A Variational Autoencoder Trained with Priors from Canonical Pathways Increases the Interpretability of Transcriptome Data
PLOS COMPUTATIONAL BIOLOGY(2024)
摘要
Interpreting transcriptome data is an important yet challenging aspect of bioinformatic analysis. While gene set enrichment analysis is a standard tool for interpreting regulatory changes, we utilize deep learning techniques, specifically autoencoder architectures, to learn latent variables that drive transcriptome signals. We investigate whether simple, variational autoencoder (VAE), and beta-weighted VAE are capable of learning reduced representations of transcriptomes that retain critical biological information. We propose a novel VAE that utilizes priors from biological data to direct the network to learn a representation of the transcriptome that is based on understandable biological concepts. After benchmarking five different autoencoder architectures, we found that each succeeded in reducing the transcriptomes to 50 latent dimensions, which captured enough variation for accurate reconstruction. The simple, fully connected autoencoder, performs best across the benchmarks, but lacks the characteristic of having directly interpretable latent dimensions. The beta-weighted, prior-informed VAE implementation is able to solve the benchmarking tasks, and provide semantically accurate latent features equating to biological pathways. This study opens a new direction for differential pathway analysis in transcriptomics with increased transparency and interpretability.
更多查看译文
关键词
Transcriptomics,Pathway Analysis,Gene Set Enrichment Analysis
AI 理解论文
溯源树
样例
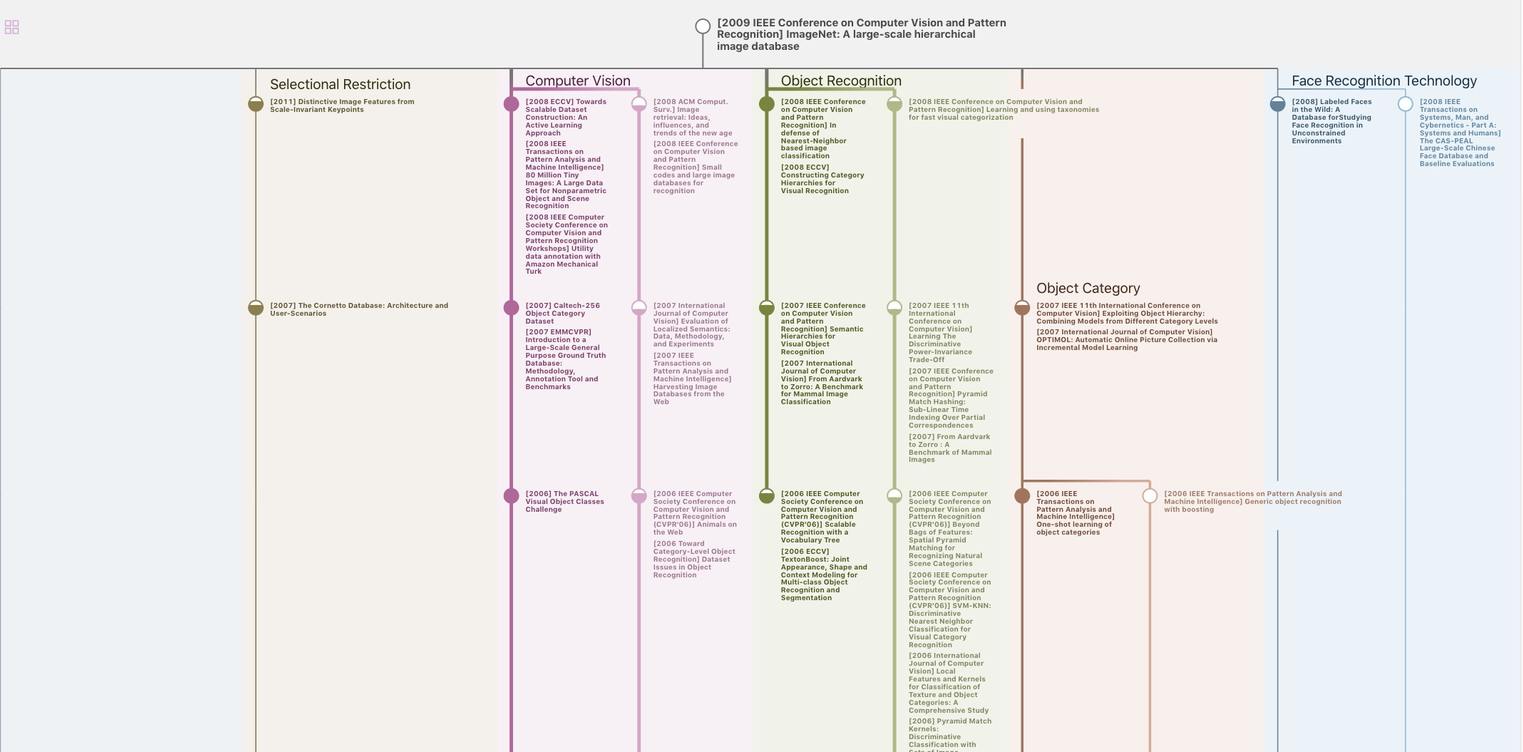
生成溯源树,研究论文发展脉络
Chat Paper
正在生成论文摘要