Cross-Modal Label Contrastive Learning for Unsupervised Audio-Visual Event Localization.
AAAI(2023)
摘要
This paper for the first time explores audio-visual event localization in an unsupervised manner. Previous methods tackle this problem in a supervised setting and require segment-level or video-level event category ground-truth to train the model. However, building large-scale multi-modality datasets with category annotations is human-intensive and thus not scalable to real-world applications. To this end, we propose cross-modal label contrastive learning to exploit multi-modal information among unlabeled audio and visual streams as self-supervision signals. At the feature representation level, multimodal representations are collaboratively learned from audio and visual components by using self-supervised representation learning. At the label level, we propose a novel self-supervised pretext task i.e. label contrasting to self-annotate videos with pseudo-labels for localization model training. Note that irrelevant background would hinder the acquisition of high-quality pseudo-labels and thus lead to an inferior localization model. To address this issue, we then propose an expectation-maximization algorithm that optimizes the pseudo-label acquisition and localization model in a coarse-to-fine manner. Extensive experiments demonstrate that our unsupervised approach performs reasonably well compared to the state-of-the-art supervised methods.
更多查看译文
关键词
localization,event,cross-modal,audio-visual
AI 理解论文
溯源树
样例
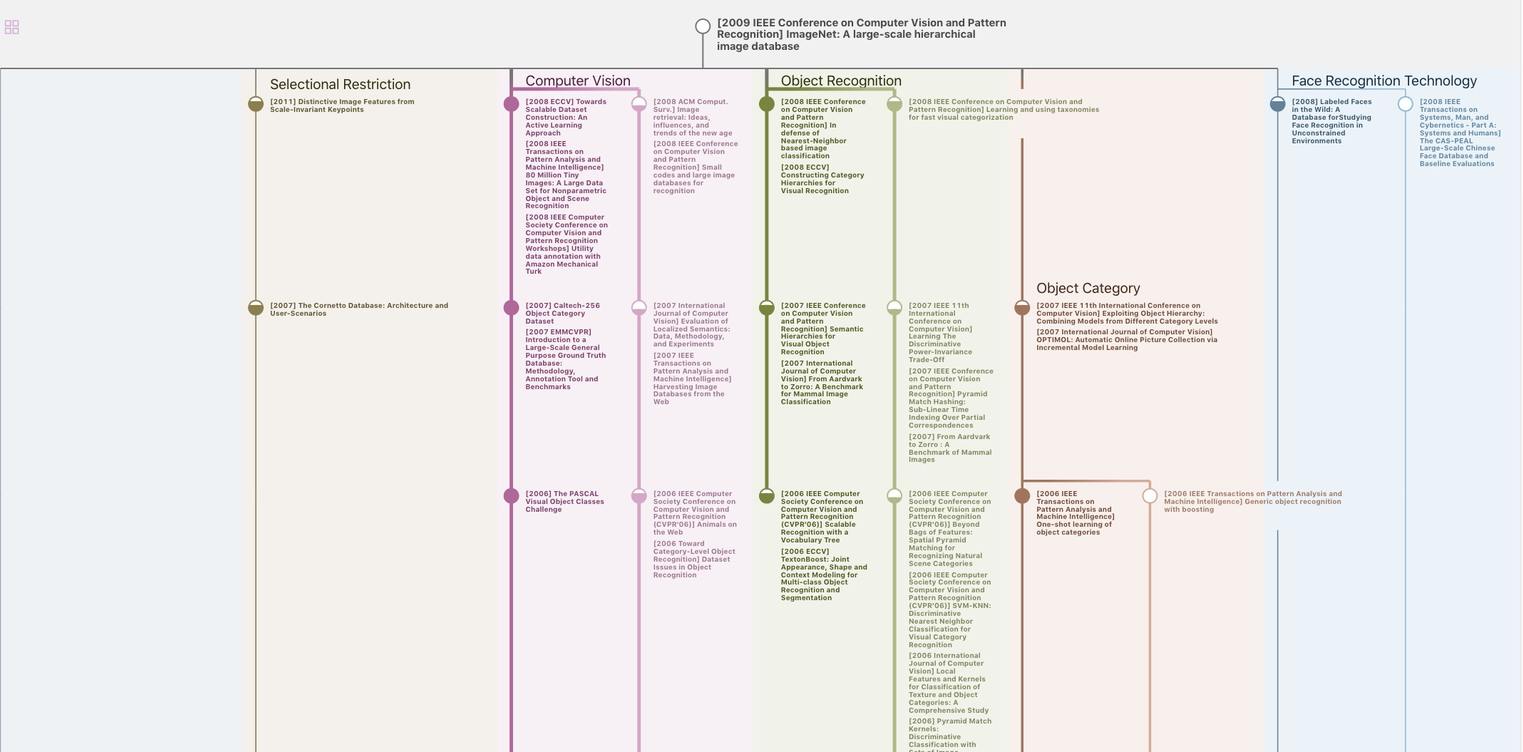
生成溯源树,研究论文发展脉络
Chat Paper
正在生成论文摘要