AVSegFormer: Audio-Visual Segmentation with Transformer
AAAI 2024(2024)
摘要
Audio-visual segmentation (AVS) aims to locate and segment the sounding objects in a given video, which demands audio-driven pixel-level scene understanding. The existing methods cannot fully process the fine-grained correlations between audio and visual cues across various situations dynamically. They also face challenges in adapting to complex scenarios, such as evolving audio, the coexistence of multiple objects, and more. In this paper, we propose AVSegFormer, a novel framework for AVS that leverages the transformer architecture. Specifically, It comprises a dense audio-visual mixer, which can dynamically adjust interested visual features, and a sparse audio-visual decoder, which implicitly separates audio sources and automatically matches optimal visual features. Combining both components provides a more robust bidirectional conditional multi-modal representation, improving the segmentation performance in different scenarios. Extensive experiments demonstrate that AVSegFormer achieves state-of-the-art results on the AVS benchmark. The code is available at https://github.com/vvvb-github/AVSegFormer.
更多查看译文
关键词
ML: Multimodal Learning,ML: Deep Learning Algorithms,ML: Transfer, Domain Adaptation, Multi-Task Learning
AI 理解论文
溯源树
样例
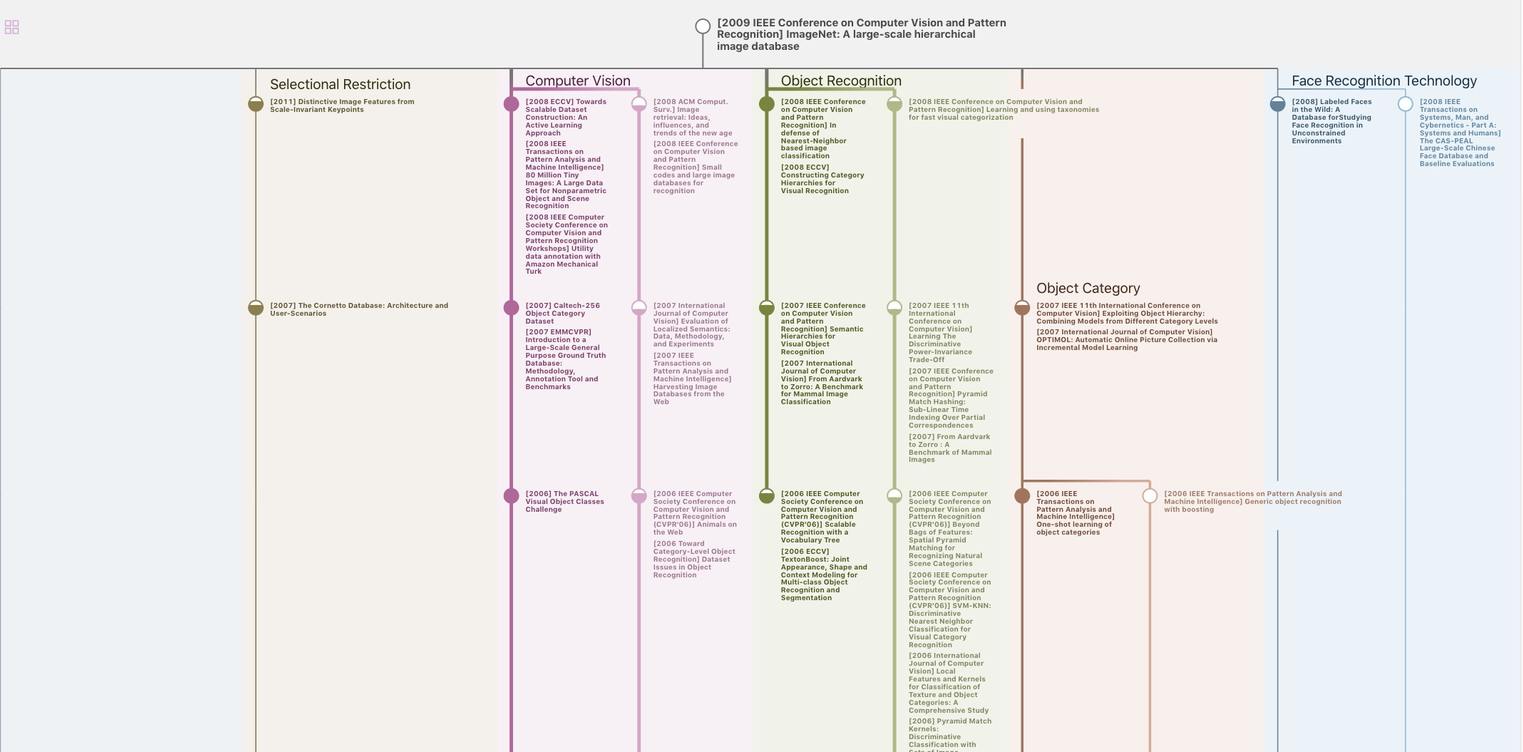
生成溯源树,研究论文发展脉络
Chat Paper
正在生成论文摘要