LLMs as Workers in Human-Computational Algorithms? Replicating Crowdsourcing Pipelines with LLMs
CoRR(2023)
摘要
LLMs have shown promise in replicating human-like behavior in crowdsourcing tasks that were previously thought to be exclusive to human abilities. However, current efforts focus mainly on simple atomic tasks. We explore whether LLMs can replicate more complex crowdsourcing pipelines. We find that modern LLMs can simulate some of crowdworkers' abilities in these "human computation algorithms," but the level of success is variable and influenced by requesters' understanding of LLM capabilities, the specific skills required for sub-tasks, and the optimal interaction modality for performing these sub-tasks. We reflect on human and LLMs' different sensitivities to instructions, stress the importance of enabling human-facing safeguards for LLMs, and discuss the potential of training humans and LLMs with complementary skill sets. Crucially, we show that replicating crowdsourcing pipelines offers a valuable platform to investigate (1) the relative strengths of LLMs on different tasks (by cross-comparing their performances on sub-tasks) and (2) LLMs' potential in complex tasks, where they can complete part of the tasks while leaving others to humans.
更多查看译文
关键词
crowdsourcing pipelines,llms,algorithms,workers,human-computational
AI 理解论文
溯源树
样例
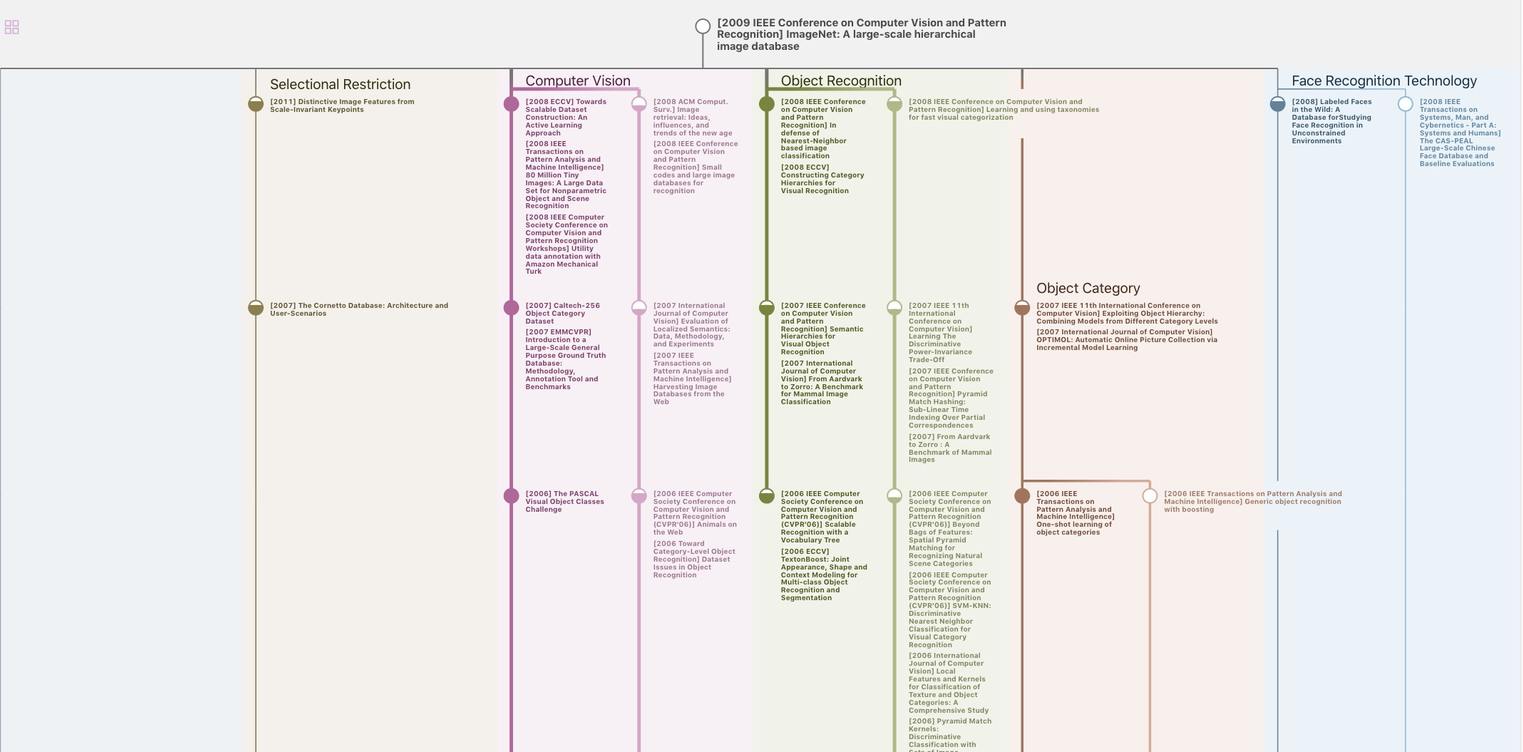
生成溯源树,研究论文发展脉络
Chat Paper
正在生成论文摘要