From Boring to Bravo! Using Learning Science to Create Memorable Presentations.
American Society of Clinical Oncology educational book American Society of Clinical Oncology Annual Meeting(2023)
Department of Medicine | Division of HematologyOncology
Abstract
The practice of oncology continues to evolve over time. Educators find themselves in a position where they are no longer able to teach a topic in its entirety. Moreover, the rapid expansion of information available through research and discovery in the field of oncology makes it difficult for learners to process the constant barrage of new content. Lecturers continue to impart knowledge using didactic techniques, often trying to include as much material as possible in the time permitted. The question becomes: In the face of an impossibly large field, how can one assist learners in learning, and retaining, what is most important? The science of learning continues to develop, and we now recognize that there are ways to teach that optimally facilitate the retention and application of knowledge. By using these strategies, educators can make it easier for learners to absorb and retain key information. This article will touch upon several such techniques: cognitive load optimization, analogy, contrasting cases, elaboration, and just-in-time telling. By applying these methods to didactic presentations, educators can ensure that their lessons are heard, understood, and ultimately transformed into something unforgettable.
MoreTranslated text
求助PDF
上传PDF
View via Publisher
AI Read Science
AI Summary
AI Summary is the key point extracted automatically understanding the full text of the paper, including the background, methods, results, conclusions, icons and other key content, so that you can get the outline of the paper at a glance.
Example
Background
Key content
Introduction
Methods
Results
Related work
Fund
Key content
- Pretraining has recently greatly promoted the development of natural language processing (NLP)
- We show that M6 outperforms the baselines in multimodal downstream tasks, and the large M6 with 10 parameters can reach a better performance
- We propose a method called M6 that is able to process information of multiple modalities and perform both single-modal and cross-modal understanding and generation
- The model is scaled to large model with 10 billion parameters with sophisticated deployment, and the 10 -parameter M6-large is the largest pretrained model in Chinese
- Experimental results show that our proposed M6 outperforms the baseline in a number of downstream tasks concerning both single modality and multiple modalities We will continue the pretraining of extremely large models by increasing data to explore the limit of its performance
Upload PDF to Generate Summary
Must-Reading Tree
Example
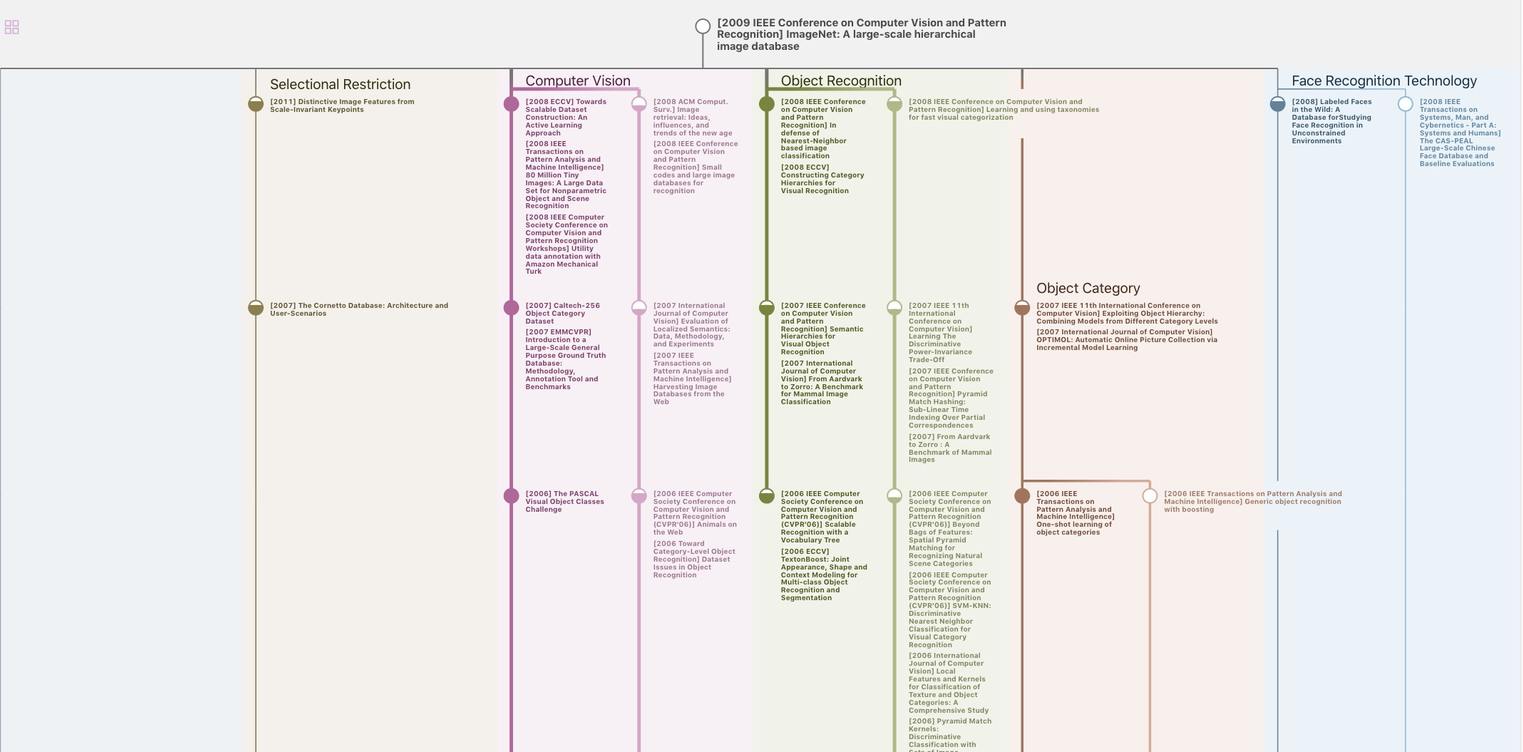
Generate MRT to find the research sequence of this paper
Related Papers
Data Disclaimer
The page data are from open Internet sources, cooperative publishers and automatic analysis results through AI technology. We do not make any commitments and guarantees for the validity, accuracy, correctness, reliability, completeness and timeliness of the page data. If you have any questions, please contact us by email: report@aminer.cn
Chat Paper