Invasiveness identification in pure ground-glass nodules: exploring the generalizability of radiomics based on external validation and stress testing
Journal of cancer research and clinical oncology(2023)
Abstract
Purpose This study aimed to apply external validation and stress tests to evaluate the generalizability of radiomics models built using various machine-learning methods for identifying the invasiveness of lung adenocarcinomas manifesting as pure ground-glass nodules (pGGNs). Methods This retrospective study enrolled 495 patients (514 pGGNs) confirmed as lung adenocarcinomas by postoperative pathology from three centers. All nodules were included in the primary cohort (randomly divided into training and test cohorts), two external validation cohorts, and two stress test cohorts. Six machine-learning radiomics models were constructed in the training cohort using the optimal features. Performance of radiomics models and clinical models were compared in primary cohort and external validation cohorts. The stress tests included stratified performance evaluation and shifted performance evaluation and contrastive evaluation under three single-condition modification settings. The predictive performance was validated by area under curve (AUC) of receiver operating characteristic (ROC). Results Of the six radiomics models, the best logistic regression (LR) model was able to maintain high differential diagnostic capability (AUC: 0.849 ± 0.049) and good stability (relative standard deviation, 5.719%), but it showed poorer performance (AUC = 0.835) than the clinical model (AUC = 0.862) in the external validation cohort E1. The stress tests suggested LR model had no significant difference in performance between subgroups after stratification and had good consistency in the predictions before and after the three transformations (Kappa = 0.960, 0.840, and 0.933, respectively; p < 0.05, all). Conclusion The rigorous testing procedure facilitates the selection of high-performance radiomics models with good clinical generalizability.
MoreTranslated text
Key words
Adenocarcinoma of lung,Machine learning,Solitary pulmonary nodule,Computer-aided diagnosis
AI Read Science
Must-Reading Tree
Example
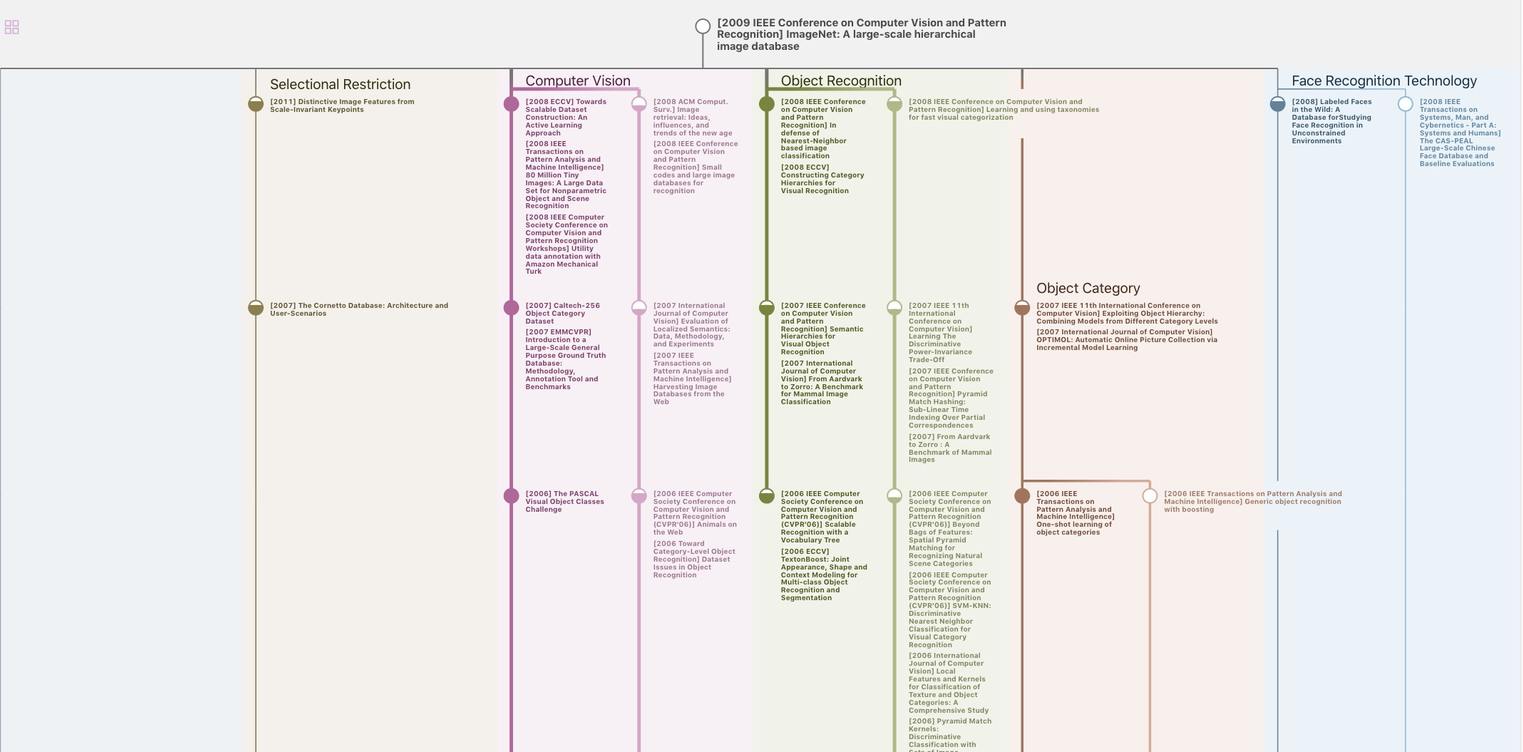
Generate MRT to find the research sequence of this paper
Chat Paper
Summary is being generated by the instructions you defined