Effect of Sequence Padding on the Performance of Protein-Based Deep Learning Models
Research Square (Research Square)(2020)
摘要
Abstract Background The use of raw amino acid sequences as input for protein-based deep learning models has gained popularity in recent years. This scheme obliges to manage proteins with different lengths, while deep learning models require same-shape input. To accomplish this, zeros are usually added to each sequence up to a established common length in a process called zero-padding. However, the effect of different padding strategies on model performance and data structure is yet unknown. Results We analysed the impact of different ways of padding the amino acid sequences in a hierarchical Enzyme Commission number prediction problem. Our results show that padding has an effect on model performance even when there are convolutional layers implied. We propose and implement four novel types of padding the amino acid sequences. Conclusions The present study highlights the relevance of the step of padding the one-hot encoded amino acid sequences when building deep learning-based models for Enzyme Commission number prediction. The fact that this has an effect on model performance should raise awareness on the need of justifying the details of this step on future works. The code of this analysis is available at https://github.com/b2slab/padding_benchmark.
更多查看译文
关键词
sequence padding,deep learning models,deep learning,protein-based
AI 理解论文
溯源树
样例
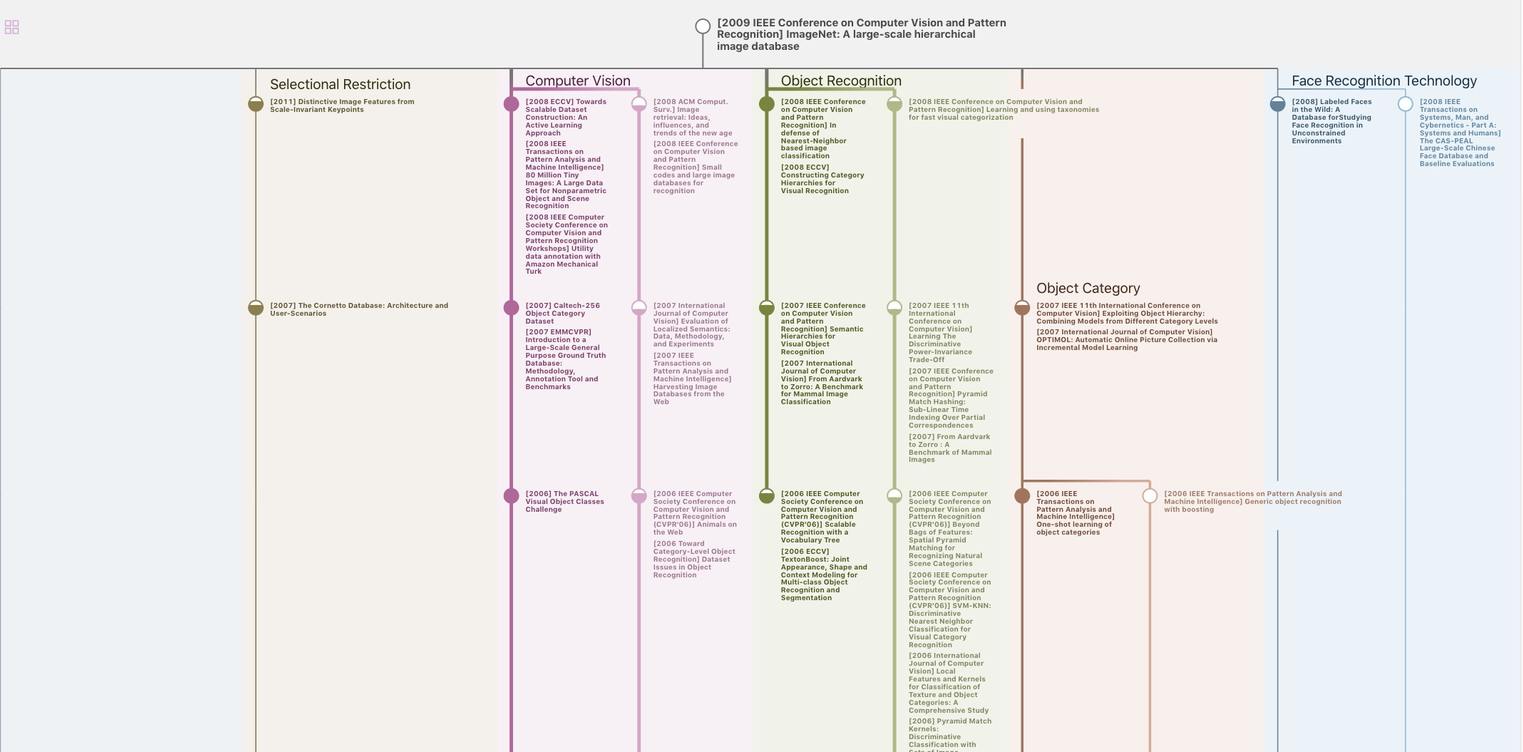
生成溯源树,研究论文发展脉络
Chat Paper
正在生成论文摘要