MDMA: Multimodal Data and Multi-attention Based Deep Learning Model for Alzheimer’s Disease Diagnosis
2023 8th International Conference on Cloud Computing and Big Data Analytics (ICCCBDA)(2023)
摘要
Recently, multimodal data-based methods have shown excellent performance in Alzheimer’s Disease(AD) diagnosis. However, these methods commonly have the following two shortcomings: 1) the feature extraction processes of different modalities are independent and lack cooperation, which may lead to limited representation ability of the extracted features, and 2) the multimodal fusion operation is a simple concatenation, thus causing rough fusion features. To address these two issues, we propose a deep learning network based on multimodal data and multi-attention(MDMA), which consists of two key components, namely Cross-Modal Channel and Spatial attention (CMCS) and Cross-Modal Cross-Attention (CMCA). The CMCS module uses the interaction information from MRI and PET to recalibrate both channel-wise and spatial features for each modality. The CMCA module utilizes two multi-head cross attention to interactively fuse information from images and clinical data. In addition, Gradient-weighted Class Activation Mapping (Grad-CAM) method is used for visualizing the focused areas of the proposed model to make our model more transparent. Evaluated on the ADNI dataset with multimodal data collected from 113 AD, 146 mild cognitive impairment (MCI), and 135 normal controls (NC), we demonstrate that the proposed model achieves better results in terms of accuracy, sensitivity and specificity.
更多查看译文
关键词
alzheimer’s disease,multimodal fusion,deep learning,atttention mechanism,classification
AI 理解论文
溯源树
样例
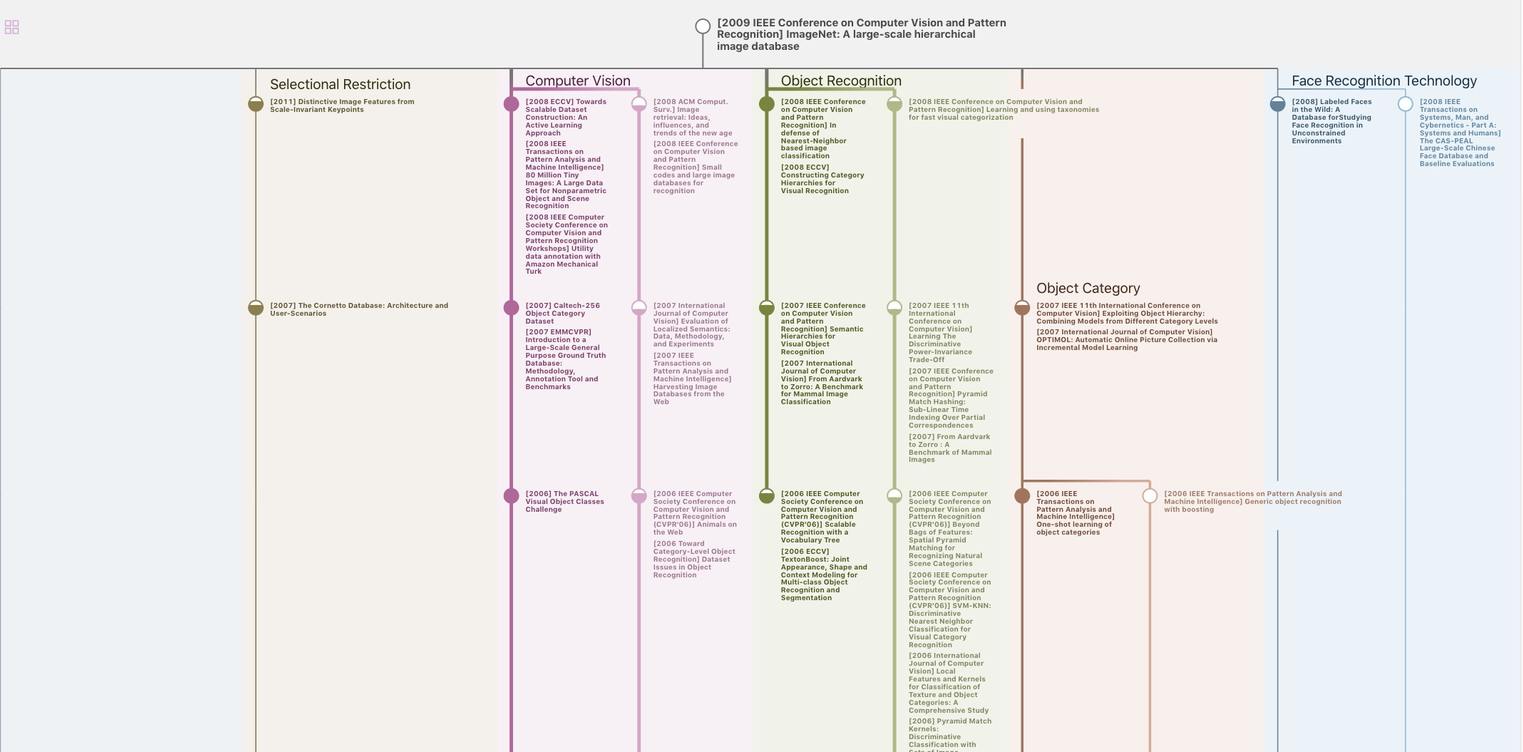
生成溯源树,研究论文发展脉络
Chat Paper
正在生成论文摘要