) to measure how each sample suffers from different biases, and dynamically adjust their debiasing concentration to different shortcut correlations. Extensive experiments demonstrate the effectiveness as well as backbone-agnostic ability of our COCA strategy, and it achieves state-of-the-art performance on the large-scale MUSIC-AVQA dataset.
更多查看译文
关键词
collaborative causal regularization,audio-visual
AI 理解论文
溯源树
样例
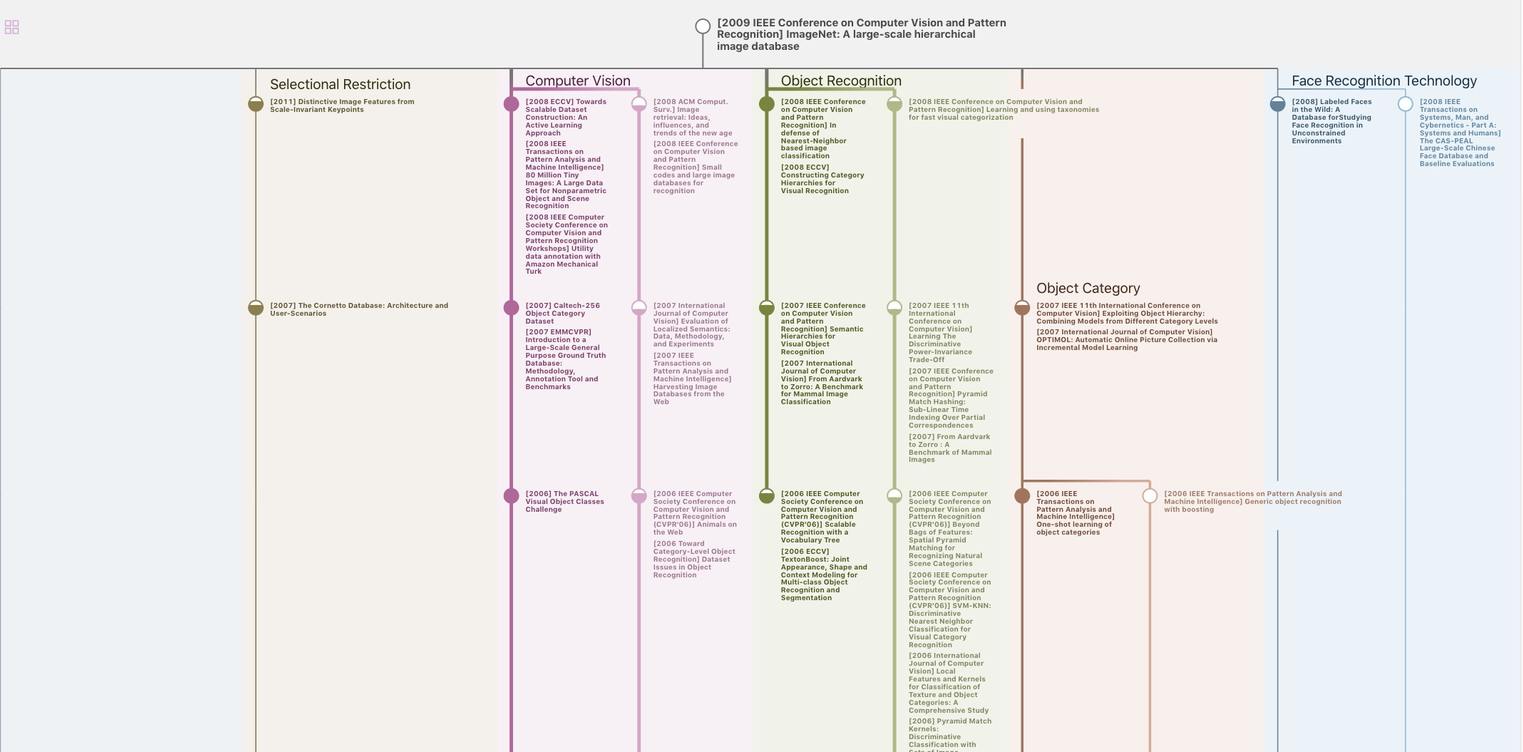
生成溯源树,研究论文发展脉络
Chat Paper
正在生成论文摘要