Supervised Dynamic PCA: Linear Dynamic Forecasting with Many Predictors
arXiv (Cornell University)(2023)
摘要
This paper proposes a novel dynamic forecasting method using a new supervised Principal Component Analysis (PCA) when a large number of predictors are available. The new supervised PCA provides an effective way to bridge the gap between predictors and the target variable of interest by scaling and combining the predictors and their lagged values, resulting in an effective dynamic forecasting. Unlike the traditional diffusion-index approach, which does not learn the relationships between the predictors and the target variable before conducting PCA, we first re-scale each predictor according to their significance in forecasting the targeted variable in a dynamic fashion, and a PCA is then applied to a re-scaled and additive panel, which establishes a connection between the predictability of the PCA factors and the target variable. Furthermore, we also propose to use penalized methods such as the LASSO approach to select the significant factors that have superior predictive power over the others. Theoretically, we show that our estimators are consistent and outperform the traditional methods in prediction under some mild conditions. We conduct extensive simulations to verify that the proposed method produces satisfactory forecasting results and outperforms most of the existing methods using the traditional PCA. A real example of predicting U.S. macroeconomic variables using a large number of predictors showcases that our method fares better than most of the existing ones in applications. The proposed method thus provides a comprehensive and effective approach for dynamic forecasting in high-dimensional data analysis.
更多查看译文
关键词
supervised dynamic pca,linear dynamic forecasting,predictors
AI 理解论文
溯源树
样例
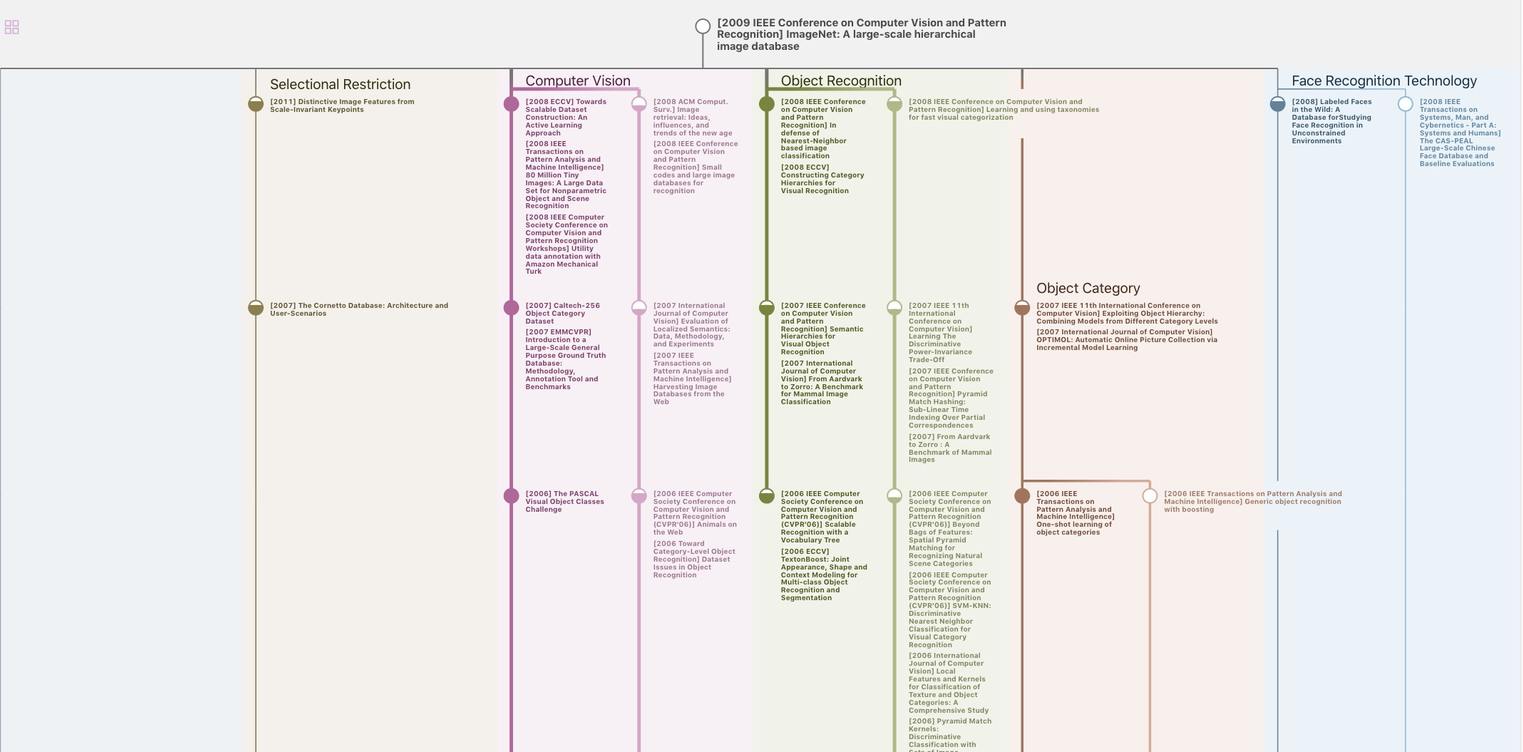
生成溯源树,研究论文发展脉络
Chat Paper
正在生成论文摘要