Wine component tracing method based on near infrared spectrum fusion machine learning
FRONTIERS IN SUSTAINABLE FOOD SYSTEMS(2023)
摘要
An intelligent wine detection and traceability method based on infrared spec-troscopy and machine learning is proposed, in order to meet the needs of online rapid nondestructive testing of wine. On the basis of extracting infrared spectrum of wine, the principal component analysis (PCA) - support vector machine (SVM) model was modified by chemometrics. A total of 300 grape wine samples were collected from six production areas. The composition of the samples was analyzed by ultra performance liquid chromatography-quadrupole time-of-flight mass spectrometry (UPLC-Q-TOF-MS). According to the experimental results, indole, sulfacetamide and caffeine were selected as characteristics of different origins. Near infrared spectral wavelengths of wine samples were compressed between 900 and 2,500 nm. The ranges of 1,000 nm similar to 1,400 nm and 1,500 nm similar to 1800 nm were selected for PCA principal component analysis and key spectral wavelengths were extracted. The unsupervised learning model of SVM is used to classify and identify key spectral wavelengths. The experimental results show that the algorithm has higher classification accuracy than traditional PCA-LDA, PCA and other algorithms. The classification accuracy of the algorithm is improved from 98.3 to 99.75%. The improved PCA-SVM algorithm can achieve fast and loss-less source tracing of wine.
更多查看译文
关键词
near infrared spectrum,machine learning
AI 理解论文
溯源树
样例
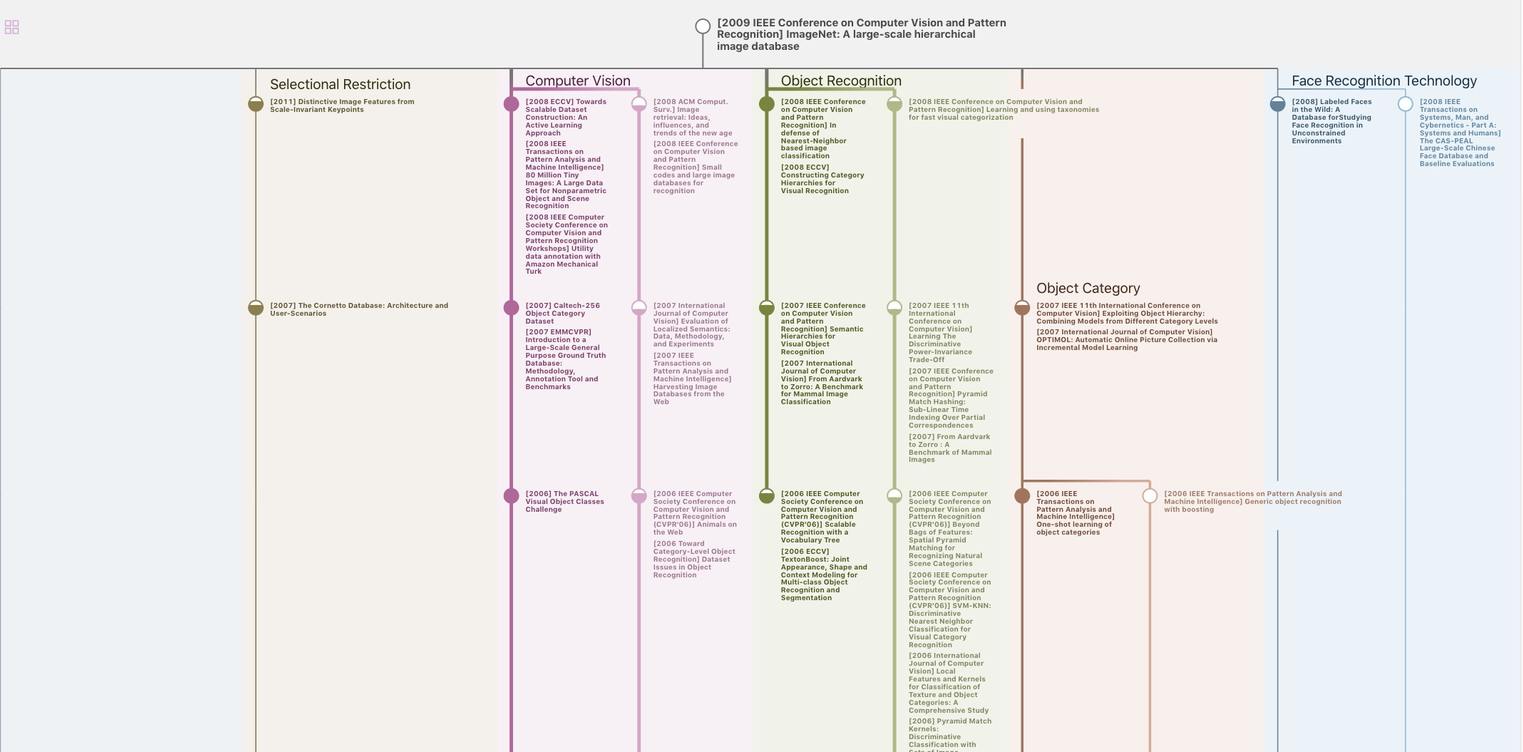
生成溯源树,研究论文发展脉络
Chat Paper
正在生成论文摘要