Manifold-based Denoising, Outlier Detection, and Dimension Reduction Algorithm for High-Dimensional Data
International journal of machine learning and cybernetics(2023)
摘要
Manifold learning, which has emerged in recent years, plays an increasingly important role in machine learning. However, because inevitable noises and outliers destroy the manifold structure of data, the dimensionality reduction effect of manifold learning will be reduced. Therefore, this paper proposes a denoising algorithm for high-dimensional data based on manifold learning. The algorithm first projects noisy sample vectors onto the local manifold, thereby achieving noise reduction. Then, a statistical analysis of noises is performed to obtain a data boundary. Because all the data come from the same background and obey the same distribution, the sample vectors that are not within the data boundary are marked as outliers, and these outliers are eliminated. Finally, the dimension reduction of the data after noise reduction and outlier detection is performed. Experimental results show that the algorithm can effectively eliminate the interference of noises and outliers in high-dimensional datasets to some extent for manifold learning.
更多查看译文
关键词
Noise reduction,Outlier detection,Manifold learning
AI 理解论文
溯源树
样例
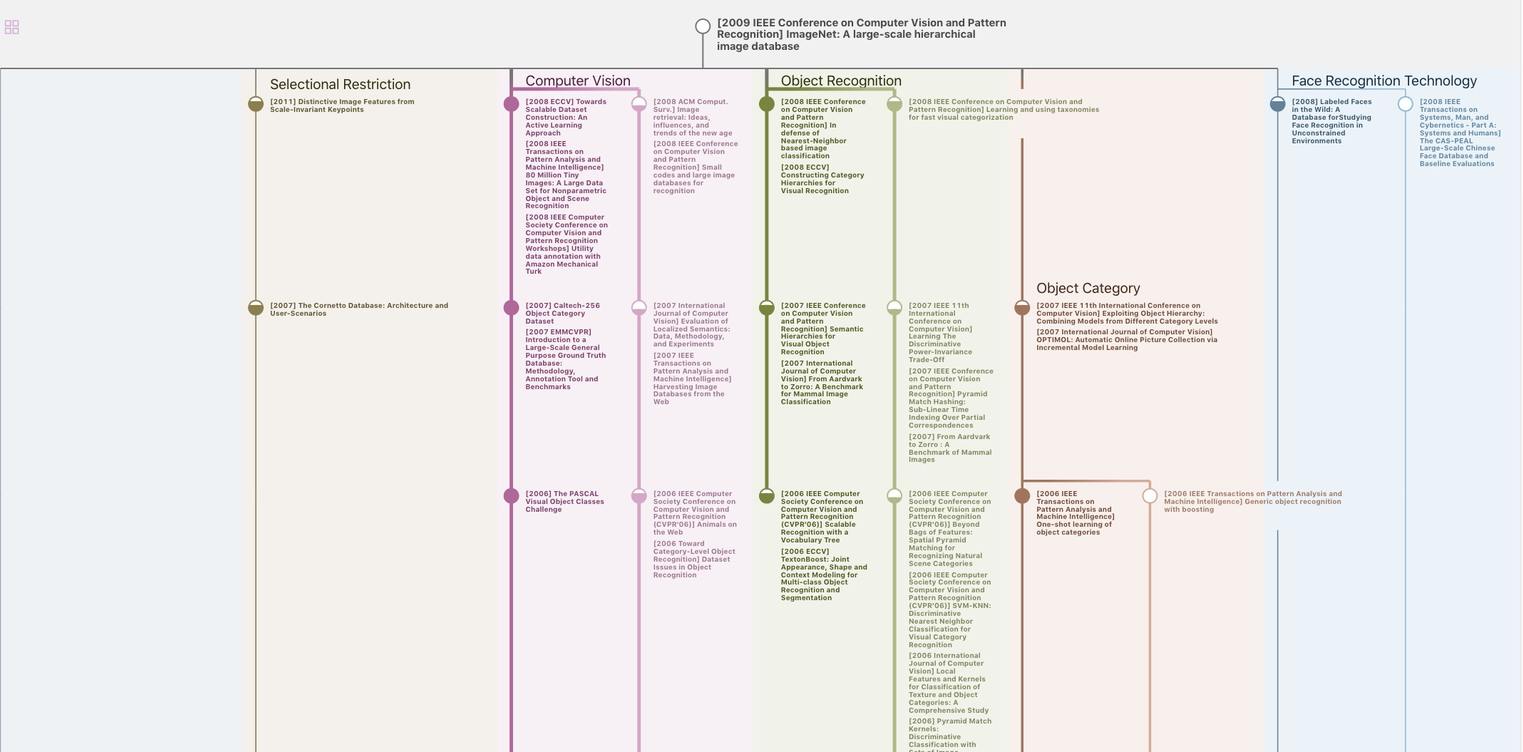
生成溯源树,研究论文发展脉络
Chat Paper
正在生成论文摘要