Multi-granular labels with three-way decisions for multi-label classification
INTERNATIONAL JOURNAL OF MACHINE LEARNING AND CYBERNETICS(2023)
摘要
Multi-label classification is a challenging issue because it simultaneously embraces the characteristics of the imbalanced class distribution for each label and the uncertain label correlation among the whole label space. The decision-theoretic rough set can describe the roughness of concepts in the sense of minimizing decision risk but fails to consider the case where concepts are compatible. We argue that it is feasible to analyze the uncertainty of coarse-grained logical labels with limited label correlation assumptions and reduce the classification error for those uncertain instances by learning fine-grained numerical labels. Consequently, we develop a multi-granular label information system by introducing a multi-granular threshold with a three-way-based label enhancement (MGT-LEML) model. With the second-order label correlation assumption, we deduce the pseudo-positive and pseudo-negative classes for each label. The decision-theoretic rough set evaluates the possibility of misclassification independently, and a novel uncertain measure called instance uncertainty degree determines whether it is necessary to conduct label enhancement afterward. In this way, instances with the most uncertain classifications across label space compute fine-granule numerical labels by label enhancement, whereas remaining unchanged otherwise. We analyze the comparison results among nine algorithms on eight benchmarks with six metrics to demonstrate the superiority of the proposed MGT-LEML algorithm over state-of-the-art multi-label classification algorithms. Compared with the HNOML algorithm, our algorithm achieves significant improvement. Concretely, the performance is reduced by 2.9% in Hamming Loss, 12.4% in Ranking Loss, 14.3% in One Error, 465.5% in Coverage, and is increased by 14.2% in Average Precision.
更多查看译文
关键词
Multi-label classification,Multi-granular,Three-way decisions,Decision-theoretic rough sets,Uncertainty,Label enhancement
AI 理解论文
溯源树
样例
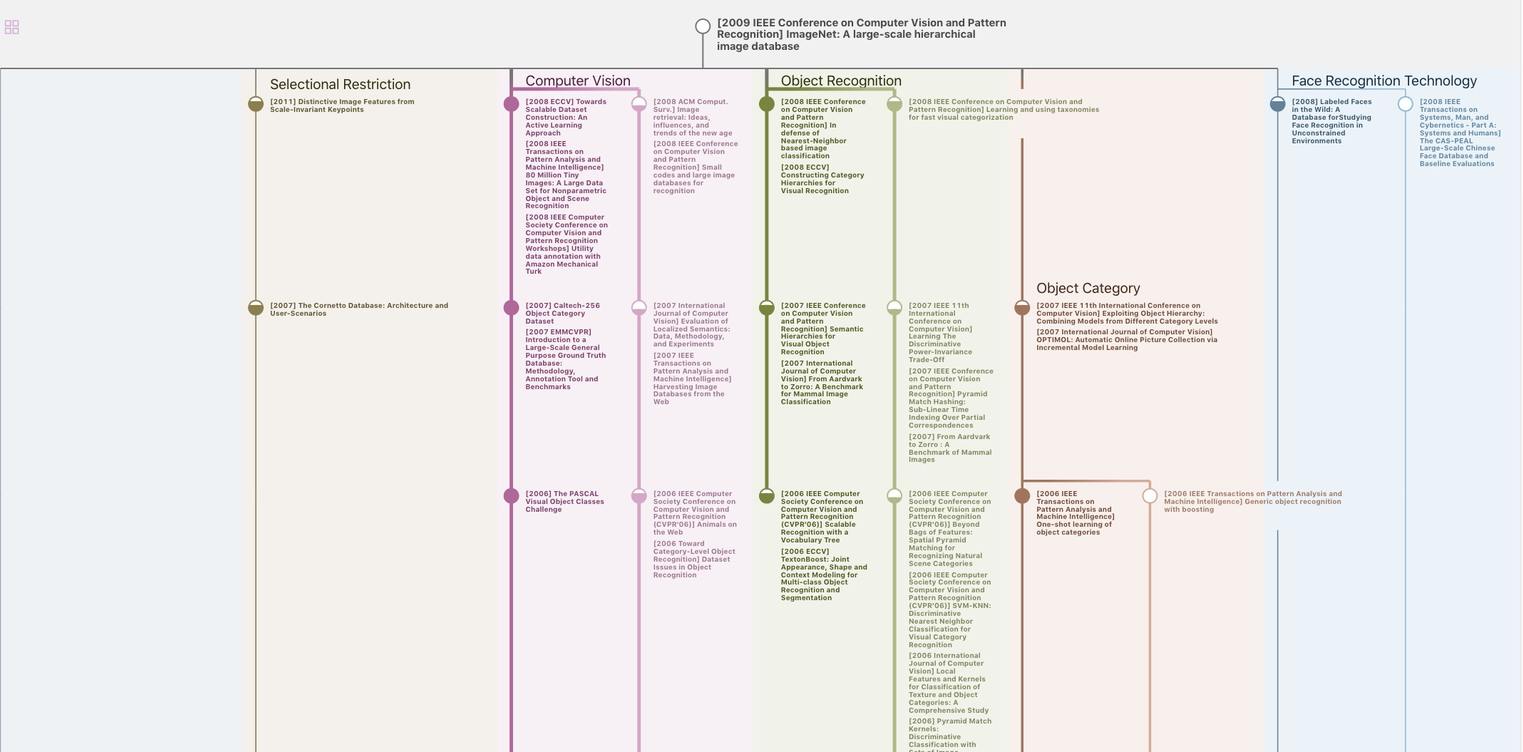
生成溯源树,研究论文发展脉络
Chat Paper
正在生成论文摘要