Leukocyte Detection with Novel Fully Convolutional Network and a New Dataset of Blood Smear Complete Samples
Pattern Recognition(2023)
Abstract
The analysis of leukocytes in blood smear sample images has been a successful tool for medical diagnosis, and there are machine-learning methods for segmenting and classifying leukocytes with these images. However, the datasets for designing these methods have images with different compositions than blood smear samples acquired with the standard protocols used in real clinical laboratories. Then, to contribute to the effort to improve the research related to the analysis of leukocytes, this paper presents a new dataset and a method for Leukocyte detection named Color Normalized UNet (CUNet). LeukoSet comprises 1497 images of blood smear complete samples using a protocol developed in a real clinical laboratory environment. The dataset has ground truths that classify the leukocytes into Lymphocytes, Monocytes, Neutrophils, Basophils, Eosinophils, and deformed leukocytes. CUNet is a Fully Convolutional Network (FCN) based on UNet for leukocyte detection designed with the images of LeukoSet. Experiments report that CUNet has an accuracy of 94.22% and an inference time of 27 ms. The results of CUNet are better than other leukocyte detection methods implemented in the literature.
MoreTranslated text
Key words
novel fully convolutional network,blood,smear
AI Read Science
Must-Reading Tree
Example
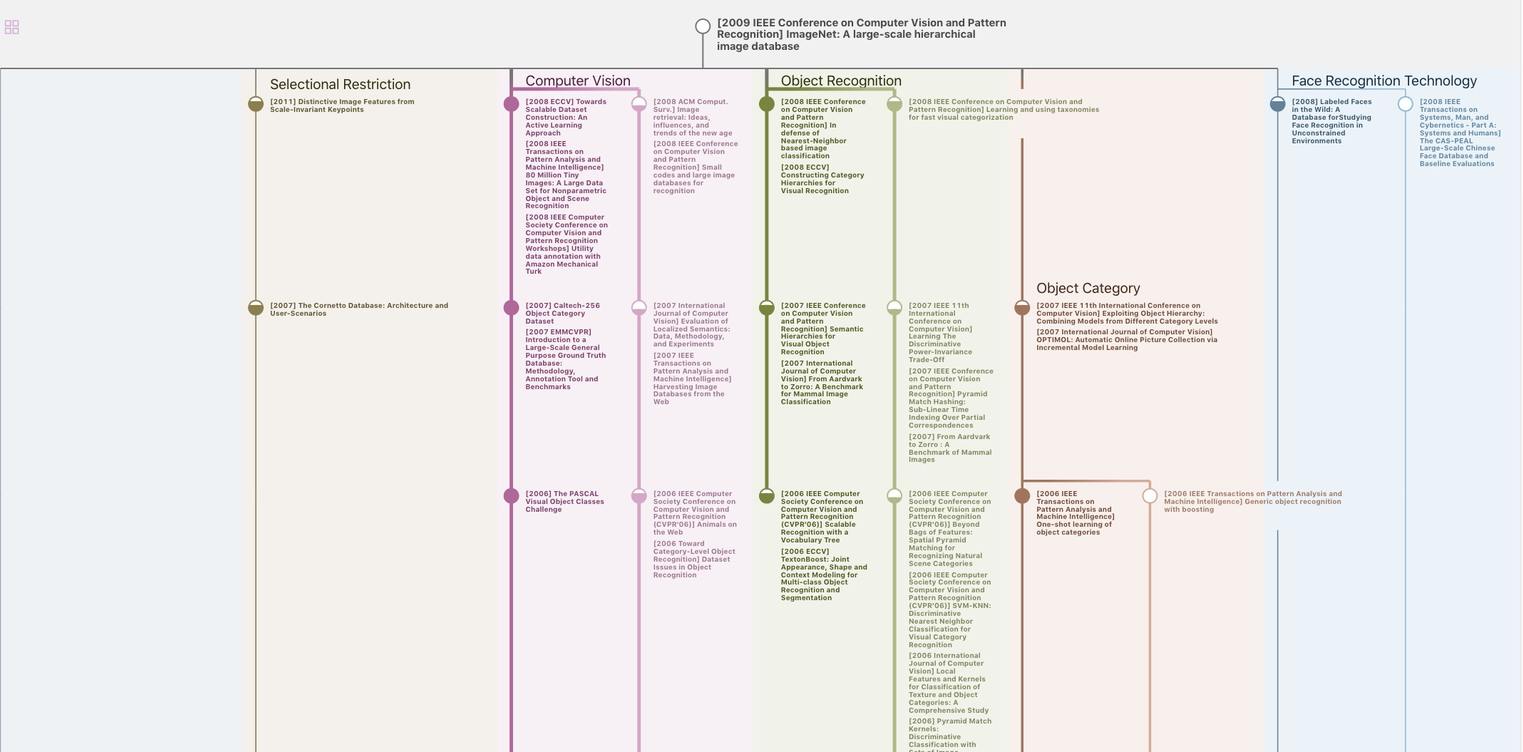
Generate MRT to find the research sequence of this paper
Chat Paper
Summary is being generated by the instructions you defined