AFFNet: an Attention-Based Feature-Fused Network for Surface Defect Segmentation
Applied sciences(2023)
摘要
Recently, deep learning methods have widely been employed for surface defect segmentation in industrial production with remarkable success. Nevertheless, accurate segmentation of various types of defects is still challenging due to their irregular appearance and low contrast with the background. In light of this challenge, we propose an attention-based network with a U-shaped structure, referred to as AFFNet. In the encoder part, we present a newly designed module, Residual-RepGhost-Dblock (RRD), which focuses on the extraction of more representative features using CA attention and dilated convolution with varying expansion rates without a concomitant increase in the parameters. In the decoder part, we introduce a novel global feature attention (GFA) module to selectively fuse low-level and high-level features, suppressing distracting information such as background. Moreover, considering the imbalance of the dataset sampled from actual industrial production and the difficulty of training samples with small defects, we use the online hard sample mining (OHEM) cross-entropy loss function to improve the learning ability of hard samples. Experimental results on the NEU-seg dataset demonstrate the superiority of our method over other state-of-the-art methods.
更多查看译文
关键词
deep CNN,surface defect segmentation,U-shape architecture,feature attention,feature fusion
AI 理解论文
溯源树
样例
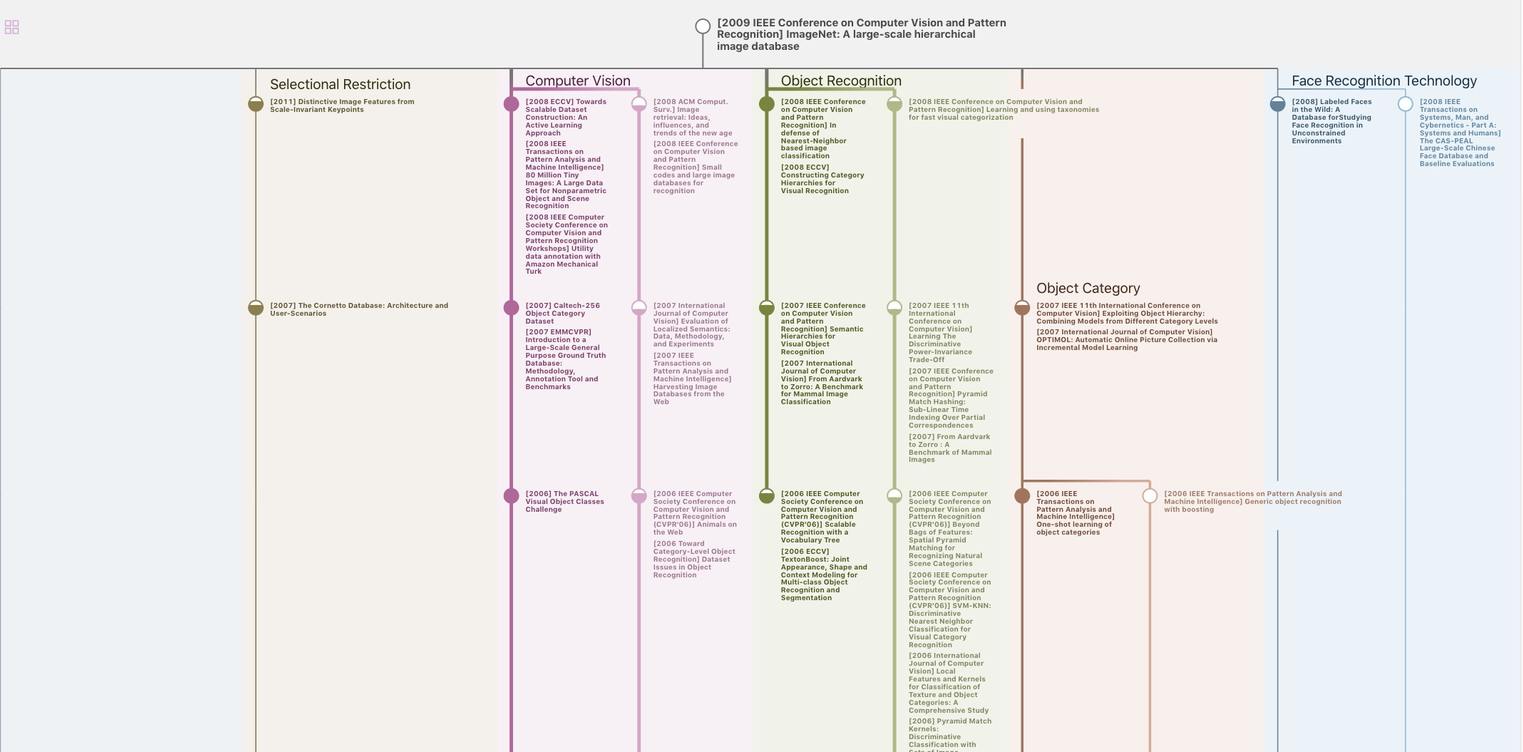
生成溯源树,研究论文发展脉络
Chat Paper
正在生成论文摘要