Predicting Intraoperative Hypothermia Burden During Non-Cardiac Surgery: A Retrospective Study Comparing Regression to Six Machine Learning Algorithms.
JOURNAL OF CLINICAL MEDICINE(2023)
Med Univ Vienna | Ludwig Boltzmann Inst Digital Hlth & Patient Safet
Abstract
BACKGROUND:Inadvertent intraoperative hypothermia is a common complication that affects patient comfort and morbidity. As the development of hypothermia is a complex phenomenon, predicting it using machine learning (ML) algorithms may be superior to logistic regression.METHODS:We performed a single-center retrospective study and assembled a feature set comprised of 71 variables. The primary outcome was hypothermia burden, defined as the area under the intraoperative temperature curve below 37 °C over time. We built seven prediction models (logistic regression, extreme gradient boosting (XGBoost), random forest (RF), multi-layer perceptron neural network (MLP), linear discriminant analysis (LDA), k-nearest neighbor (KNN), and Gaussian naïve Bayes (GNB)) to predict whether patients would not develop hypothermia or would develop mild, moderate, or severe hypothermia. For each model, we assessed discrimination (F1 score, area under the receiver operating curve, precision, recall) and calibration (calibration-in-the-large, calibration intercept, calibration slope).RESULTS:We included data from 87,116 anesthesia cases. Predicting the hypothermia burden group using logistic regression yielded a weighted F1 score of 0.397. Ranked from highest to lowest weighted F1 score, the ML algorithms performed as follows: XGBoost (0.44), RF (0.418), LDA (0.406), LDA (0.4), KNN (0.362), and GNB (0.32).CONCLUSIONS:ML is suitable for predicting intraoperative hypothermia and could be applied in clinical practice.
MoreTranslated text
Key words
anesthesia,surgery,hypothermia,prediction,machine learning
求助PDF
上传PDF
View via Publisher
AI Read Science
AI Summary
AI Summary is the key point extracted automatically understanding the full text of the paper, including the background, methods, results, conclusions, icons and other key content, so that you can get the outline of the paper at a glance.
Example
Background
Key content
Introduction
Methods
Results
Related work
Fund
Key content
- Pretraining has recently greatly promoted the development of natural language processing (NLP)
- We show that M6 outperforms the baselines in multimodal downstream tasks, and the large M6 with 10 parameters can reach a better performance
- We propose a method called M6 that is able to process information of multiple modalities and perform both single-modal and cross-modal understanding and generation
- The model is scaled to large model with 10 billion parameters with sophisticated deployment, and the 10 -parameter M6-large is the largest pretrained model in Chinese
- Experimental results show that our proposed M6 outperforms the baseline in a number of downstream tasks concerning both single modality and multiple modalities We will continue the pretraining of extremely large models by increasing data to explore the limit of its performance
Upload PDF to Generate Summary
Must-Reading Tree
Example
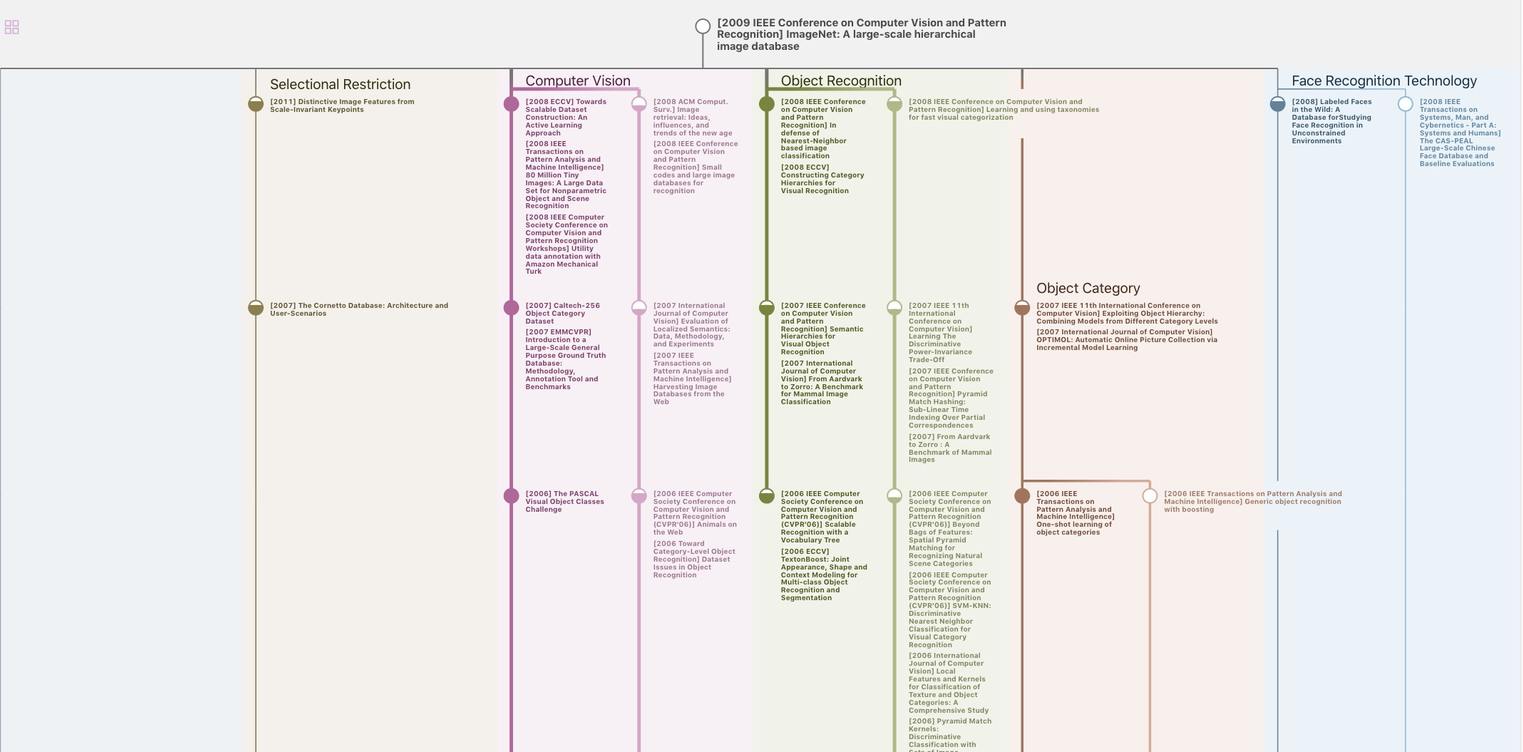
Generate MRT to find the research sequence of this paper
Related Papers
Opportunities of AI-powered Applications in Anesthesiology to Enhance Patient Safety.
International Anesthesiology Clinics 2024
被引用0
Data Disclaimer
The page data are from open Internet sources, cooperative publishers and automatic analysis results through AI technology. We do not make any commitments and guarantees for the validity, accuracy, correctness, reliability, completeness and timeliness of the page data. If you have any questions, please contact us by email: report@aminer.cn
Chat Paper
GPU is busy, summary generation fails
Rerequest