Linear-Scaling Kernels for Protein Sequences and Small Molecules Outperform Deep Learning While Providing Uncertainty Quantitation and Improved Interpretability.
JOURNAL OF CHEMICAL INFORMATION AND MODELING(2023)
摘要
Gaussian process (GP) is a Bayesian model which provides several advantages for regression tasks in machine learning such as reliable quantitation of uncertainty and improved interpretability. Their adoption has been precluded by their excessive computational cost and by the difficulty in adapting them for analyzing sequences (e.g., amino acid sequences) and graphs (e.g., small molecules). In this study, we introduce a group of random feature-approximated kernels for sequences and graphs that exhibit linear scaling with both the size of the training set and the size of the sequences or graphs. We incorporate these new kernels into our new Python library for GP regression, xGPR, and develop an efficient and scalable algorithm for fitting GPs equipped with these kernels to large datasets. We compare the performance of xGPR on 17 different benchmarks with both standard and state-of-the-art deep learning models and find that GP regression achieves highly competitive accuracy for these tasks while providing with well-calibrated uncertainty quantitation and improved interpretability. Finally, in a simple experiment, we illustrate how xGPR may be used as part of an active learning strategy to engineer a protein with a desired property in an automated way without human intervention.
更多查看译文
关键词
deep learning,protein sequences,linear-scaling
AI 理解论文
溯源树
样例
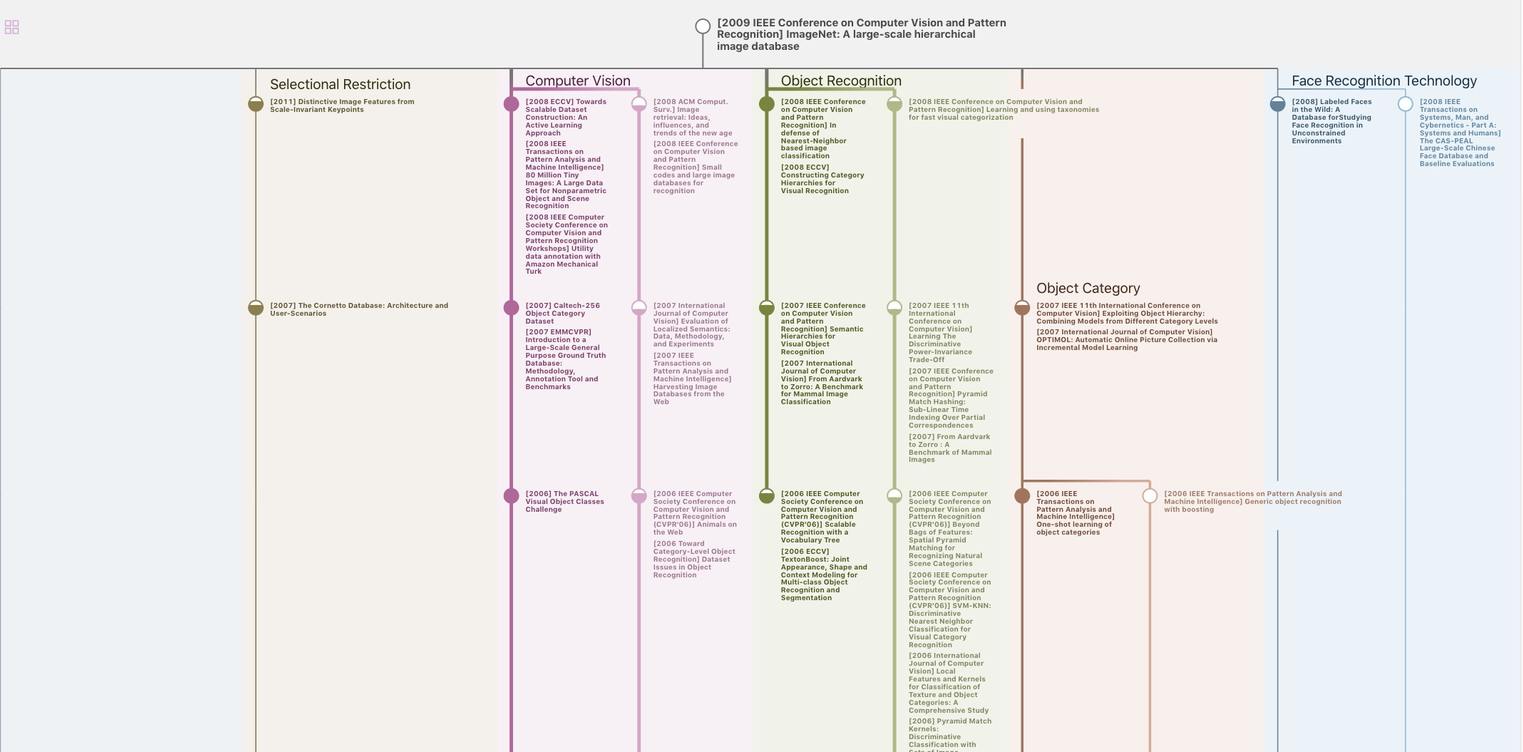
生成溯源树,研究论文发展脉络
Chat Paper
正在生成论文摘要