Lightweight video salient object detection via channel-shuffle enhanced multi-modal fusion network
MULTIMEDIA TOOLS AND APPLICATIONS(2024)
Abstract
Video salient object detection (VSOD) has witnessed great development with the application of deep neural networks. However, the high computational cost of neural networks has hindered the deployment of VSOD models in real-world applications.In this work, we focus on developing lightweight VSOD model. The main issues involved in designing lightweight video saliency models include: how to combine multi-modal information (i.e., spatial and temporal information) and model multi-scale spatial context in an efficient setting. To tackle these issues, we propose a lightweight neural network architecture for VSOD. We start by adopting the ImageNet-pretrained ShuffleNet-V2 for deep feature extraction. Based on the backbone network, a Depth-wise Multi-scale Pooling Module (DMPM) is proposed to aggregate multi-scale spatial context information, which occupies only a small amount of parameters and computational overheads. Most importantly, a Shuffle enhanced Multi-modal Fusion Module (SMFM) is proposed to fuse spatial and temporal information progressively in an efficient manner, deriving the final saliency prediction. With these proposed modules, our method could achieve competitive detection accuracy with current outstanding methods while holding a much smaller model size. Specifically, the proposed model could run at a GPU speed of 49.2 FPS and hold only 1.9M parameters, making it suitable for real-time applications.
MoreTranslated text
Key words
Video salient object detection,Lightweight model,Multi-modal fusion
AI Read Science
Must-Reading Tree
Example
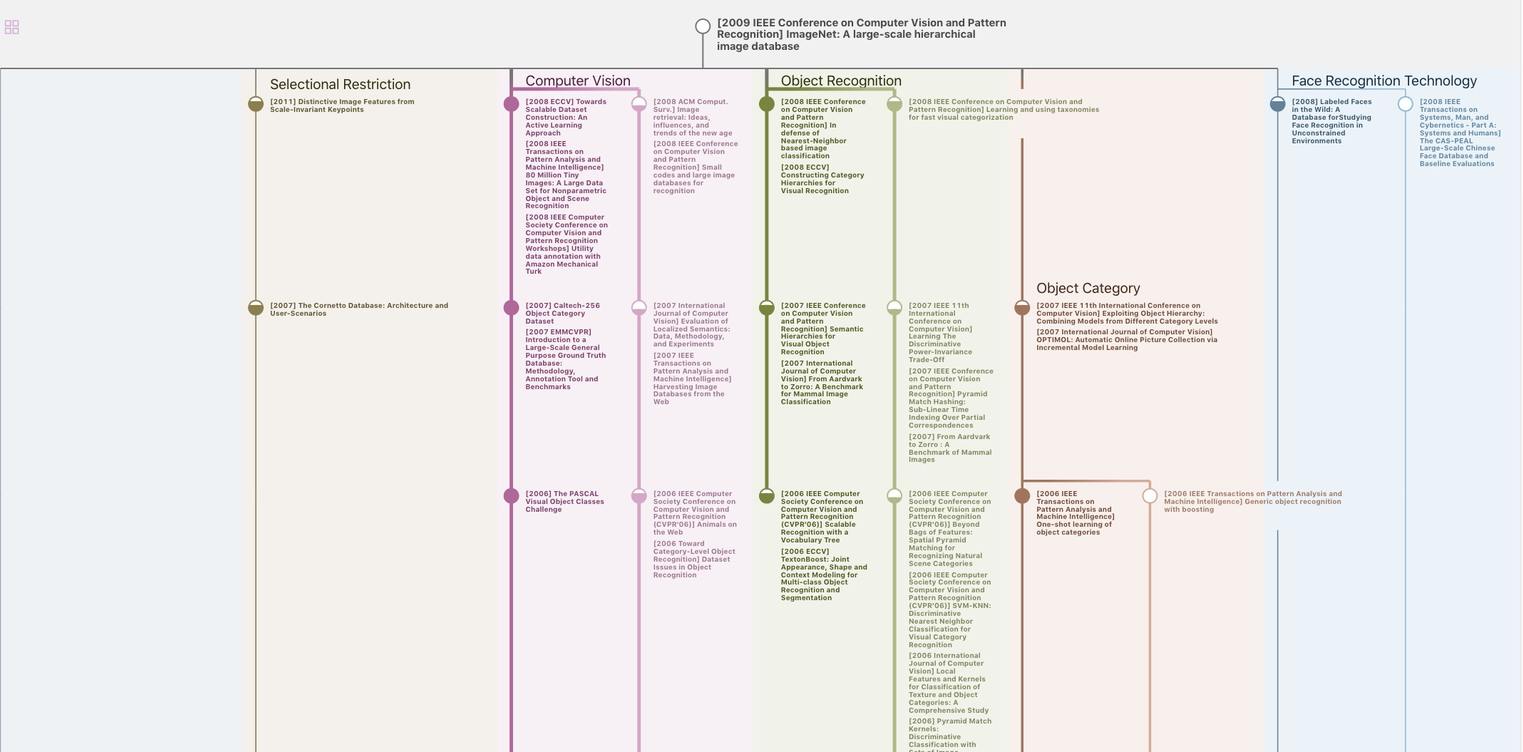
Generate MRT to find the research sequence of this paper
Chat Paper
Summary is being generated by the instructions you defined