A Geometric Notion of Causal Probing
CoRR(2023)
摘要
Large language models rely on real-valued representations of text to make their predictions. These representations contain information learned from the data that the model has trained on, including knowledge of linguistic properties and forms of demographic bias, e.g., based on gender. A growing body of work has considered information about concepts such as these using orthogonal projections onto subspaces of the representation space. We contribute to this body of work by proposing a formal definition of intrinsic information in a subspace of a language model's representation space. We propose a counterfactual approach that avoids the failure mode of spurious correlations (Kumar et al., 2022) by treating components in the subspace and its orthogonal complement independently. We show that our counterfactual notion of information in a subspace is optimizing by an causal concept subspace. Furthermore, this intervention allows us to attempt concept controlled generation by manipulating the value of the conceptual component of a representation. Empirically, we find that R-LACE (Ravfogel et al., 2022) returns a one-dimensional subspace containing roughly half of total concept information under our framework. Our causal controlled intervention shows that, for at least one model, the subspace returned by R-LACE can be used to manipulate the concept value of the generated word with precision.
更多查看译文
关键词
geometric notion
AI 理解论文
溯源树
样例
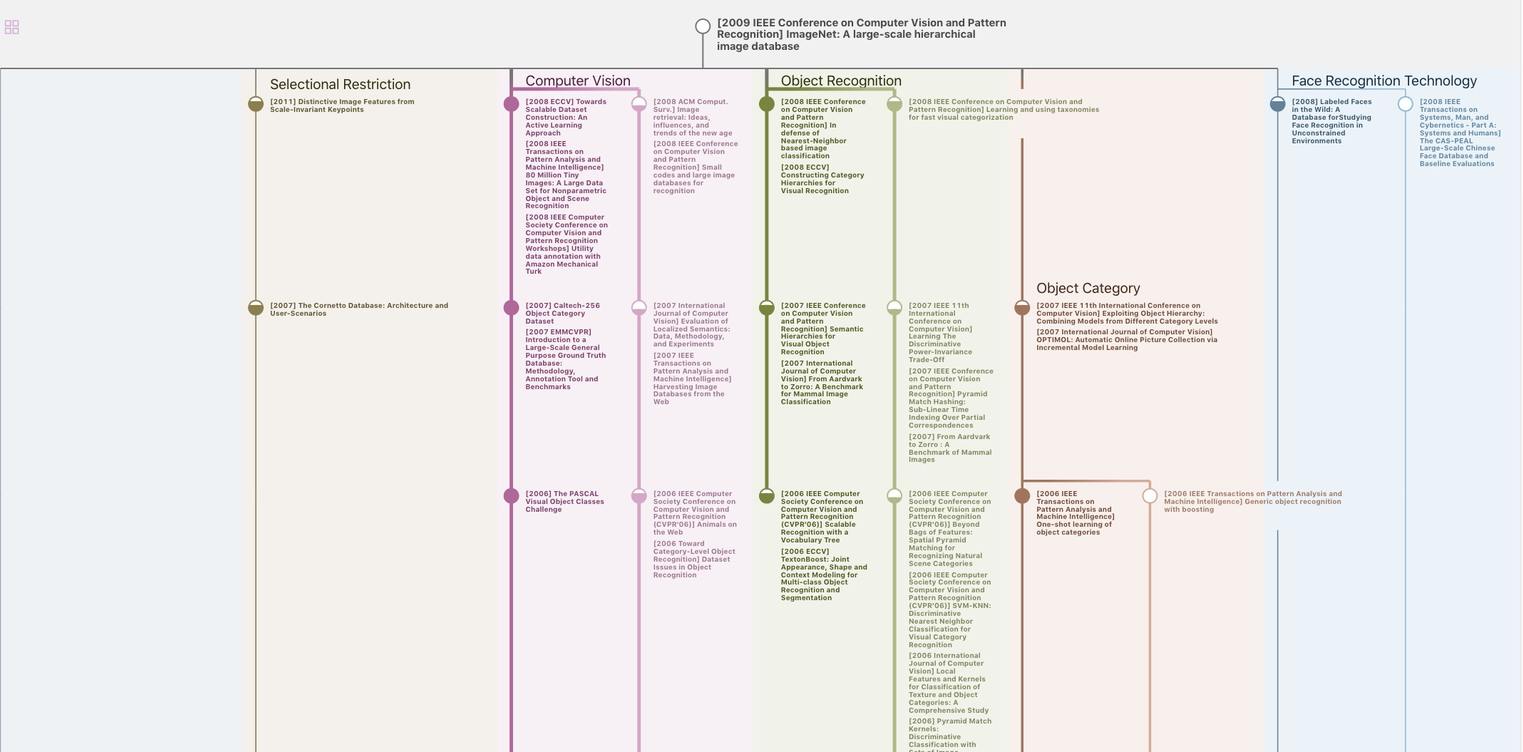
生成溯源树,研究论文发展脉络
Chat Paper
正在生成论文摘要