On efficacy of Meta-Learning for Domain Generalization in Speech Emotion Recognition.
PerCom Workshops(2023)
摘要
Speech Emotion Recognition (SER) refers to the recognition of human emotions from natural speech, vital for building human-centered context-aware intelligent systems. Here, domain shift, where models' trained on one domain exhibit performance degradation when exposed to an unseen domain with different statistics, is a major limiting factor in SER applicability, as models have a strong dependence on speakers and languages characteristics used during training. Meta-Learning for Domain Generalization (MLDG) has shown great success in improving models' generalization capacity and alleviate the domain shift problem in the vision domain; yet, its' efficacy on SER remains largely unexplored. In this work, we propose a “domain-shift aware” MLDG approach to learn generalizable models across multiple domains in SER. Based on our extensive evaluation, we identify a number of pitfalls that contribute to poor models' DG ability, and demonstrate that log-mel spectrograms representations lack distinct features required for MLDG in SER. We further explore the use of appropriate features to achieve DG in SER as to provide insides to future research directions for DG in SER.
更多查看译文
关键词
deep learning,meta-learning,speech emotion recognition,domain generalization,domain shift
AI 理解论文
溯源树
样例
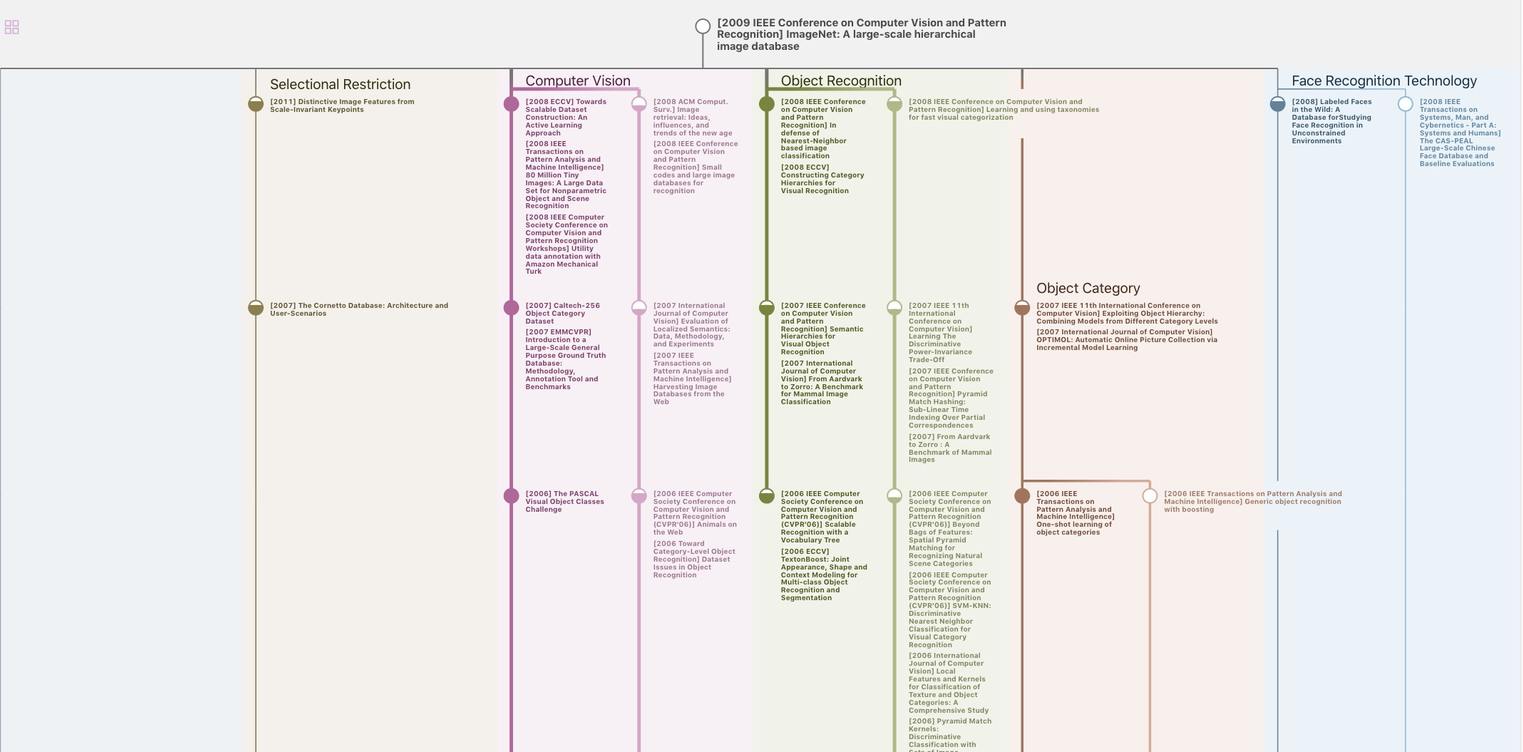
生成溯源树,研究论文发展脉络
Chat Paper
正在生成论文摘要