A Novel BILSTM-CNN Method for Pattern Recognition in Real Time Under Triple Physical Loads in Lower Extremity Exoskeleton
IEEE Sensors Journal(2023)
摘要
Pattern recognition is of great importance in compliant control of the lower extremity exoskeleton. We propose a novel method based on the bidirectional long short-term memory-convolutional neural network (BILSTM-CNN) model for pattern recognition in real time under triple physical loads on different terrains. BILSTM is skilled in dealing with temporal series data, while CNN is proficient in coping with spatial series data. Five patterns include level ground walking (LW), stair ascending (SA), stair descending (SD), ramp ascending (RA), and ramp descending (RD). Their accuracies in multigrade loads of 0, 20, and 40 kg reach 98.81%, 98.24%, and 97.04%, respectively. Moreover, compared with the universal methods of long short-term memory (LSTM), CNN, and back propagation (BP), the hybrid method has a competitive advantage in evaluation indexes of accuracy, precision, recall, and
${F}1$
score. In addition to five steady patterns, eight pattern transitions are identified between two neighboring states, such as LW to SA, LW to SD, LW to RA, LW to RD, SA to LW, SD to LW, RA to LW, and RD to LW. For pattern transitions, the prediction time (Pre-T) of the next pattern in multilevel loads of 0, 20, and 40 kg are 190–620, 180–420, and 50–90 ms, respectively, before the step into that pattern. Pattern transition time (Aug-In) in multilevel loads of 0, 20, and 40 kg are 50–190, 30–310, and 0–280 ms, respectively, before Pre-T of the next pattern. Eventually, the experimental results indicate the proposed method has excellent performance in pattern recognition and pattern transition.
更多查看译文
关键词
Bidirectional long short-term memory-convolutional neural network (BILSTM-CNN), pattern recognition, pattern transition, triple physical loads
AI 理解论文
溯源树
样例
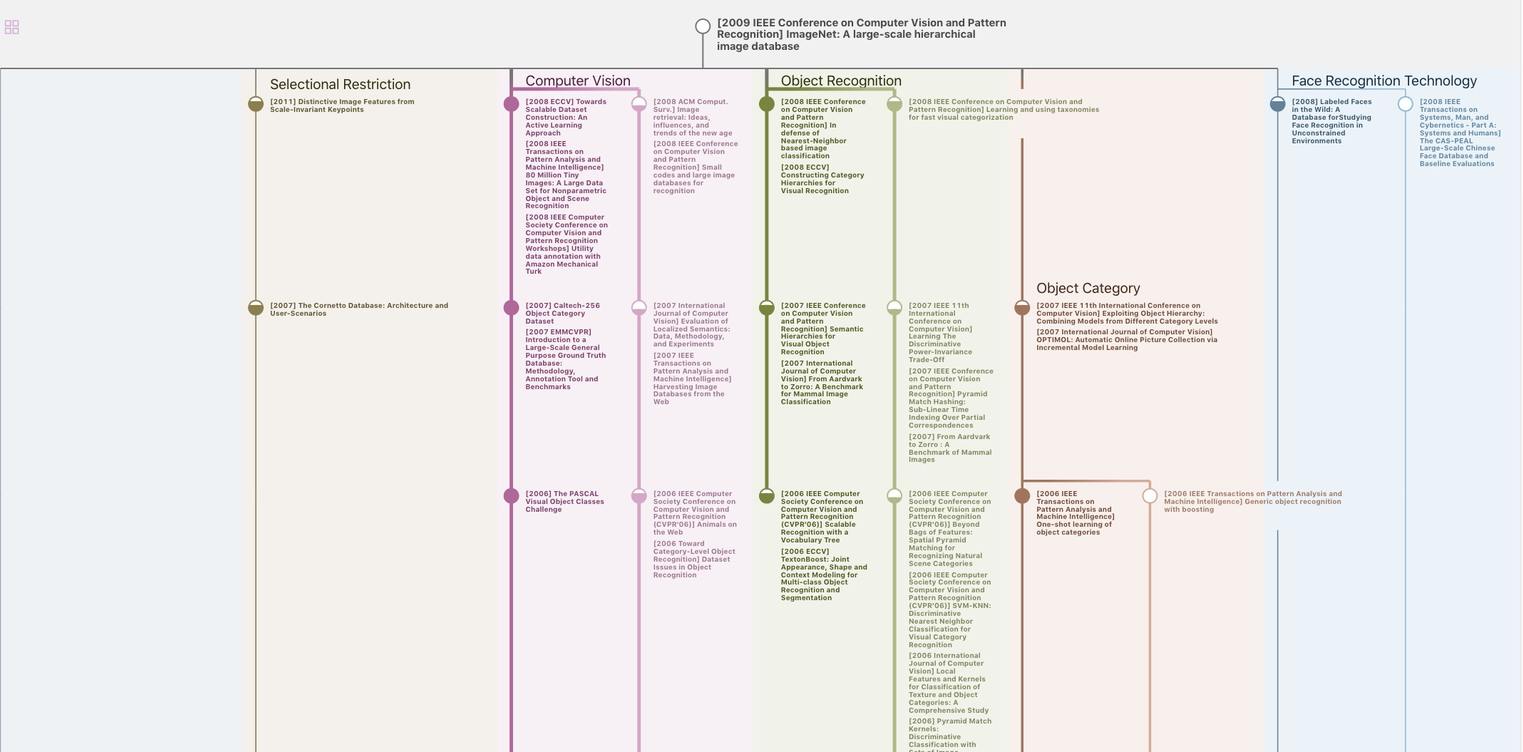
生成溯源树,研究论文发展脉络
Chat Paper
正在生成论文摘要