CrowdFlowTransformer: Capturing Spatio-Temporal Dependence for Forecasting Human Mobility.
PerCom Workshops(2023)
摘要
Crowd flow forecasting is expected to have a wide range of applications such as human resource allocation, guidance design, marketing, disaster mitigation and congestion prediction for avoiding epidemic such as COVID-19. Crowd flow forecasting is challenging because it requires considering both the task of capturing the temporal dependency of data and capturing the spatial dependence. To address these challenges, in this paper, we propose a mechanism for referencing time-series features that are important for forecasting and incorporating graph convolution into Transformer, and we introduce CrowdFlowTransformer(CF-Transformer), a deep learning model based on Google's Transformer framework captures the Spatio-temporal dependency of time series. CF-Transformer captures the time series dependency by extracting important local time series from the past time series, inputting them to the decoder of Transformer, and encoding critical features into the model's input. We adapted CF-Transformer to a real-world crowd flow dataset. We evaluated it by comparing its forecasting accuracy with conventional models, and the results demonstrate that our model outperforms the conventional models.
更多查看译文
关键词
crowd flow,Transformer,graph convolution,time series forecasting,human mobility
AI 理解论文
溯源树
样例
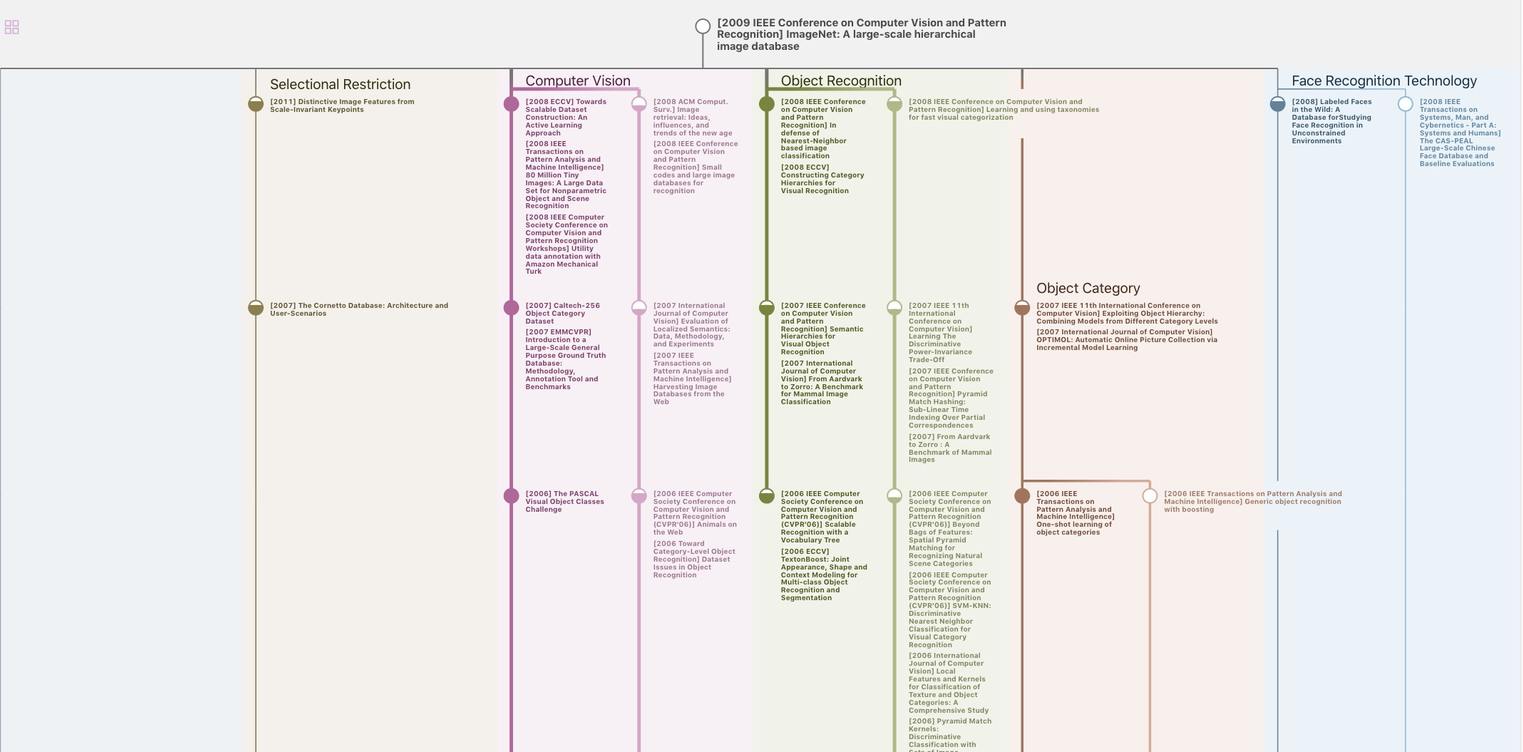
生成溯源树,研究论文发展脉络
Chat Paper
正在生成论文摘要