Selective attention to audiovisual speech routes activity through recurrent feedback-feedforward loops between different nodes of the speech network
bioRxiv (Cold Spring Harbor Laboratory)(2024)
摘要
Selective attention related top-down modulation plays a significant role in separating relevant speech from irrelevant background speech when vocal attributes separating concurrent speakers are small and continuously evolving. Electrophysiological studies have shown that such top-down modulation enhances neural tracking of attended speech. Yet, the specific cortical regions involved remain unclear due to the limited spatial resolution of most electrophysiological techniques. To overcome such limitations, we collected both EEG (high temporal resolution) and fMRI (high spatial resolution), while human participants selectively attended to speakers in audiovisual scenes containing overlapping cocktail party speech. To utilize the advantages of the respective techniques, we analysed neural tracking of speech using the EEG data and performed representational dissimilarity-based EEG-fMRI fusion. We observed that attention enhanced neural tracking and modulated EEG correlates throughout the latencies studied. Further, attention related enhancement of neural tracking fluctuated in predictable temporal profiles. We discuss how such temporal dynamics could arise from a combination of interactions between attention and prediction as well as plastic properties of the auditory cortex. EEG-fMRI fusion revealed attention related iterative feedforward-feedback loops between hierarchically organised nodes of the ventral auditory object related processing stream. Our findings support models where attention facilitates dynamic neural changes in the auditory cortex, ultimately aiding discrimination of relevant sounds from irrelevant ones while conserving neural resources.
### Competing Interest Statement
The authors have declared no competing interest.
更多查看译文
关键词
audiovisual speech,selective attention,feedback-feedforward
AI 理解论文
溯源树
样例
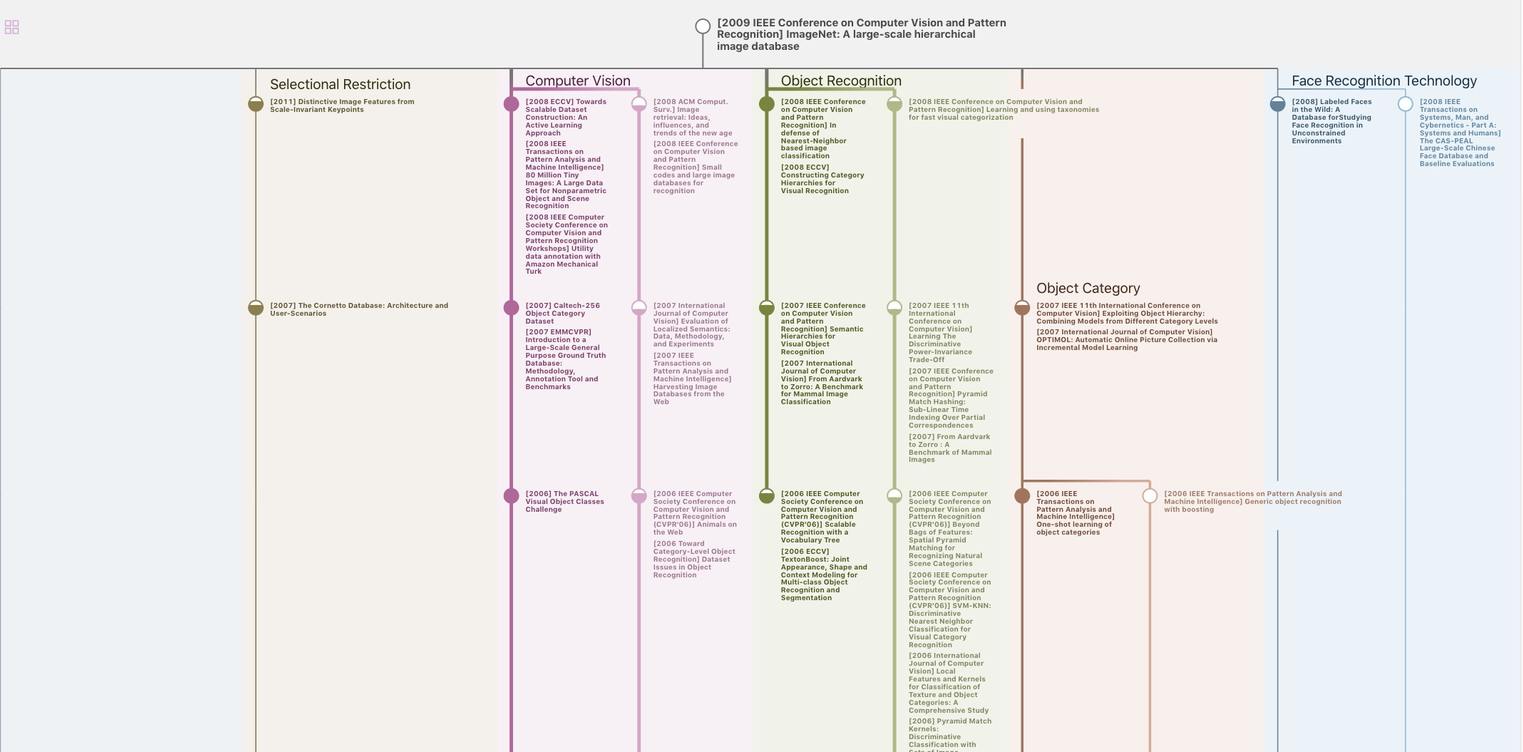
生成溯源树,研究论文发展脉络
Chat Paper
正在生成论文摘要