Fine Mapping of Qahps07 and Functional Studies of AhRUVBL2 Controlling Pod Size in Peanut (arachis Hypogaea L.).
Plant biotechnology journal(2023)SCI 1区
Abstract
SummaryCultivated peanut (Arachis hypogaea L.) is an important oil and cash crop. Pod size is one of the major traits determining yield and commodity characteristic of peanut. Fine mapping of quantitative trait locus (QTL) and identification of candidate genes associated with pod size are essential for genetic improvement and molecular breeding of peanut varieties. In this study, a major QTL related to pod size, qAHPS07, was fine mapped to a 36.46 kb interval on chromosome A07 using F2, recombinant inbred line (RIL) and secondary F2 populations. qAHPS07 explained 38.6%, 23.35%, 37.48%, 25.94% of the phenotypic variation for single pod weight (SPW), pod length (PL), pod width (PW) and pod shell thickness (PST), respectively. Whole genome resequencing and gene expression analysis revealed that a RuvB‐like 2 protein coding gene AhRUVBL2 was the most likely candidate for qAHPS07. Overexpression of AhRUVBL2 in Arabidopsis led to larger seeds and plants than the wild type. AhRUVBL2‐silenced peanut seedlings represented small leaves and shorter main stems. Three haplotypes were identified according to three SNPs in the promoter of AhRUVBL2 among 119 peanut accessions. Among them, SPW, PW and PST of accessions carrying Hap_ATT represent 17.6%, 11.2% and 26.3% higher than those carrying Hap_GAC,respectively. In addition, a functional marker of AhRUVBL2 was developed. Taken together, our study identified a key functional gene of peanut pod size, which provides new insights into peanut pod size regulation mechanism and offers practicable markers for the genetic improvement of pod size‐related traits in peanut breeding.
MoreTranslated text
Key words
peanut,pod size,fine mapping,qAHPS07,AhRUVBL2
求助PDF
上传PDF
View via Publisher
AI Read Science
AI Summary
AI Summary is the key point extracted automatically understanding the full text of the paper, including the background, methods, results, conclusions, icons and other key content, so that you can get the outline of the paper at a glance.
Example
Background
Key content
Introduction
Methods
Results
Related work
Fund
Key content
- Pretraining has recently greatly promoted the development of natural language processing (NLP)
- We show that M6 outperforms the baselines in multimodal downstream tasks, and the large M6 with 10 parameters can reach a better performance
- We propose a method called M6 that is able to process information of multiple modalities and perform both single-modal and cross-modal understanding and generation
- The model is scaled to large model with 10 billion parameters with sophisticated deployment, and the 10 -parameter M6-large is the largest pretrained model in Chinese
- Experimental results show that our proposed M6 outperforms the baseline in a number of downstream tasks concerning both single modality and multiple modalities We will continue the pretraining of extremely large models by increasing data to explore the limit of its performance
Upload PDF to Generate Summary
Must-Reading Tree
Example
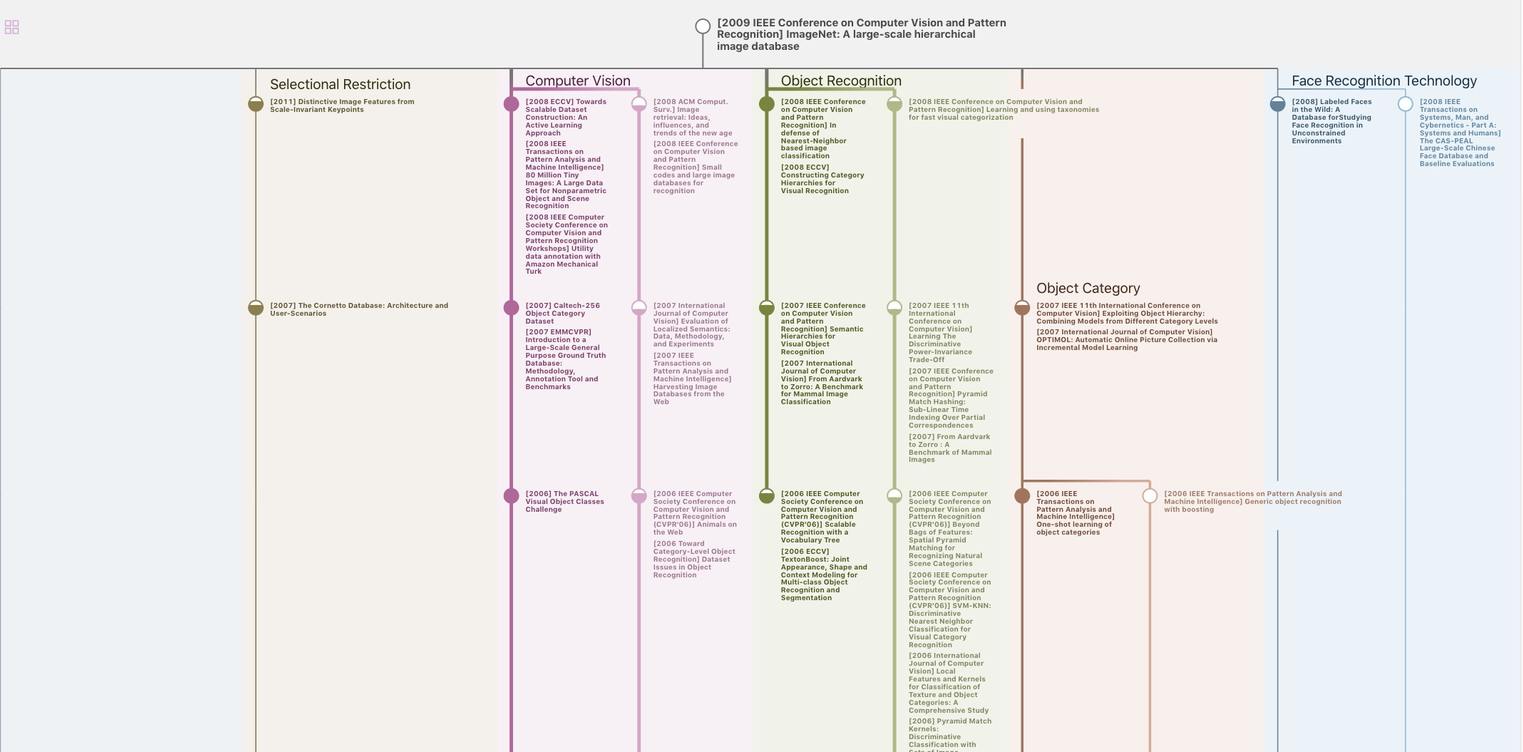
Generate MRT to find the research sequence of this paper
Related Papers
Euphytica 2023
被引用0
BMC GENOMICS 2023
被引用1
Designing Future Peanut: the Power of Genomics-Assisted Breeding
Theoretical and Applied Genetics 2024
被引用0
Data Disclaimer
The page data are from open Internet sources, cooperative publishers and automatic analysis results through AI technology. We do not make any commitments and guarantees for the validity, accuracy, correctness, reliability, completeness and timeliness of the page data. If you have any questions, please contact us by email: report@aminer.cn
Chat Paper