Impact of Clinical-Text-Based Fall Prediction Model on Preventing Extended Hospital Stays for Elderly Inpatients (Preprint)
crossref(2022)
Abstract
BACKGROUND Falls may cause elderly people to be bedridden, requiring professional intervention; thus, prevention is crucial. The use of electronic health records (EHRs) is expected to provide highly accurate risk assessment and length-of-stay data related to falls, which may be used to estimate the costs and benefits of prevention. However, no studies exist that investigate the extent to which hospital stays could be shortened through fall avoidance resulting from the use of prediction tools. OBJECTIVE First, the extended length of hospital stays caused by falls among elderly inpatients was estimated. Next, we developed a model that predicts falls by using clinical text as input and evaluated its accuracy. Finally, we estimated the potentially shortened hospital stay that would be made possible by appropriate interventions based on the prediction model. METHODS Patients 65 years or older were selected as subjects, and the EHRs of 1,728 falls and 70,586 non-falls were subjected to analysis. The extended stay lengths were estimated using the propensity score-matching of 49 associated variables. Bidirectional encoder representations from transformers and bidirectional Long Short-Term Memory (LSTM) methods were used to predict falls from clinical text. The estimated length of stay and the outputs of the prediction model were used to determine stay reductions. RESULTS The extended length of hospital stay due to falls was estimated to be 17.8 days (95% CI: 16.6–19.0), and it fell to 8.6 days when there were unobserved covariants at an odds ratio of 2.0. The accuracy of the prediction model was as follows: area under the receiver operating characteristic curve, 0.851; F-value, 0.165; recall, 0.737; precision, 0.093; and specificity, 0.839. When assuming interventions with 25 or 100% effectiveness against cases where the model predicted a fall, the stay reduction was estimated at 0.038 and 0.154 days/day, respectively. CONCLUSIONS The accuracy of the prediction model using clinical text is thought to be higher than the prediction accuracy of conventional assessments. However, our model’s precision remained low at 9.3%. This may be due, in part, to the inclusion of cases in which falls did not occur because of preventative interventions during hospitalization. Nonetheless, it is estimated that interventions for cases when falls were predicted will reduce medical costs by 1,520 yen/day of intervention, even if the preventative effect is 25%. Limitations include the fact that these results cannot be extrapolated to short- or long-term hospitalization cases, and that this was a single-center study.
MoreTranslated text
AI Read Science
Must-Reading Tree
Example
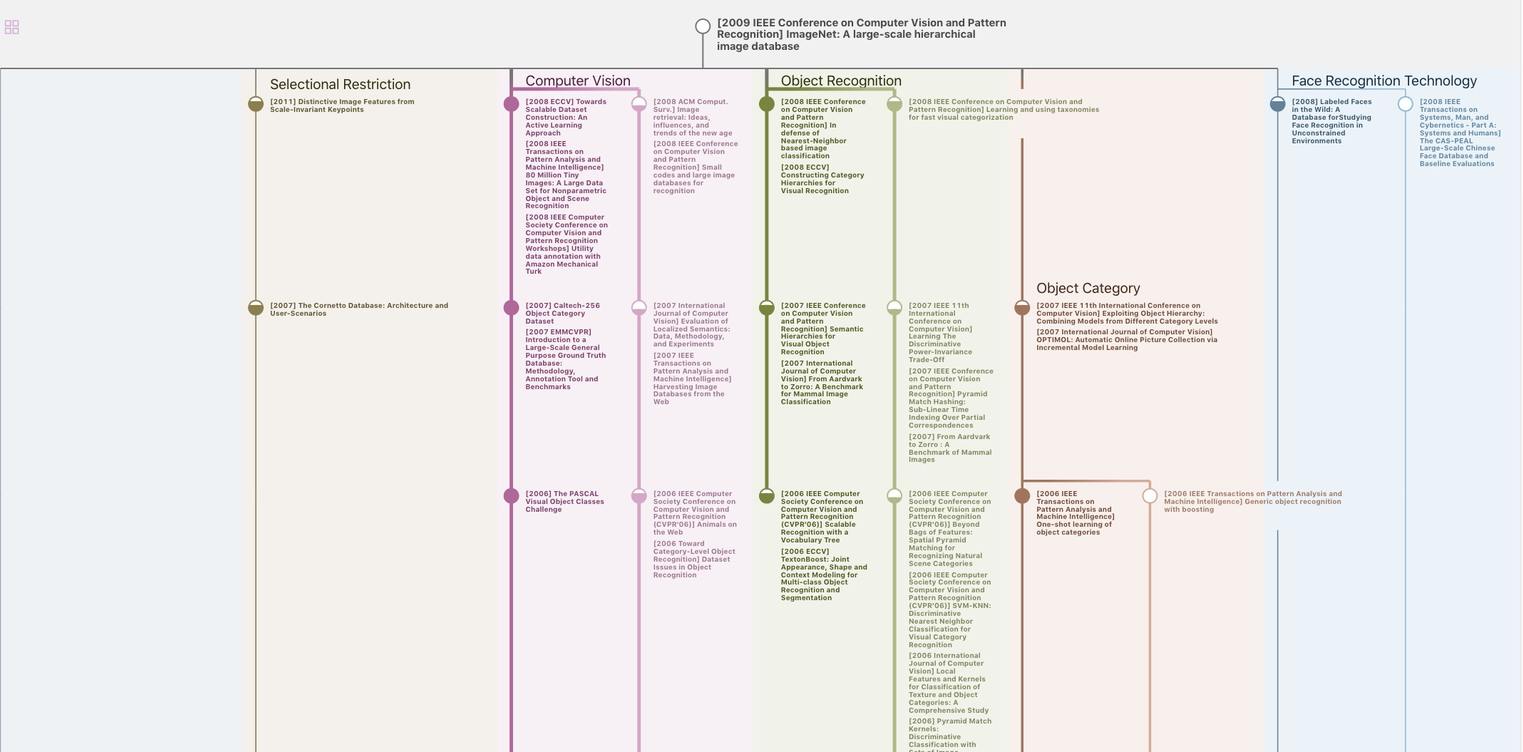
Generate MRT to find the research sequence of this paper
Chat Paper
Summary is being generated by the instructions you defined