Optimal Symbolic Entropy: an Adaptive Feature Extraction Algorithm for Condition Monitoring of Bearings
Information fusion(2023)
摘要
Due to their effectiveness in vibration-based fault feature extraction from bearings, entropy-based methods have become a hot research topic. Symbolic dynamic filtering reduces background noise in bearing signals, making it ideal for entropy analysis. However, the partitioning approach selection of symbolic dynamic filtering mainly depends on experience, which may bring over-track and under-track phenomena. The optimal symbolic entropy (OSE) method proposes a solution by using mean spectral kurtosis to evaluate the symbolization performance of partitioning approaches. This method improves the identification of bearing faults through steps such as evaluating frequency and amplitude preservation, selecting the optimal symbolization approach, and using the OSE method with multiscale analysis. Simulative and experimental data analysis demonstrates its superior ability to extract bearing fault characteristics, with better performance and robustness than existing methods.
更多查看译文
关键词
Bearings,Symbolic dynamic filtering,Mean spectral kurtosis,Optimal symbolic entropy,Weak fault feature extraction,Fault diagnosis
AI 理解论文
溯源树
样例
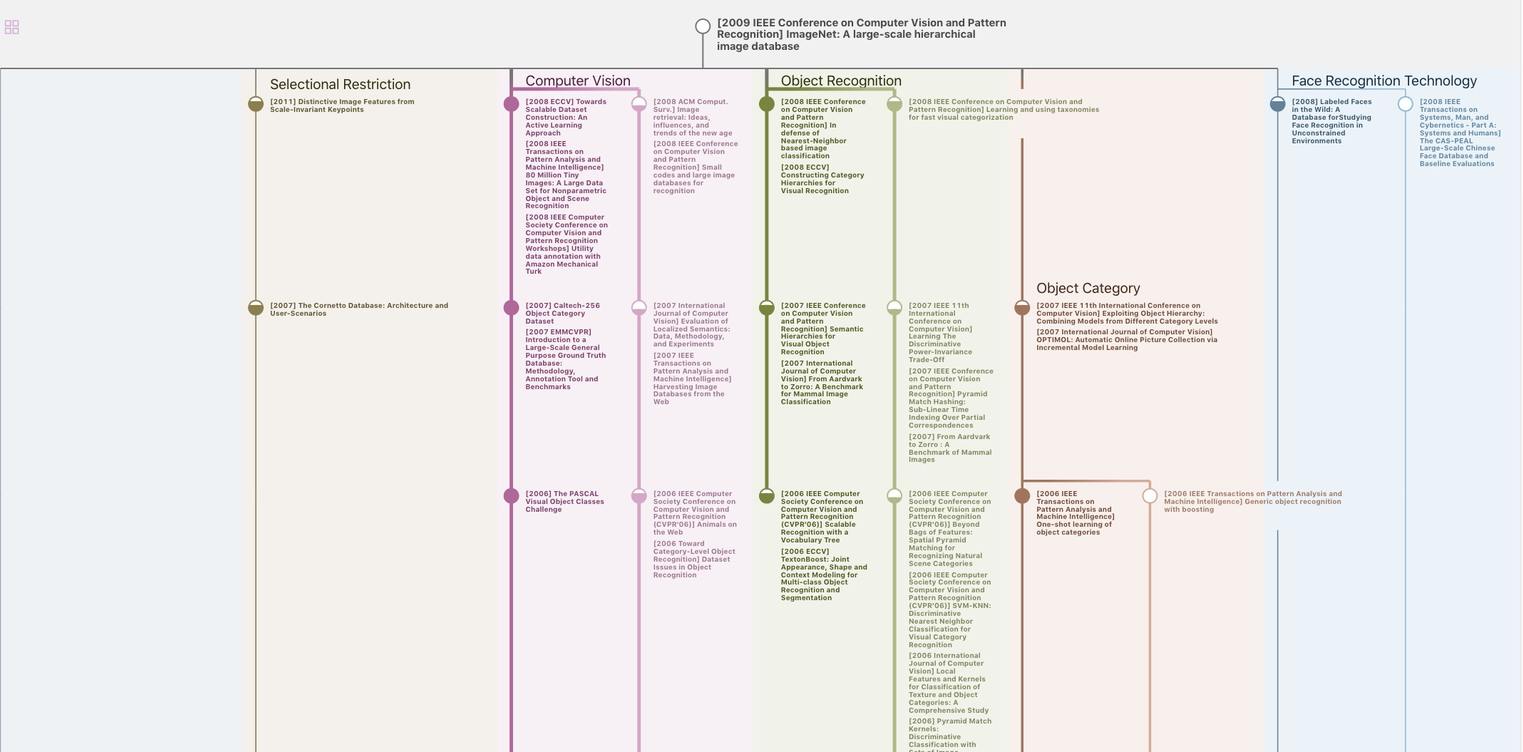
生成溯源树,研究论文发展脉络
Chat Paper
正在生成论文摘要