Image Captioning Using Transformer-Based Double Attention Network.
Engineering applications of artificial intelligence(2023)
摘要
Image captioning generates a human-like description for a query image, which has attracted considerable attention recently. The most broadly utilized model for image description is an encoder–decoder structure, where the encoder extracts the visual information of the image, and the decoder generates textual descriptions of the image. Transformers have significantly enhanced the performance of image description models. However, a single attention structure in transformers cannot consider more complex relationships between key and query vectors. Furthermore, attention weights are assigned to entire candidate vectors based on the assumption that entire vectors are related. In this paper, a new double-attention framework is presented, which improves the encoder–decoder structure to consider image captioning problems. Hence, a local generator module and a global generator module are designed to predict textual descriptions collaboratively. The proposed approach improves Self-Attention (SA) from two aspects to enhance the performance of image description. First, a Masked Self-Attention module is presented to attend on the most relevant information. Second, to evade a single shallow attention distribution and make deeper internal relations, a Hybrid Weight Distribution (HWD) module is proposed, that develops SA to use the relations between key and query vectors efficiently. Experiments over the Flickr30k and MS-COCO datasets prove that the proposed approach achieves desirable performance on different evaluation measures compared to the state-of-the-art frameworks.
更多查看译文
关键词
Self-attention,Transformer,Image captioning,Encoder–decoder
AI 理解论文
溯源树
样例
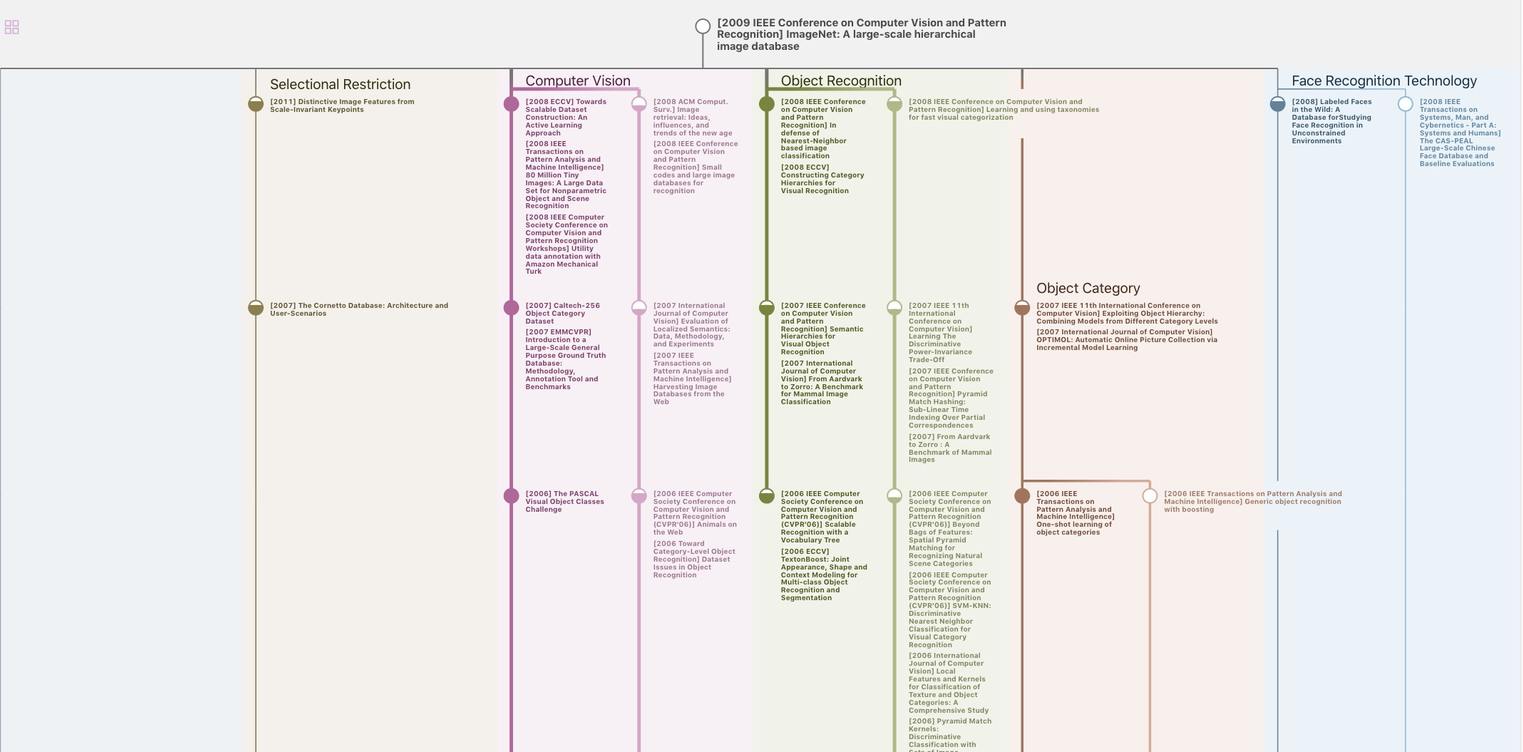
生成溯源树,研究论文发展脉络
Chat Paper
正在生成论文摘要