Deep-learning-enabled protein–protein interaction analysis for prediction of SARS-CoV-2 infectivity and variant evolution
Nature medicine(2023)
Key words
Protein function predictions,Viral infection,Biomedicine,general,Cancer Research,Metabolic Diseases,Infectious Diseases,Molecular Medicine,Neurosciences
AI Read Science
Must-Reading Tree
Example
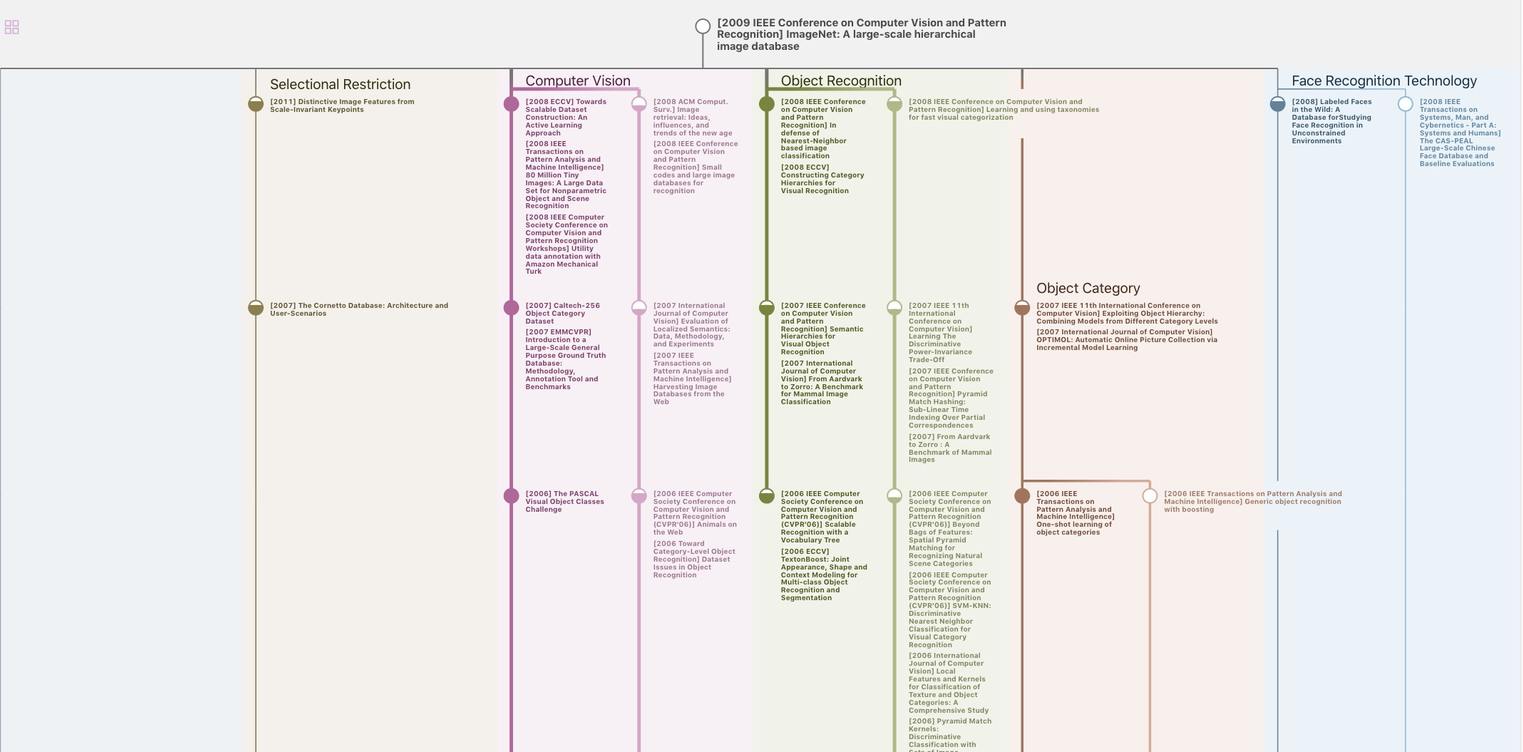
Generate MRT to find the research sequence of this paper
Chat Paper
Summary is being generated by the instructions you defined