Exploring Model Structures to Reduce Data Requirements for Neural ODE Learning in Control Systems.
Journal of advanced computational intelligence and intelligent informatics(2023)
摘要
In this study, we investigate model structures for neural ODEs to improve the data efficiency in learning the dynamics of control systems. We introduce two model structures and compare them with a typical baseline structure. The first structure considers the relationship between the coordinates and velocities of the control system, while the second structure adds linearity with respect to the control term to the first structure. Both of these structures can be easily implemented without requiring additional computation. In numerical experiments, we evaluate these structure on simulated simple pendulum and CartPole systems and show that incorporating these characteristics into the model structure leads to accurate learning with a smaller amount of training data compared to the baseline structure.
更多查看译文
关键词
system identification,neural ODE
AI 理解论文
溯源树
样例
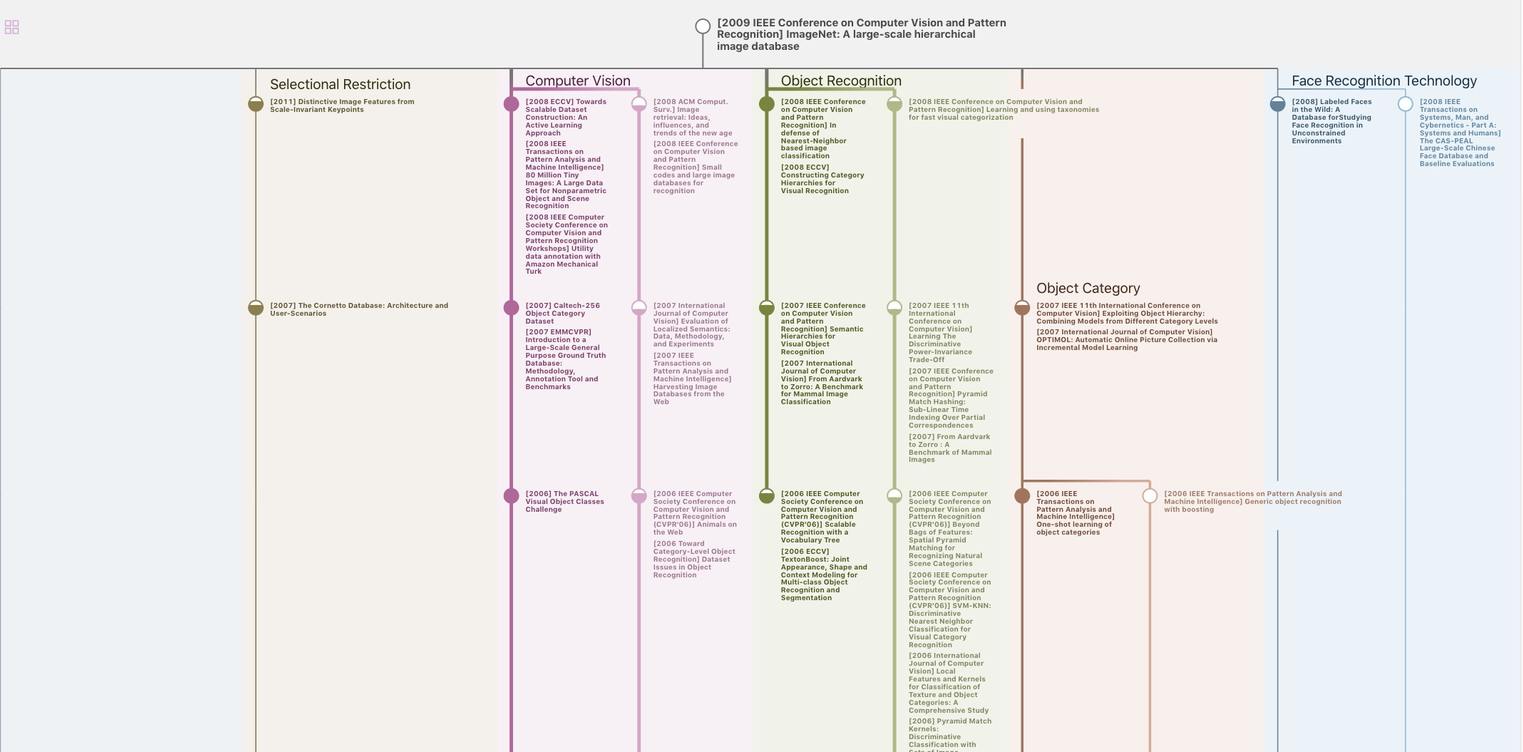
生成溯源树,研究论文发展脉络
Chat Paper
正在生成论文摘要