Determination of α S using OPAL hadronic event shapes at √(s) = 91209 GeV and resummed NNLO calculations
The European Physical Journal C(2011)
Dipartimento di Fisica dell’ Università di Bologna and INFN | Cavendish Laboratory | CERN | Department of Physics and Astronomy | School of Physics and Astronomy | Enrico Fermi Institute and Department of Physics | International Centre for Elementary Particle Physics and Department of Physics | Department of Physics | Max-Planck-Institute für Physik | Institut für Experimentalphysik | Rutherford Appleton Laboratory | Ottawa-Carleton Institute for Physics | Ludwig-Maximilians-Universität München | Fakultät für Physik | Physikalisches Institut | Queen Mary and Westfield College | Research Institute for Particle and Nuclear Physics | Institute of Nuclear Research | Particle Physics Department | Laboratoire de Physique Nucléaire | Technische Hochschule Aachen | University College London
- Pretraining has recently greatly promoted the development of natural language processing (NLP)
- We show that M6 outperforms the baselines in multimodal downstream tasks, and the large M6 with 10 parameters can reach a better performance
- We propose a method called M6 that is able to process information of multiple modalities and perform both single-modal and cross-modal understanding and generation
- The model is scaled to large model with 10 billion parameters with sophisticated deployment, and the 10 -parameter M6-large is the largest pretrained model in Chinese
- Experimental results show that our proposed M6 outperforms the baseline in a number of downstream tasks concerning both single modality and multiple modalities We will continue the pretraining of extremely large models by increasing data to explore the limit of its performance
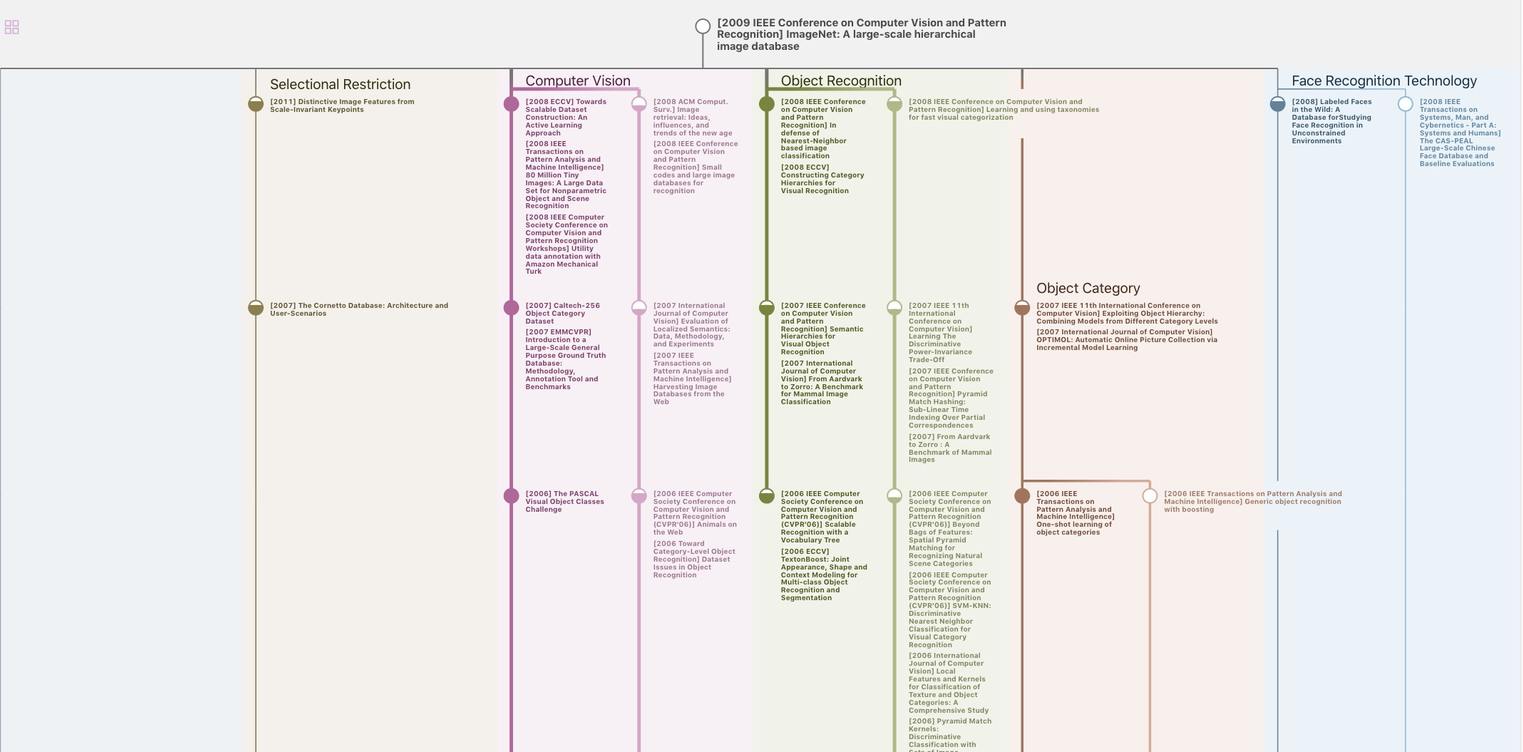