A consistent multi-method global extreme event attribution framework
crossref(2020)
摘要
In order to streamline observational and global climate model based extreme event attribution techniques, we propose a multi-method framework which drastically increases the robustness of rapid attribution studies, hence further facilitating the communication of extreme weather related risks across the globe. We use advanced observational datasets for temperature (Berkeley Earth) and rainfall (CPC), together with CMIP5 simulations and the large HadRM3P ensemble from the weather@home project (W@H) Recent (Climatology) and current/future warming scenarios (1°C, 1.5°C, 2°C, 3°C and 4°C) are juxtaposed to pre-industrial (Natural) baseline conditions. Two scaling approaches are applied to the observational data to estimate the statistics of future warming scenarios. One in which percentiles of the metric of interest (Tmax, Tmin, Precip) are scaled with Global Mean Surface Temperature (GMST) and another in which the mean is scaled against GMST. Model subsetting (similar to the HAPPI experiment) as function of GMST is applied to the CMIP5 data in order to assign the warming thresholds. W@H scenarios are prescribed to achieve the desired warming threshold. We analyse the results in terms of classes of events, using percentiles, absolute and return-time based thresholds. Before the subsetting, model biases are removed means of quantile-mapping (both for CMIP5 and W@H). The results between both scaling methods and model subsetting are mostly consistent across many regions and virtually for all temperature thresholds under consideration. The percentile-based scaling method does, however, reveal that the tail of the distributions (highest Tmax, lowest Tmin) has potentially widened with warming. Overall, we find that historically rare extreme events become increasingly common in the future as far as Tmax and Precip is concerned. In contrast, cold extremes become increasingly rare.
更多查看译文
AI 理解论文
溯源树
样例
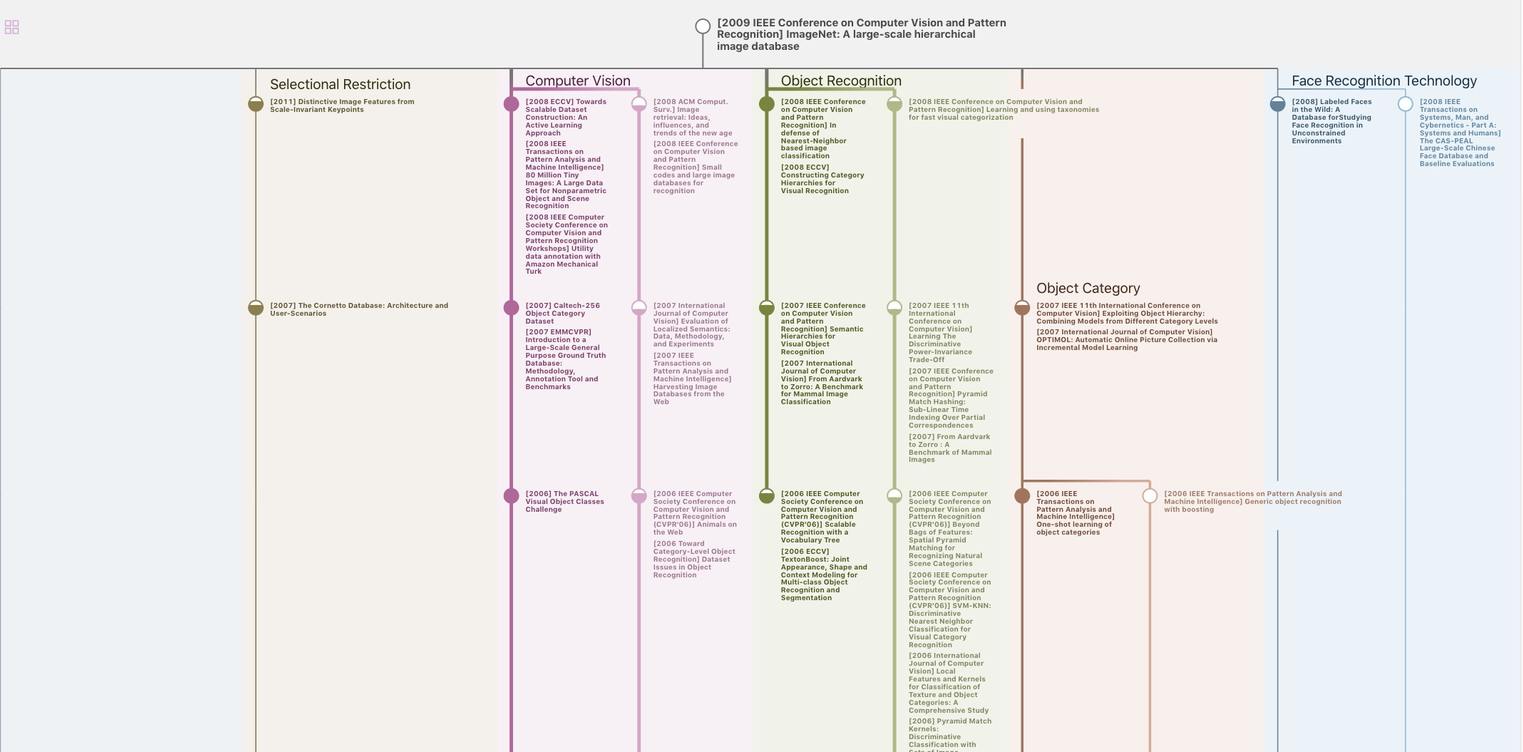
生成溯源树,研究论文发展脉络
Chat Paper
正在生成论文摘要