A Novel Framework to Predict Chlorophyll-a Concentrations in Water Bodies Through Multi-Source Big Data and Machine Learning Algorithms.
Environmental science and pollution research international(2023)
摘要
Eutrophication happens when water bodies are enriched by minerals and nutrients. Dense blooms of noxious are the most obvious effect of eutrophication that harms water quality, and by increasing toxic substances damage the water ecosystem. Therefore, it is critical to monitor and investigate the development process of eutrophication. The concentration of chlorophyll-a (chl-a) in water bodies is an essential indicator of eutrophication in them. Previous studies in predicting chlorophyll-a concentrations suffered from low spatial resolution and discrepancies between predicted and observed values. In this paper, we used various remote sensing and ground observation data and proposed a novel machine learning-based framework, a random forest inversion model, to provide the spatial distribution of chl-a in 2 m spatial resolution. The results showed our model outperformed other base models, and the goodness of fit improved by over 36.6% while MSE and MAE decreased by over 15.17% and over 21.26% respectively. Moreover, we compared the feasibility of GF-1 and Sentinel-2 remote sensing data in chl-a concentration prediction. We found that better prediction results can be obtained by using GF-1 data, with the goodness of fit reaching 93.1% and MSE only 3.589. The proposed method and findings of this study can be used in future water management studies and as an aid for decision-makers in this field.
更多查看译文
关键词
Remote sensing,Random forest,Water pollution,Eutrophication,Spatial analysis
AI 理解论文
溯源树
样例
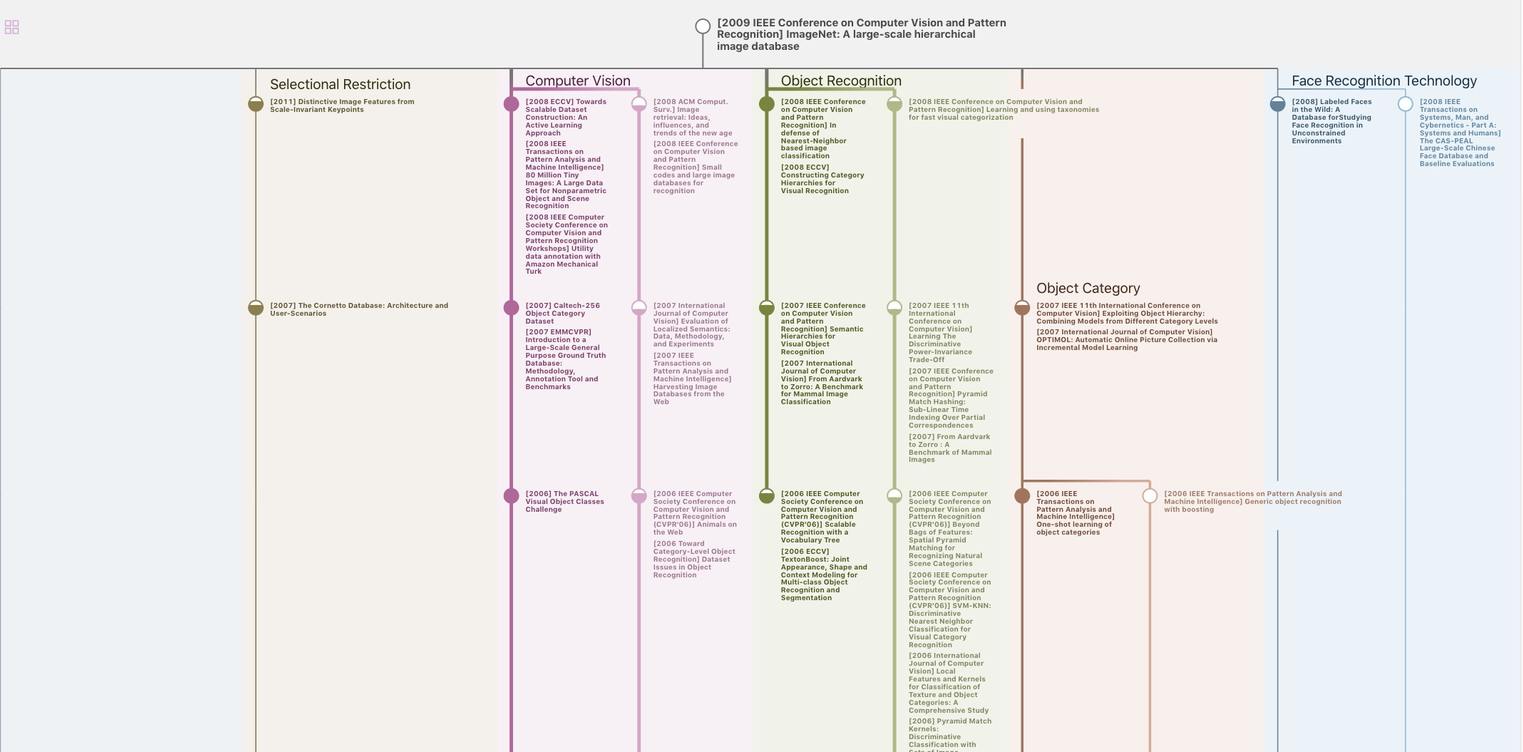
生成溯源树,研究论文发展脉络
Chat Paper
正在生成论文摘要