An integrated approach based on the correction of imbalanced small datasets and the application of machine learning algorithms to predict total phosphorus concentration in rivers
Ecological Informatics(2023)
摘要
Increased concentrations of Total Phosphorus (TP) in freshwater systems lead to eutrophication and can contribute to a wide range of environmental effects. In the modern era, water quality models have increasingly been used globally for the development of management scenarios with the aim of reducing the eutrophication risk. However, the accuracy of these models is limited by the quality of the boundary conditions forcing data, namely TP concentration datasets. In this study, a novel methodology is proposed to improve machine learning prediction accuracy in the modeling of river TP concentration forced with small input training datasets. These models can then be used to increase the quality and consistency of the TP concentration datasets required to force water quality models. This new methodology relies on the generation of 100 new training datasets from the raw training datasets of input predictors through the implementation of an over/undersampling technique. The modeling approach used in this study was supported by the application of ten machine learning algorithms to estimate the TP concentration values in 22 rivers located in Portugal. The modeling approach also included an input feature importance evaluation, as well as model hyperparameter optimization. In general terms, the Extreme Gradient Boosting (XGBoost) and Support Vector Regressor (SVR) models performed best overall, with the ensemble results recorded for both models working to increase the mean Nash-Sutcliffe efficiency (NSE) across all the areas being studied by 96% (0.01 ± 0.22 to 0.31 ± 0.32) and reduce the mean percentage bias (PBIAS) by 43% (18.47 ± 17.31 to 10.60 ± 17.40). The results of this study suggest that the solution proposed has the potential to significantly improve the modeling of TP concentration in rivers with machine learning methods, as well as providing increased scope for its application to larger training datasets and the prediction of other types of dependent variables. Hopefully, the results of this study will further add to the body of information available in this area of research and aid the development of the water management process.
更多查看译文
关键词
Total phosphorus, Machine learning, Rivers, Bayesian optimization, Data augmentation
AI 理解论文
溯源树
样例
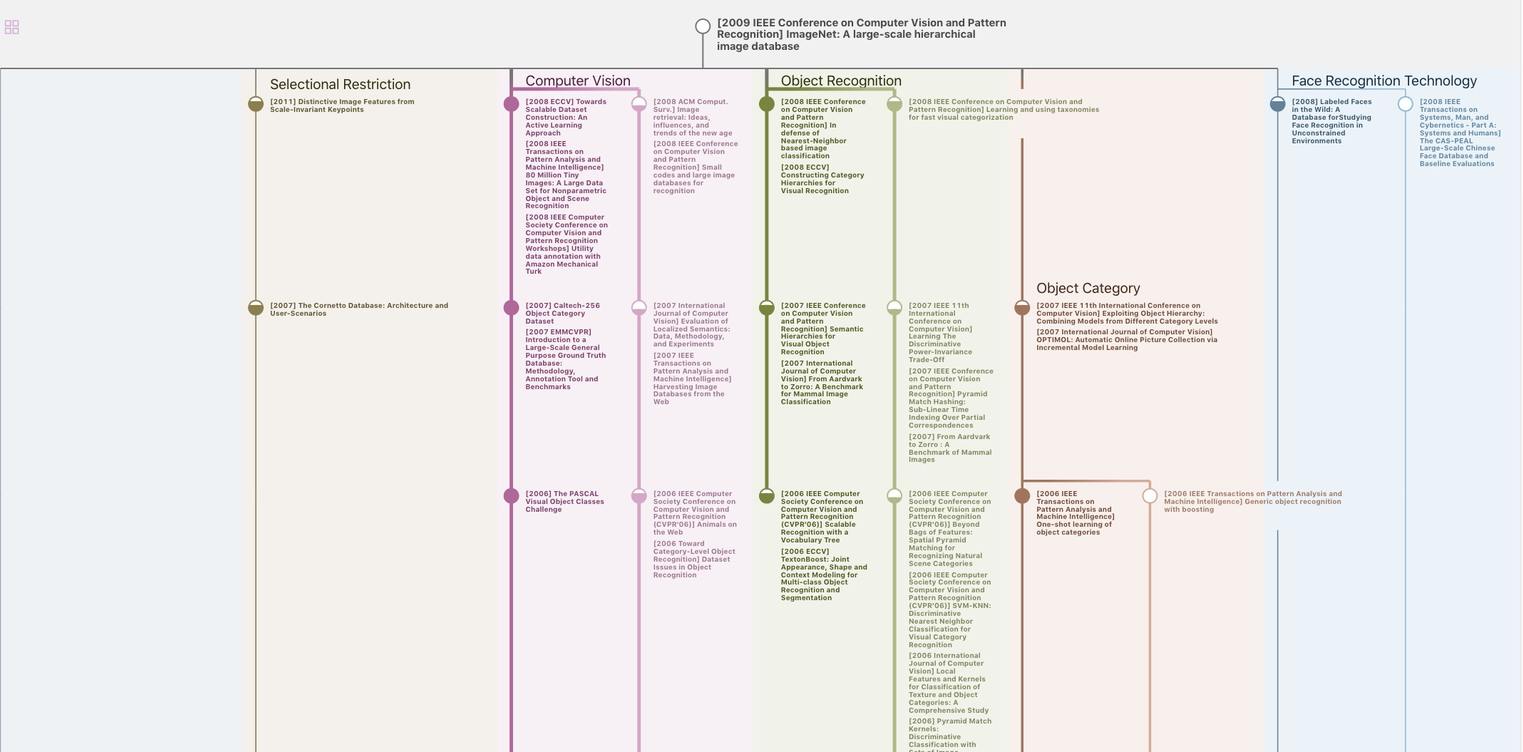
生成溯源树,研究论文发展脉络
Chat Paper
正在生成论文摘要