Evidence of Variable Human Fcγ Receptor-Fc Affinities Across Differentially-Complexed IgG.
MAbs(2023)
摘要
Antibody-mediated effector functions are widely considered to unfold according to an associative model of IgG-Fcγ receptor (FcγR) interactions. The associative model presupposes that Fc receptors cannot discriminate antigen-bound IgG from free IgG in solution and have equivalent affinities for each. Therefore, the clustering of Fcγ receptors (FcγR) in the cell membrane, cross-activation of intracellular signaling domains, and the formation of the immune synapse are all the result of avid interactions between the Fc region of IgG and FcγRs that collectively overcome the individually weak, transient interactions between binding partners. Antibody allostery, specifically conformational allostery, is a competing model in which antigen-bound antibody molecules undergo a physical rearrangement that causes them to stand out from the background of free IgG by virtue of greater FcγR affinity. Various evidence exists in support of this model of antibody allostery, but it remains controversial. We report observations from multiplexed, label-free kinetic experiments in which the affinity values of FcγR were characterized for covalently immobilized, captured, and antigen-bound IgG. Across the strategies tested, receptors had greater affinity for the antigen-bound mode of IgG presentation. This phenomenon was observed across multiple FcγRs and generalized to multiple antigens, antibody specificities, and subclasses. Furthermore, the thermodynamic signatures of FcγR binding to free or immune-complexed IgG in solution differed when measured by an orthogonal label-free method, but the failure to recapitulate the trend in overall affinity leaves open questions as to what additional factors may be at play.
更多查看译文
关键词
Affinity,allostery,antibody,Fc receptor,immunoglobulin G
AI 理解论文
溯源树
样例
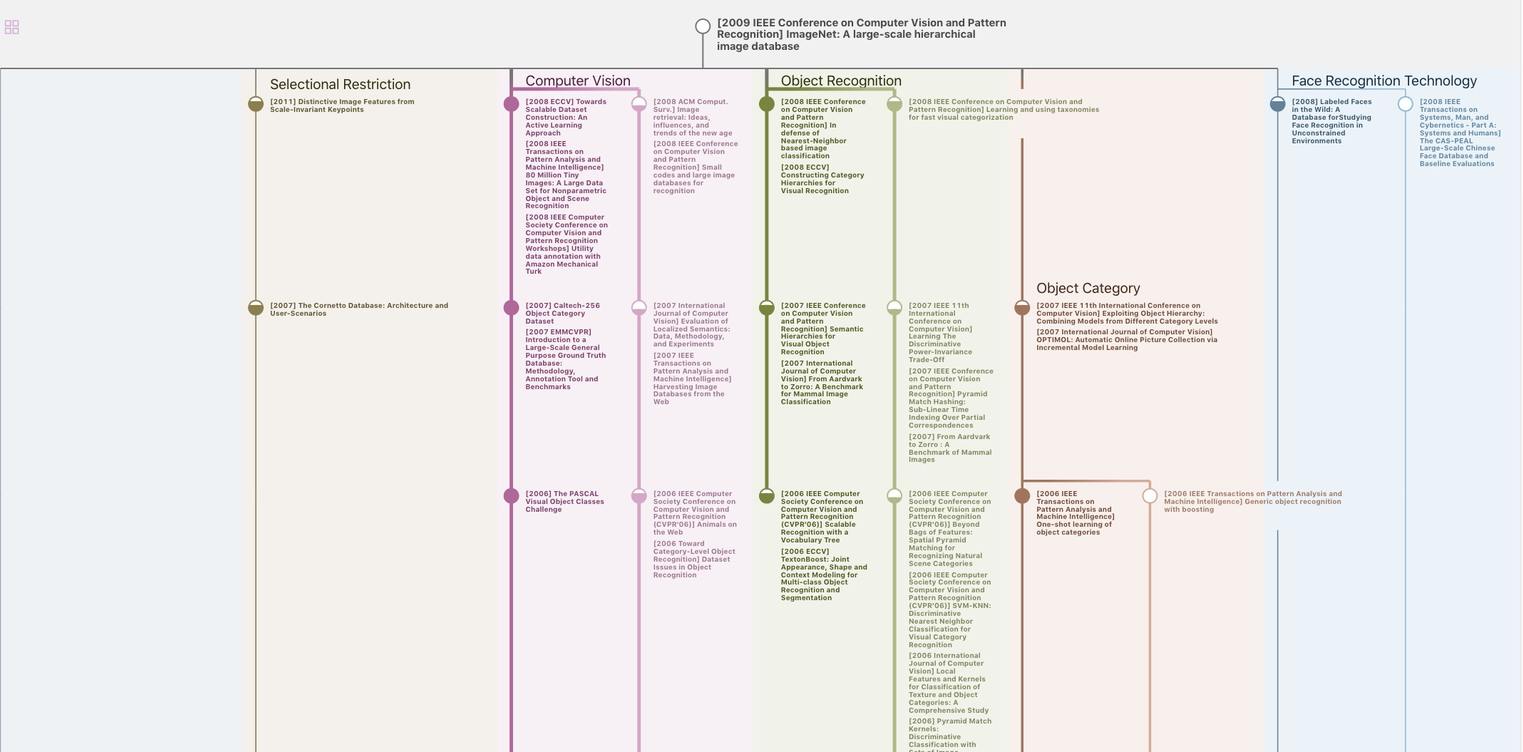
生成溯源树,研究论文发展脉络
Chat Paper
正在生成论文摘要