Power Transformer Oil Temperature Prediction Based on Empirical Mode Decomposition-Bidirectional Long Short-Term Memory
Journal of Algorithms & Computational Technology(2023)
摘要
Power transformers are crucial components of power transmission and transformation networks. Their operational status has a direct impact on the reliability of power supply systems. As such, the security and stability of power systems depend heavily on the state of transformers within them. The oil temperature of a transformer is a critical indicator of its working condition. Accurately and rapidly predicting transformer oil temperature is therefore of significant practical importance for ensuring the safe and effective operation of power systems. To address this prediction problem, this article proposes a transformer oil temperature prediction method based on empirical mode decomposition-bidirectional long short-term memory (EMD-BiLSTM). The time series of oil temperature is first cleaned before being processed. Next, the EMD algorithm is used to decompose the time series into relatively stable components. The BiLSTM neural network is then utilized to predict the complex nonlinear long-term series. The proposed method is evaluated using the open data set Electricity Transformer Temperature (ETT)-small. Experimental results show that the EMD-BiLSTM model outperforms traditional LSTM, BiLSTM, EMD-BP, and Wavelet Transform-Bidirectional Long Short-Term Memory (WT-BiLSTM) methods, demonstrating that it is an effective and accurate prediction method for transformer oil temperature.
更多查看译文
关键词
Power transformer,working state,EMD-BiLSTM,Oil temperature prediction
AI 理解论文
溯源树
样例
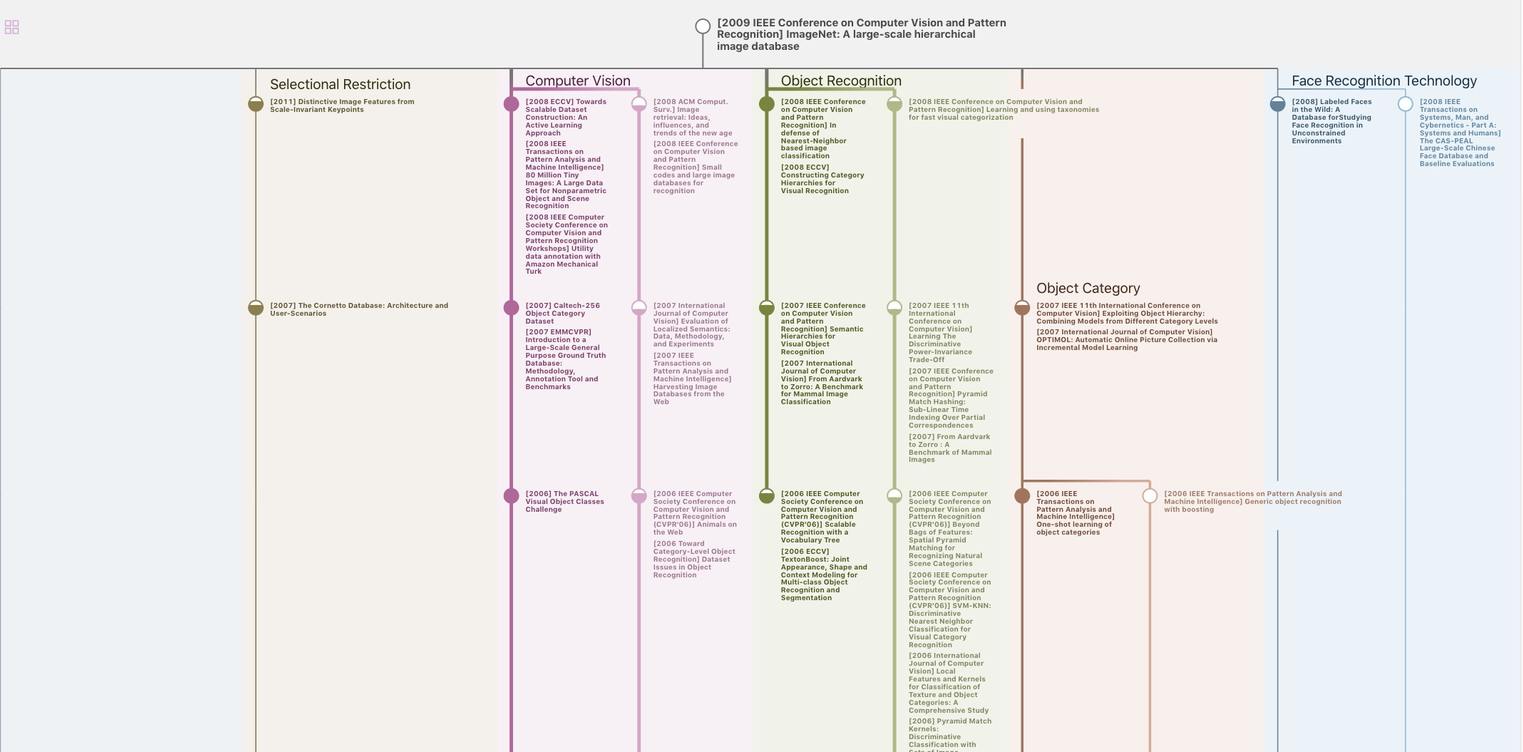
生成溯源树,研究论文发展脉络
Chat Paper
正在生成论文摘要