Classification of Vulnerability Levels Using Multivariate Biomarkers in Schizophrenia: a Machine-Learning Approach
crossref(2020)
摘要
Abstract Background: Schizophrenia is a heterogeneous neurodevelopmental disease involving cognitive and motor impairments. Motor dysfunctions, such as eye movements or neurological soft signs, are proposed as endophenotypic markers. Methods: Supervised machine-learning methods (Support Vector Machines) applied on oculomotor performances using comprehensive testing with prosaccades, antisaccades, memory-guided saccade tasks and smooth pursuit, as well as neurological soft signs assessment, was used to discriminate patients with schizophrenia (SZ, N=53), full siblings of patients (FS, N=45) and healthy volunteers (C, N=48). 80% of patients were used in a training/validation set and 20% on a test set. The discrimination was measured using the classification error (rate of misclassified patients).Results: The most reliable classification was between C and SZ, with only 15% and 12% of error rates for validation and test, whereas the SZ vs. FS classification provided the highest error rates (32% of error rate in both validation and test). Interestingly, neurological soft signs were selected as the best predictor, together with a combination of measures, for the two classifications: C vs. SZ, SZ vs. FS. In addition, memory-guided saccades were consistently selected among the best two multimodal features for the classifications involving the control group (C vs. SZ or FS). Conclusions: Taken together, these results emphasize the importance of neurological soft signs and sensitive oculomotor parameters, especially memory-guided saccades. This classification provides promising avenues for improving early detection of / early intervention in psychosis.
更多查看译文
AI 理解论文
溯源树
样例
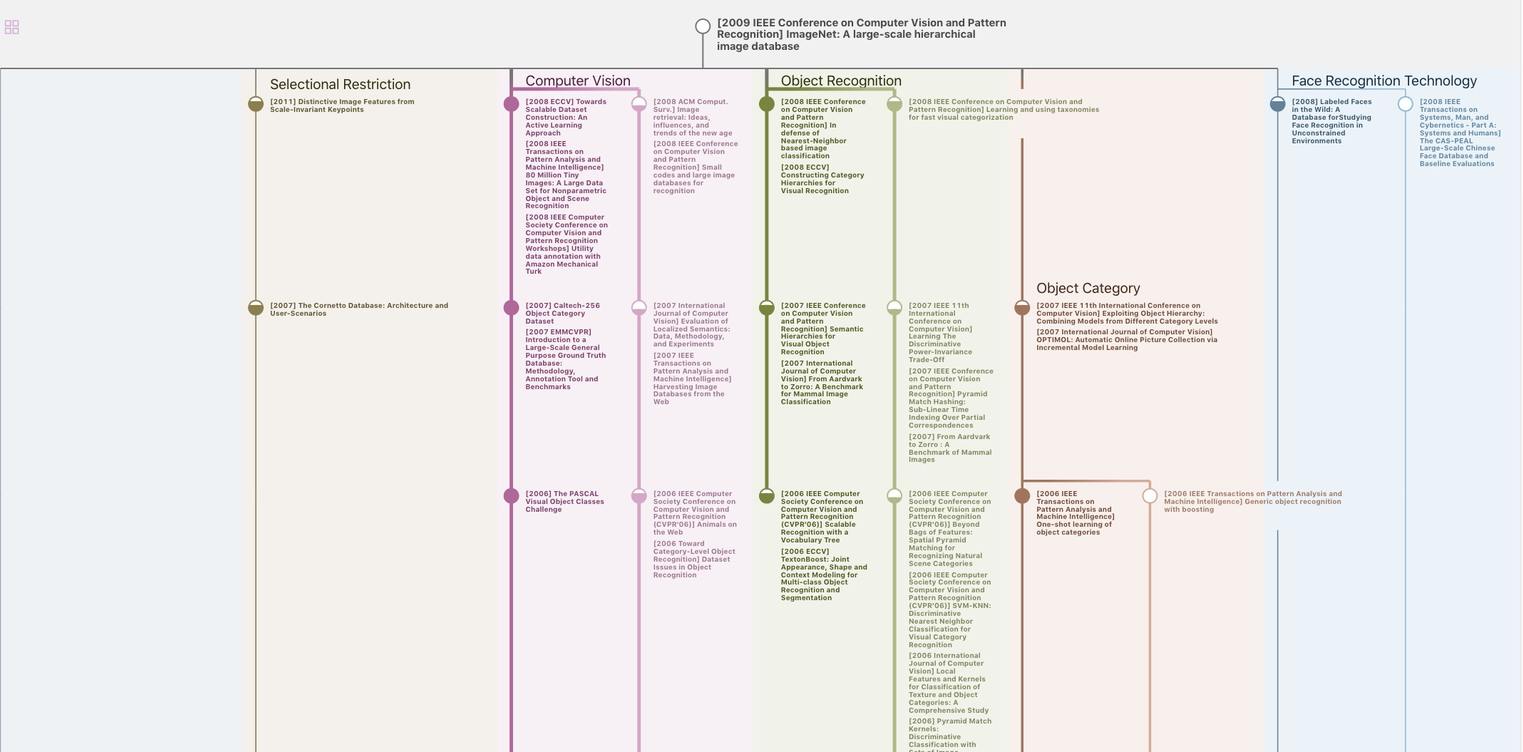
生成溯源树,研究论文发展脉络
Chat Paper
正在生成论文摘要